Applied Spatial Statistics for Public Health Data
While mapped data provide a common ground for discussions between the public, the media, regulatory agencies, and public health researchers, the analysis of spatially referenced data has experienced a phenomenal growth over the last two decades, thanks in part to the development of geographical information systems (GISs). This is the first thorough overview to integrate spatial statistics with data management and the display capabilities of GIS. It describes methods for assessing the likelihood of observed patterns and quantifying the link between exposures and outcomes in spatially correlated data.
This introductory text is designed to serve as both an introduction for the novice and a reference for practitioners in the field
Requires only minimal background in public health and only some knowledge of statistics through multiple regression
Touches upon some advanced topics, such as random effects, hierarchical models and spatial point processes, but does not require prior exposure
Includes lavish use of figures/illustrations throughout the volume as well as analyses of several data sets (in the form of "data breaks")
Exercises based on data analyses reinforce concepts
1116661452
This introductory text is designed to serve as both an introduction for the novice and a reference for practitioners in the field
Requires only minimal background in public health and only some knowledge of statistics through multiple regression
Touches upon some advanced topics, such as random effects, hierarchical models and spatial point processes, but does not require prior exposure
Includes lavish use of figures/illustrations throughout the volume as well as analyses of several data sets (in the form of "data breaks")
Exercises based on data analyses reinforce concepts
Applied Spatial Statistics for Public Health Data
While mapped data provide a common ground for discussions between the public, the media, regulatory agencies, and public health researchers, the analysis of spatially referenced data has experienced a phenomenal growth over the last two decades, thanks in part to the development of geographical information systems (GISs). This is the first thorough overview to integrate spatial statistics with data management and the display capabilities of GIS. It describes methods for assessing the likelihood of observed patterns and quantifying the link between exposures and outcomes in spatially correlated data.
This introductory text is designed to serve as both an introduction for the novice and a reference for practitioners in the field
Requires only minimal background in public health and only some knowledge of statistics through multiple regression
Touches upon some advanced topics, such as random effects, hierarchical models and spatial point processes, but does not require prior exposure
Includes lavish use of figures/illustrations throughout the volume as well as analyses of several data sets (in the form of "data breaks")
Exercises based on data analyses reinforce concepts
This introductory text is designed to serve as both an introduction for the novice and a reference for practitioners in the field
Requires only minimal background in public health and only some knowledge of statistics through multiple regression
Touches upon some advanced topics, such as random effects, hierarchical models and spatial point processes, but does not require prior exposure
Includes lavish use of figures/illustrations throughout the volume as well as analyses of several data sets (in the form of "data breaks")
Exercises based on data analyses reinforce concepts
194.95
In Stock
5
1
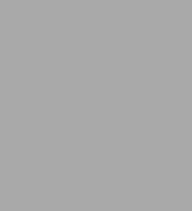
Applied Spatial Statistics for Public Health Data
520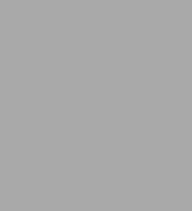
Applied Spatial Statistics for Public Health Data
520Hardcover
$194.95
194.95
In Stock
Product Details
ISBN-13: | 9780471387718 |
---|---|
Publisher: | Wiley |
Publication date: | 07/15/2004 |
Series: | Wiley Series in Probability and Statistics , #368 |
Pages: | 520 |
Product dimensions: | 6.50(w) x 9.60(h) x 1.32(d) |
About the Author
What People are Saying About This
From the B&N Reads Blog