What is bad data? Some people consider it a technical phenomenon, like missing values or malformed records, but bad data includes a lot more. In this handbook, data expert Q. Ethan McCallum has gathered 19 colleagues from every corner of the data arena to reveal how they’ve recovered from nasty data problems.
From cranky storage to poor representation to misguided policy, there are many paths to bad data. Bottom line? Bad data is data that gets in the way. This book explains effective ways to get around it.
Among the many topics covered, you’ll discover how to:
- Test drive your data to see if it’s ready for analysis
- Work spreadsheet data into a usable form
- Handle encoding problems that lurk in text data
- Develop a successful web-scraping effort
- Use NLP tools to reveal the real sentiment of online reviews
- Address cloud computing issues that can impact your analysis effort
- Avoid policies that create data analysis roadblocks
- Take a systematic approach to data quality analysis
What is bad data? Some people consider it a technical phenomenon, like missing values or malformed records, but bad data includes a lot more. In this handbook, data expert Q. Ethan McCallum has gathered 19 colleagues from every corner of the data arena to reveal how they’ve recovered from nasty data problems.
From cranky storage to poor representation to misguided policy, there are many paths to bad data. Bottom line? Bad data is data that gets in the way. This book explains effective ways to get around it.
Among the many topics covered, you’ll discover how to:
- Test drive your data to see if it’s ready for analysis
- Work spreadsheet data into a usable form
- Handle encoding problems that lurk in text data
- Develop a successful web-scraping effort
- Use NLP tools to reveal the real sentiment of online reviews
- Address cloud computing issues that can impact your analysis effort
- Avoid policies that create data analysis roadblocks
- Take a systematic approach to data quality analysis
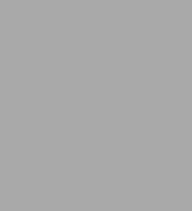
Bad Data Handbook: Cleaning Up The Data So You Can Get Back To Work
264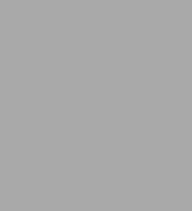
Bad Data Handbook: Cleaning Up The Data So You Can Get Back To Work
264Related collections and offers
Product Details
ISBN-13: | 9781449324971 |
---|---|
Publisher: | O'Reilly Media, Incorporated |
Publication date: | 11/07/2012 |
Sold by: | Barnes & Noble |
Format: | eBook |
Pages: | 264 |
File size: | 5 MB |