5
1
9783642107474
Analysing Seasonal Health Data / Edition 1 available in Hardcover, Paperback
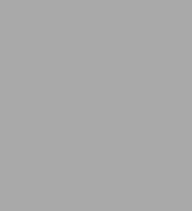
Analysing Seasonal Health Data / Edition 1
- ISBN-10:
- 3642107478
- ISBN-13:
- 9783642107474
- Pub. Date:
- 02/12/2010
- Publisher:
- Springer Berlin Heidelberg
- ISBN-10:
- 3642107478
- ISBN-13:
- 9783642107474
- Pub. Date:
- 02/12/2010
- Publisher:
- Springer Berlin Heidelberg
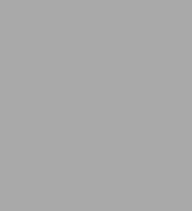
Analysing Seasonal Health Data / Edition 1
$109.99
Current price is , Original price is $109.99. You
109.99
In Stock
Product Details
ISBN-13: | 9783642107474 |
---|---|
Publisher: | Springer Berlin Heidelberg |
Publication date: | 02/12/2010 |
Series: | Statistics for Biology and Health |
Edition description: | 2010 |
Pages: | 164 |
Product dimensions: | 6.40(w) x 9.30(h) x 0.70(d) |
About the Author
From the B&N Reads Blog