Deep Learning for Image/Video Restoration and Super-Resolution
In this monograph, an overview of recent developments and the state-of-the-art in image/video restoration and super-resolution (SR) using deep learning is presented. Deep learning has made a significant impact, not only on computer vision and natural language processing but also on classical signal processing problems such as image/video restoration/SR and compression. Recent advances in neural architectures led to significant improvements in the performance of learned image/video restoration and SR. An important benefit of data-driven deep learning approaches is that neural models can be optimized for any differentiable loss function, including visual perceptual loss functions, leading to perceptual video restoration and SR, which cannot be easily handled by traditional model-based approaches.
The publication starts with a problem statement and a short discussion on traditional vs. data-driven solutions. Thereafter, recent advances in neural architectures are considered, and the loss functions and evaluation criteria for image/video restoration and SR are discussed. Also considered are the learned image restoration and SR, as learning either a mapping from the space of degraded images to ideal images based on the universal approximation theorem, or a generative model that captures the probability distribution of ideal images. Practical problems in applying supervised training to real-life restoration and SR are also included, as well as the solution models.
In the section on learned video SR, approaches to exploit temporal correlations in learned video processing are covered, and then the perceptual optimization of the network parameters to obtain natural texture and motion is discussed. A comparative discussion of various approaches concludes the publication.
1141451174
The publication starts with a problem statement and a short discussion on traditional vs. data-driven solutions. Thereafter, recent advances in neural architectures are considered, and the loss functions and evaluation criteria for image/video restoration and SR are discussed. Also considered are the learned image restoration and SR, as learning either a mapping from the space of degraded images to ideal images based on the universal approximation theorem, or a generative model that captures the probability distribution of ideal images. Practical problems in applying supervised training to real-life restoration and SR are also included, as well as the solution models.
In the section on learned video SR, approaches to exploit temporal correlations in learned video processing are covered, and then the perceptual optimization of the network parameters to obtain natural texture and motion is discussed. A comparative discussion of various approaches concludes the publication.
Deep Learning for Image/Video Restoration and Super-Resolution
In this monograph, an overview of recent developments and the state-of-the-art in image/video restoration and super-resolution (SR) using deep learning is presented. Deep learning has made a significant impact, not only on computer vision and natural language processing but also on classical signal processing problems such as image/video restoration/SR and compression. Recent advances in neural architectures led to significant improvements in the performance of learned image/video restoration and SR. An important benefit of data-driven deep learning approaches is that neural models can be optimized for any differentiable loss function, including visual perceptual loss functions, leading to perceptual video restoration and SR, which cannot be easily handled by traditional model-based approaches.
The publication starts with a problem statement and a short discussion on traditional vs. data-driven solutions. Thereafter, recent advances in neural architectures are considered, and the loss functions and evaluation criteria for image/video restoration and SR are discussed. Also considered are the learned image restoration and SR, as learning either a mapping from the space of degraded images to ideal images based on the universal approximation theorem, or a generative model that captures the probability distribution of ideal images. Practical problems in applying supervised training to real-life restoration and SR are also included, as well as the solution models.
In the section on learned video SR, approaches to exploit temporal correlations in learned video processing are covered, and then the perceptual optimization of the network parameters to obtain natural texture and motion is discussed. A comparative discussion of various approaches concludes the publication.
The publication starts with a problem statement and a short discussion on traditional vs. data-driven solutions. Thereafter, recent advances in neural architectures are considered, and the loss functions and evaluation criteria for image/video restoration and SR are discussed. Also considered are the learned image restoration and SR, as learning either a mapping from the space of degraded images to ideal images based on the universal approximation theorem, or a generative model that captures the probability distribution of ideal images. Practical problems in applying supervised training to real-life restoration and SR are also included, as well as the solution models.
In the section on learned video SR, approaches to exploit temporal correlations in learned video processing are covered, and then the perceptual optimization of the network parameters to obtain natural texture and motion is discussed. A comparative discussion of various approaches concludes the publication.
85.0
In Stock
5
1
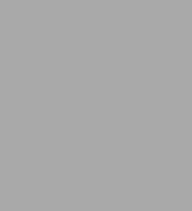
Deep Learning for Image/Video Restoration and Super-Resolution
124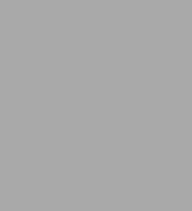
Deep Learning for Image/Video Restoration and Super-Resolution
124Paperback
$85.00
85.0
In Stock
Product Details
ISBN-13: | 9781680839722 |
---|---|
Publisher: | Now Publishers |
Publication date: | 05/05/2022 |
Series: | Foundations and Trends in Computer Graphics and Vision , #36 |
Pages: | 124 |
Product dimensions: | 6.14(w) x 9.21(h) x 0.27(d) |
From the B&N Reads Blog