Fuzzy rule systems have found a wide range of applications in many fields of science and technology. Traditionally, fuzzy rules are generated from human expert knowledge or human heuristics for relatively simple systems. In the last few years, data-driven fuzzy rule generation has been very active. Compared to heuristic fuzzy rules, fuzzy rules generated from data are able to extract more profound knowledge for more complex systems. This book presents a number of approaches to the generation of fuzzy rules from data, ranging from the direct fuzzy inference based to neural net works and evolutionary algorithms based fuzzy rule generation. Besides the approximation accuracy, special attention has been paid to the interpretabil ity of the extracted fuzzy rules. In other words, the fuzzy rules generated from data are supposed to be as comprehensible to human beings as those generated from human heuristics. To this end, many aspects of interpretabil ity of fuzzy systems have been discussed, which must be taken into account in the data-driven fuzzy rule generation. In this way, fuzzy rules generated from data are intelligible to human users and therefore, knowledge about unknown systems can be extracted.
1101367866
Advanced Fuzzy Systems Design and Applications
Fuzzy rule systems have found a wide range of applications in many fields of science and technology. Traditionally, fuzzy rules are generated from human expert knowledge or human heuristics for relatively simple systems. In the last few years, data-driven fuzzy rule generation has been very active. Compared to heuristic fuzzy rules, fuzzy rules generated from data are able to extract more profound knowledge for more complex systems. This book presents a number of approaches to the generation of fuzzy rules from data, ranging from the direct fuzzy inference based to neural net works and evolutionary algorithms based fuzzy rule generation. Besides the approximation accuracy, special attention has been paid to the interpretabil ity of the extracted fuzzy rules. In other words, the fuzzy rules generated from data are supposed to be as comprehensible to human beings as those generated from human heuristics. To this end, many aspects of interpretabil ity of fuzzy systems have been discussed, which must be taken into account in the data-driven fuzzy rule generation. In this way, fuzzy rules generated from data are intelligible to human users and therefore, knowledge about unknown systems can be extracted.
109.99
In Stock
5
1
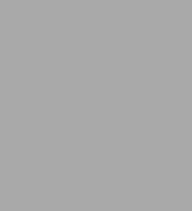
Advanced Fuzzy Systems Design and Applications
272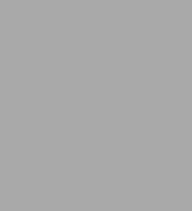
Advanced Fuzzy Systems Design and Applications
272Paperback(Softcover reprint of the original 1st ed. 2003)
$109.99
109.99
In Stock
Product Details
ISBN-13: | 9783790825206 |
---|---|
Publisher: | Physica-Verlag HD |
Publication date: | 12/12/2011 |
Series: | Studies in Fuzziness and Soft Computing , #112 |
Edition description: | Softcover reprint of the original 1st ed. 2003 |
Pages: | 272 |
Product dimensions: | 6.10(w) x 9.25(h) x 0.02(d) |
From the B&N Reads Blog