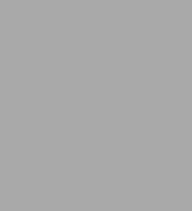
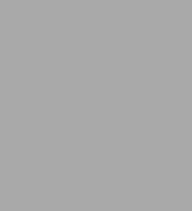
Paperback(New Edition)
-
SHIP THIS ITEMIn stock. Ships in 1-2 days.PICK UP IN STORE
Your local store may have stock of this item.
Available within 2 business hours
Related collections and offers
Overview
Product Details
ISBN-13: | 9780521615594 |
---|---|
Publisher: | Cambridge University Press |
Publication date: | 05/10/2007 |
Edition description: | New Edition |
Pages: | 312 |
Product dimensions: | 5.91(w) x 8.90(h) x 0.71(d) |
About the Author
Read an Excerpt
Cambridge University Press
978-0-521-85057-5 - Bayesian Methods for Ecology - by Michael A. McCarthy
Excerpt
1
Introduction
There is a revolution brewing in ecology. Granted, it is a gentle and slow revolution, but there is growing dissatisfaction with the statistical methods that have been most commonly taught and used in ecology (Hilborn and Mangel, 1997; Wade, 2000; Clark, 2005).1 One aspect of this revolution is the increasing interest in Bayesian statistics (Fig. 1.1). This book aims to foster the revolution by making Bayesian statistics more accessible to every ecologist.
Ecology is the scientific study of the distribution and abundance of biological organisms, and how their interactions with each other and the environment influence their distribution and abundance (Begon et al., 2005). The discipline depends on the measurement of variables and analysis of relationships between them. Because of the size and complexity of ecological systems, ecological data are almost invariably subject to error. Ecologists use statistical methods to distinguish true responses from error. Statistical methods make the interpretation of data transparent and repeatable, so they play an extremely important role in ecology.
The Bayesian approach is one of a number of ways in which ecologists use data to make inferences about nature. The different approaches are underpinned by fundamentally different philosophies and logic. The appropriateness of different statistical approaches has been fiercelydebated in numerous disciplines but ecologists are only now becoming aware of this controversy. This occurs at least in part because the majority of statistical books read by ecologists propound conventional
Image not available in HTML version |
statistics, ignore criticisms of these methods and do not acknowledge that there are alternatives (Fowler et al., 1998; Sokal and Rohlf, 1995; Underwood, 1997; Zar, 1999). Those that do address the controversy usually aim to change the status quo (Hilborn and Mangel, 1997; Burnham and Anderson, 2002), although there are exceptions (Quinn and Keough, 2002; Gotelli and Ellison, 2004).
The Bayesian approach is used relatively rarely (Fig. 1.1), so why should it interest ecologists? There are several reasons but two are particularly relevant ones. Firstly, Bayesian methods are fully consistent with mathematical logic, while conventional statistics are only logical when making probabilistic statements about data, not hypotheses (Cox, 1946; Berger and Berry, 1988; Jaynes, 2003). Bayesian methods can be used to make probabilistic predictions about the state of the world, while conventional statistics are restricted to statements about long-run averages obtained from hypothetical replicates of sampled data.
Secondly, relevant prior information can be incorporated naturally into Bayesian analyses by specifying the appropriate prior probabilities for the parameters. In contrast, conventional statistical methods are forced to ignore any relevant information other than that contained in the data. Difficulties with Bayesian methods and other benefits are discussed more fully in Chapter 2 and throughout this book.
Bayesian statistics are founded on the work of the Reverend Thomas Bayes, who lived and died in eighteenth century England (Box 1.1). Bayesian methods explicitly recognize and combine four
Box 1.1 The Reverend Thomas Bayes, FRS
Image not available in HTML version |
Very little is known about Thomas Bayes. The portrait above (O'Donnell, 1936) may be of Bayes, but no other portraits are known (Bellhouse, 2004). Even the year (1701 or 1702) and place of his birth (London or Hertfordshire, England) are uncertain (Dale, 1999). There are few records to indicate the nature of his early schooling, but he is known to have studied divinity and mathematics at the University of Edinburgh. He was ordained as a Presbyterian minister by 1728. He was elected as a Fellow of the Royal Society in 1742 but it was not until after his death in 1761 that his most famous contribution, his essay in the Philosophical Transactions of the Royal Society of London, was published (Bayes, 1763). In that essay, Bayes described his theory of probability and presented what is now known as Bayes' rule (or Bayes' theorem), establishing the basis of Bayesian statistics.
components of knowledge. Prior knowledge and new data are combined using a model to produce posterior knowledge.2 These four components may be represented as:
Displayed matter not available in HTML version |
It is common in everyday life to combine prior information and new data to update knowledge. We might hear a weather forecast that the chance of rain is small. However, if we stepped outside and saw dark clouds looming above us, most people would think that the risk of rain was higher than previously believed. In contrast, our expectation of a fine day would be reinforced by a sunny sky. Thus, both the prior information (the weather forecast) and the data (the current state of the weather) influence our newly updated belief in the prospects of rain.
Our updated belief in the chance of rain (the posterior) will depend on the relative weight we place on the prior information compared to the new data and the magnitude of the difference between the two pieces of information. In this case the ‘model’ is contained within our understanding of the weather. Our thought processes combine the prior information, data, and model to update our belief that it will rain. Bayesian statistics provide a logically consistent, objective and repeatable method for combining prior information with data to produce the posterior, rather than the subjective judgement that most people would use when stepping outside.
Before considering the benefits and limitations of Bayesian methods and its alternatives in Chapter 2, I will illustrate the use of the different statistical approaches with two examples. These highlight how Bayesian methods provide answers to the kinds of questions that ecologists ask, and how they can usefully incorporate prior information.
Example 1: Logic in determining the presence or absence of a species
Consider an ecologist who surveys ponds in a city for frogs. On her first visit to a pond, she searches the edge and listens for frog calls over a 20-minute period. The southern brown tree frog (Litoria ewingii) is the most common species in her study area, but it is not found on this particular visit (Fig. 1.2). However, the researcher would not be particularly surprised that the species was not detected because she knows from experience that when surveying ponds, southern brown tree frogs are detected on only 80% of visits when they are in fact present. Given this information, what can she conclude about whether the southern brown tree frog is present at the site or not?
The question about the presence of a species is a simple example of those asked by ecologists. We assume that there is a particular true state of nature and we hope to use scientific methods to determine a reasonable approximation of the truth. However, the probability that a species is
Image not available in HTML version |
present at a site is rarely calculated by ecologists, although it should be a fundamental part of any field study that depends on knowing where a species does and does not occur. This probability is not calculated partly because the statistical methods used by most ecologists are not well-suited to this question. I will examine three different approaches to answering this question and demonstrate that a satisfactory answer requires Bayesian methods.
Frequentist approaches
Conventional approaches to data analysis in ecology estimate the likelihood of observing the data (and more extreme data in the case of null hypothesis testing). These approaches are referred to as frequentist methods because they are based on the expected frequency that such data would be observed if the same procedure of data collection and analysis was implemented many times. Frequentist methods focus on the frequency with which the observed data are likely to be obtained from hypothetical replicates of sampling.
There are numerous types of frequentist statistics that are used in ecology, including null hypothesis significance testing and information-theoretic methods. These are applied below to the question about whether southern brown tree frogs are present at the pond.
Null hypothesis significance testing
The first statistical approach to answering the question is null hypothesis significance testing. The null hypothesis for this first case might be that the southern brown tree frog is absent from the site. The researcher then seeks to disprove the null hypothesis with the collection of data. The single piece of data in this case is that the frog was not detected. The researcher then asks: ‘What is the probability of obtaining this result if the null hypothesis were true?’3 This probability is the p-value of the significance test. If the p-value is sufficiently small (conventionally if less than 0.05), it means that the data (or more extreme data) would be unlikely to occur if the null hypothesis is true. If the p-value is small, then we assume that the data are inconsistent with the null hypothesis, which is then rejected in favour of the alternative.
In the case of the frog survey, the p-value is equal to 1.0. This is calculated as the probability that we would fail to record the frog (i.e. obtain the observed data) if it is absent (i.e. if the null hypothesis is true). The high p-value means that the researcher fails to reject the null hypothesis that the frog is absent.
The other possible null hypothesis is that the frog is present at the site. In this case, the probability of obtaining the data is equal to 0.2 (one minus the probability of detecting the species if present) given that the null hypothesis is true. Thus, the p-value is 0.2, and using a conventional cut-off of 0.05, the researcher would have a non-significant result. The researcher would fail to reject the null hypothesis that the southern brown tree frog was present.
It is surprising (to some people) that the two different null hypotheses can produce different results. The conclusion about whether the species is present or absent simply depends on which null hypothesis we choose. The source of this surprise is our failure to consider statistical power, which I will return to in Chapter 2.
Another possible source of surprise is that the p-value does not necessarily provide a reliable indicator of the support for the null hypotheses. For example, the p-value is equal to 1.0 for the null hypothesis that the frog is absent. This is the largest possible p-value, but it is still not proof that the null hypothesis is true. If we continued to return to the same pond and failed to find the frog, the p-value would remain equal to 1.0, insensitive to the accumulation of evidence that the frog is absent. This apparent discrepancy occurs because frequentist methods in general and p-values in particular do not provide direct statements about the reliability of hypotheses (Berger and Sellke, 1987; Berger and Berry, 1988). They provide direct information about the frequency of occurrence of data, which only gives indirect support for or against the hypotheses. In this way, frequentist methods are only partially consistent with mathematical logic, being confined to statements about data but not directly about hypotheses (Berger and Sellke, 1987; Jaynes, 2003).
Information theoretic methods
An information theoretic approach based on ‘likelihood’ is an alternative frequentist method to null hypothesis significance testing. It evaluates the consistency of the data with multiple competing hypotheses (Burnham and Anderson, 2002). In the current example, there are only two possible hypotheses: the frog is absent (Ha) and the frog is present (Hp). Likelihood-based methods ask: ‘What is the probability of observing the data under each of the competing hypotheses?’ In this example it is the probability of not detecting the species during a visit to a site.
Unlike null hypothesis testing, likelihood-based methods, including information-theoretic methods, do not consider the possibility of more extreme (unobserved) data. The likelihood for a given hypothesis can be calculated as the probability of obtaining the data given that the hypothesis is true.4 Despite the implication of its name, the likelihood of a hypothesis is not the same as the probability that the hypothesis is true.
Under the first hypothesis (the frog is absent), the probability of observing the data (Pr(D | Ha)) is equal to 1. Under the second hypothesis (the frog is present) the probability (Pr(D | Hp)) is 0.2. Information-theoretic methods then determine the amount of evidence in favour of these two hypotheses by examining the ratio of these values (Burnham and Anderson, 2002).5 These ratios may be interpreted by rules of thumb (see also Chapter 4). Using the criteria of Burnham and Anderson (2002), we might conclude that the southern brown tree frog is ‘considerably less’ likely to be present than it is to be absent (Pr(D | Hp)/Pr(D | Ha) = 1/5).
Bayesian methods
Frequentist methods are in general not well-suited to the species detection problem because they are strictly limited to assessing long-run averages rather than predicting individual observations (Quinn and Keough, 2002). This is revealing; frequentist methods are not strictly suitable for predicting whether a species is absent from a particular site when it has not been seen. Such a problem is fundamental in ecology, which relies on knowing the distribution of species. In contrast, the species detection problem can be tackled using Bayesian methods.
Bayesian methods are similar to likelihood-based methods, but also incorporate prior information using what is known as ‘prior probabilities’. Bayesian methods update estimates of the evidence in favour of the different hypotheses by combining the prior probabilities and the probabilities of obtaining the data under each of the hypotheses. The probability that a hypothesis is true increases if the data support it more than the competing hypotheses.
Why might the prior information be useful? If the researcher visited a pond that appeared to have excellent habitat for southern brown tree frogs (e.g. a large well-vegetated pond in a large leafy garden), then a failure to detect the species on a single visit would not necessarily make the researcher believe that the frog was absent. However, if the researcher visited a pond that was very unlikely to contain the frog (e.g. a concrete fountain in the middle of an asphalt car park), a single failure to detect the frog might be enough to convince the researcher that the southern brown tree frog did not occur at the pond. Frequentist methods cannot incorporate such prior information, but it is integral to Bayesian methods.
Another key difference between Bayesian methods and frequentist methods is that instead of asking: ‘What is the probability of observing the data given that the various hypotheses are true?’ Bayesian methods ask:
What is the probability of the hypotheses being true given the observed data?
At face value, this is a better approach for our problem because we are interested in the truth of the hypotheses (the frog's presence or absence at the site) rather than the probability of obtaining the observed data given different possible truths.
In practice, Bayesian methods differ from likelihood methods by weighting the likelihood values by the prior probabilities to obtain posterior probabilities. I will use the two symbols Pr(Ha) and Pr(Hp) to represent the prior probabilities. Therefore, the likelihood for the presence of the frog given that it was not seen (0.2) is weighted by Pr(Hp) and the likelihood for the absence of the frog (1.0) is weighted by Pr(Ha). Thus, the posterior probability of presence is a function of the prior probability Pr(Hp), the data (the frog was not seen) and the model, which describes how the data were generated conditional on the presence or absence of the frog. Now we must determine a coherent scheme for determining the values for the prior probabilities Pr(Hp) and Pr(Ha). This incorporation of prior information is one of the unique aspects of Bayesian statistics. It also generates the most controversy.
Both hypotheses might be equally likely (prior to observing the data) if half the sites in the study area were occupied by southern brown tree frogs (Parris unpublished data). In this case, Pr(Ha) = 0.5, as does Pr(Hp). With these priors, the probability of the southern brown tree frog being absent will be proportional to 0.5 × 1.0 = 0.5, and the probability of it being present will be proportional to 0.5 × 0.2 = 0.1.
The posterior probabilities must sum to one, so these proportional values (0.5 and 0.1) can be converted to posterior probabilities by dividing by their sum (0.5 + 0.1 = 0.6). Therefore, the probability of the frog being present is 1/6 (= 0.1/0.6), and the probability of absence is 5/6 (= 0.5/0.6). So, with equal prior probabilities (Pr(Ha) = Pr(Hp) = 0.5), we would conclude that the presence of the frog is five times less probable than the absence of the frog because the ratio (Pr(Hp | D)/Pr(Ha | D)) equals 1/5. You may have noticed that this result is numerically identical to the likelihood-based result. I will return to this point later.
A different prior could have been chosen for the analysis. A statistical model predicts the probability of occupancy of ponds by southern brown tree frogs based on the level of urbanization (measured by road density), characteristics of the vegetation, and the size of the pond (based on Parris 2006.). If the pond represented relatively high-quality habitat, with a predicted probability of occupancy of 0.75, then the probability of the frog being present will be proportional to 0.75 × 0.2 = 0.15 and the probability of absence will be proportional to (1 – 0.75) × 1.0 = 0.25. With these priors, the probability of the frog being present is equal to 3/8 (= 0.15/(0.15 + 0.25)), and the probability of absence is 5/8 (= 0.25/(0.15 + 0.25)).
The incorporation of prior information (the presence of good quality habitat) increases the probability that the pond is occupied by southern brown tree frogs compared to when the prior information is ignored (0.375 versus 0.167). The actual occupancy has not changed at all – the pond is still either occupied or not. What has changed is the researcher's belief in whether the pond is occupied. These Bayesian analyses may be formalized using Bayes' rule, which, following a short introduction to conditional probability (Box 1.2), is given in Box 1.3.
Box 1.2 Conditional probability
Bayes' rule is based on conditional probability. Consider two events: event C and event D. We are interested in the probability of event C occurring given event D has occurred. I will write this probability using the symbol Pr(C | D), and introduce three more symbols:
- Pr(C) – the probability of event C occurring;
- Pr(D) – the probability of event D occurring; and
- Pr(C and D) – the probability of both events occurring together.
Conditional probability theory tells us that:
Pr(C and D) = Pr(D) × Pr(C | D),
which in words is: the probability of events C and D both occurring is equal to the probability of event C occurring given that event D has occurred multiplied by the probability of event D occurring (independent of event C ). The | symbol means ‘given the truth or occurrence of ’.
The above can be rearranged to give:
Pr(C | D) = Pr(C and D) / Pr(D).
For example, Pfiesteria, a toxic alga is present in samples with probability 0.03 (Stow and Borsuk 2003). Pfiesteria is a subset of Pfiesteria-like organisms (PLOs), the latter being present in samples with probability 0.35. Therefore, we can calculate the conditional probability that Pfiesteria is present given that PLOs are present:
Pr(Pfiesteria | PLO) = Pr(Pfiesteria and PLO) / Pr(PLO)
= 0.03/0.35 = 0.086.
Box 1.3 Bayes' rule for a finite number of hypotheses
Conditional probability (Box 1.2) states that for two events C and D:
Pr(C and D) = Pr (D) × Pr(C | D).
C and D are simply labels for events (outcomes) that can be swapped arbitrarily, so the following is also true:
Pr(D and C) = Pr(C) × Pr(D | C).
These two equivalent expressions for Pr(C and D) can be set equal to each other:
Pr(D) × Pr(C | D) = Pr(C) × Pr(D | C).
It is then straightforward to obtain:
Pr(C | D) = Pr(C) × Pr(D | C) / Pr(D).
Let us assume that event C is that a particular hypothesis is true, and event D is the occurrence of the data. Then, the posterior probability that the frog is absent given the data (Pr(Ha | D)) is:
Pr(Ha |D) = Pr(Ha) × Pr(D|Ha) / Pr(D).
The various components of the equation are the prior probability that the frog is absent (Pr(Ha)), the probability of obtaining the data given that it is absent (Pr(D | Ha), which is the likelihood), and the probability of obtaining the data independent of the hypothesis being considered (Pr(D)).
The probability of obtaining the data (the frog was not detected) given Ha is true (the frog is absent) was provided when using the likelihood-based methods:
Pr(D | Ha) = 1.0.
Similarly, given the presence of the frog:
Pr(D | Hp) = 0.2.
The value of Pr(D) is the same regardless of the hypothesis being considered (Hp the frog is present, or Ha the frog is absent), so it simply acts as a scaling constant. Therefore, Pr(Ha | D) is proportional to Pr(Ha) × Pr(D | Ha), and Pr(Hp | D) is proportional to Pr(Hp) × Pr(D | Hp), with both expressions having the same constant of proportionality (1/Pr(D)).
Pr(D) is calculated as the sum of the values Pr(H) × Pr(D | H) under all hypotheses. When prior probabilities are equal (Pr(Ha) = Pr(Hp) = 0.5):
Pr(D) = [Pr(Ha) × Pr(D | Ha)] + [Pr(Hp) × Pr(D | Hp)]
= (0.5 × 1) + (0.5 × 0.2) = 0.6.
Therefore, the posterior probabilities are 5/6 (0.5/0.6) for the absence of the frog, and 1/6 (0.1/0.6) for the presence of the frog.
So, for a finite number of hypotheses, Bayes' rule states that the probability of the hypothesis given the data is calculated using the prior probabilities of the different hypotheses (Pr(Hj)) and the probability of obtaining the data given the hypotheses (Pr(D | Hj)):
Display matter not available in HTML version |
This expression uses the mathematical notation for summation ∑.
If on the other hand, the pond had poor habitat for southern brown tree frogs, the prior probability of presence might be 0.1. Thus, Pr(Hp) = 0.1 and Pr(Ha) = 0.9. As before, Pr(D | Hp) = 0.2 and Pr(D | Ha) = 1.0. Note that the values for the priors but not the likelihoods have changed. Using Bayes' rule (Box 1.3), the posterior probability of presence is:
Pr(Hp | D) = Pr(Hp) × Pr(D | Hp) / [Pr(Hp) ×Pr(D | Hp) + Pr(Ha) × Pr(D | Hp)]
= 0.1 × 0.2 / [0.1 × 0.2 + 0.9 × 1.0]
= 0.022
Therefore, there is only a small chance that the frog is at the site if it has poor habitat and the species is not detected on a single visit.
1 The conventional statistical methods are known as frequentist statistics and include null hypothesis significance testing (NHST) and construction of confidence intervals. NHST attracts the most criticism. See Chapter 2 for more details of these methods.
2 Prior and posterior refer to before and after considering the data.
3 In actual fact, a null hypothesis significance test asks what is the probability of obtaining the data or a more extreme result. However, in this case, a more extreme result is not possible; it is not possible to fail to detect the frog more than once with one visit, so the p-value is simply the probability of observing the data.
4 The likelihood need only be proportional to the probability of obtaining the data, not strictly equal to it. Terms that do not include the data or the parameters being estimated can be ignored because they will cancel out of the subsequent calculations.
5 Information-theoretic methods are modified by the number of parameters that are estimated with the data. In this case, the parameter of the analyses (the detection rate) is not estimated with the data, so the number of estimated parameters is zero.
© Cambridge University Press