In this concise book, Eric Eager and Richard Erickson provide a clear introduction to using statistical models to analyze football data using both Python and R. Whether your goal is to qualify for an entry-level football analyst position, dominate your fantasy football league, or simply learn R and Python with fun example cases, this book is your starting place.
Through case studies in both Python and R, you'll learn to:
- Obtain NFL data from Python and R packages and web scraping
- Visualize and explore data
- Apply regression models to play-by-play data
- Extend regression models to classification problems in football
- Apply data science to sports betting with individual player props
- Understand player athletic attributes using multivariate statistics
In this concise book, Eric Eager and Richard Erickson provide a clear introduction to using statistical models to analyze football data using both Python and R. Whether your goal is to qualify for an entry-level football analyst position, dominate your fantasy football league, or simply learn R and Python with fun example cases, this book is your starting place.
Through case studies in both Python and R, you'll learn to:
- Obtain NFL data from Python and R packages and web scraping
- Visualize and explore data
- Apply regression models to play-by-play data
- Extend regression models to classification problems in football
- Apply data science to sports betting with individual player props
- Understand player athletic attributes using multivariate statistics
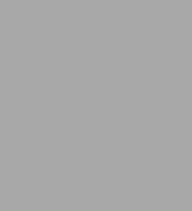
Football Analytics with Python & R: Learning Data Science Through the Lens of Sports
349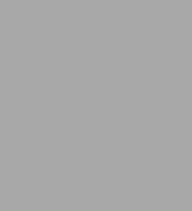
Football Analytics with Python & R: Learning Data Science Through the Lens of Sports
349Product Details
ISBN-13: | 9781492099628 |
---|---|
Publisher: | O'Reilly Media, Incorporated |
Publication date: | 09/19/2023 |
Pages: | 349 |
Product dimensions: | 7.00(w) x 9.19(h) x (d) |