Algorithms for Minimization Without Derivatives
This outstanding text for graduate students and researchers proposes improvements to existing algorithms, extends their related mathematical theories, and offers details on new algorithms for approximating local and global minima. None of the algorithms requires an evaluation of derivatives; all depend entirely on sequential function evaluation, a highly practical scenario in the frequent event of difficult-to-evaluate derivatives.
Topics include the use of successive interpolation for finding simple zeros of a function and its derivatives; an algorithm with guaranteed convergence for finding a minimum of a function of one variation; global minimization given an upper bound on the second derivative; and a new algorithm for minimizing a function of several variables without calculating derivatives. Many numerical examples augment the text, along with a complete analysis of rate of convergence for most algorithms and error bounds that allow for the effect of rounding errors.
1004816294
This outstanding text for graduate students and researchers proposes improvements to existing algorithms, extends their related mathematical theories, and offers details on new algorithms for approximating local and global minima. None of the algorithms requires an evaluation of derivatives; all depend entirely on sequential function evaluation, a highly practical scenario in the frequent event of difficult-to-evaluate derivatives.
Topics include the use of successive interpolation for finding simple zeros of a function and its derivatives; an algorithm with guaranteed convergence for finding a minimum of a function of one variation; global minimization given an upper bound on the second derivative; and a new algorithm for minimizing a function of several variables without calculating derivatives. Many numerical examples augment the text, along with a complete analysis of rate of convergence for most algorithms and error bounds that allow for the effect of rounding errors.
Algorithms for Minimization Without Derivatives
This outstanding text for graduate students and researchers proposes improvements to existing algorithms, extends their related mathematical theories, and offers details on new algorithms for approximating local and global minima. None of the algorithms requires an evaluation of derivatives; all depend entirely on sequential function evaluation, a highly practical scenario in the frequent event of difficult-to-evaluate derivatives.
Topics include the use of successive interpolation for finding simple zeros of a function and its derivatives; an algorithm with guaranteed convergence for finding a minimum of a function of one variation; global minimization given an upper bound on the second derivative; and a new algorithm for minimizing a function of several variables without calculating derivatives. Many numerical examples augment the text, along with a complete analysis of rate of convergence for most algorithms and error bounds that allow for the effect of rounding errors.
This outstanding text for graduate students and researchers proposes improvements to existing algorithms, extends their related mathematical theories, and offers details on new algorithms for approximating local and global minima. None of the algorithms requires an evaluation of derivatives; all depend entirely on sequential function evaluation, a highly practical scenario in the frequent event of difficult-to-evaluate derivatives.
Topics include the use of successive interpolation for finding simple zeros of a function and its derivatives; an algorithm with guaranteed convergence for finding a minimum of a function of one variation; global minimization given an upper bound on the second derivative; and a new algorithm for minimizing a function of several variables without calculating derivatives. Many numerical examples augment the text, along with a complete analysis of rate of convergence for most algorithms and error bounds that allow for the effect of rounding errors.
14.95
In Stock
5
1
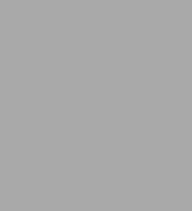
Algorithms for Minimization Without Derivatives
206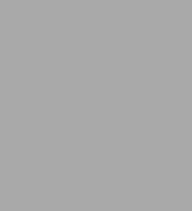
Algorithms for Minimization Without Derivatives
206
14.95
In Stock
Product Details
ISBN-13: | 9780486419985 |
---|---|
Publisher: | Dover Publications |
Publication date: | 04/17/2013 |
Series: | Dover Books on Mathematics Series |
Pages: | 206 |
Product dimensions: | 5.30(w) x 8.40(h) x 0.60(d) |
From the B&N Reads Blog