Boosting is an approach to machine learning based on the idea of creating a highly accurate predictor by combining many weak and inaccurate “rules of thumb.” A remarkably rich theory has evolved around boosting, with connections to a range of topics, including statistics, game theory, convex optimization, and information geometry. Boosting algorithms have also enjoyed practical success in such fields as biology, vision, and speech processing. At various times in its history, boosting has been perceived as mysterious, controversial, even paradoxical.
This book, written by the inventors of the method, brings together, organizes, simplifies, and substantially extends two decades of research on boosting, presenting both theory and applications in a way that is accessible to readers from diverse backgrounds while also providing an authoritative reference for advanced researchers. With its introductory treatment of all material and its inclusion of exercises in every chapter, the book is appropriate for course use as well.
The book begins with a general introduction to machine learning algorithms and their analysis; then explores the core theory of boosting, especially its ability to generalize; examines some of the myriad other theoretical viewpoints that help to explain and understand boosting; provides practical extensions of boosting for more complex learning problems; and finally presents a number of advanced theoretical topics. Numerous applications and practical illustrations are offered throughout.
Boosting is an approach to machine learning based on the idea of creating a highly accurate predictor by combining many weak and inaccurate “rules of thumb.” A remarkably rich theory has evolved around boosting, with connections to a range of topics, including statistics, game theory, convex optimization, and information geometry. Boosting algorithms have also enjoyed practical success in such fields as biology, vision, and speech processing. At various times in its history, boosting has been perceived as mysterious, controversial, even paradoxical.
This book, written by the inventors of the method, brings together, organizes, simplifies, and substantially extends two decades of research on boosting, presenting both theory and applications in a way that is accessible to readers from diverse backgrounds while also providing an authoritative reference for advanced researchers. With its introductory treatment of all material and its inclusion of exercises in every chapter, the book is appropriate for course use as well.
The book begins with a general introduction to machine learning algorithms and their analysis; then explores the core theory of boosting, especially its ability to generalize; examines some of the myriad other theoretical viewpoints that help to explain and understand boosting; provides practical extensions of boosting for more complex learning problems; and finally presents a number of advanced theoretical topics. Numerous applications and practical illustrations are offered throughout.
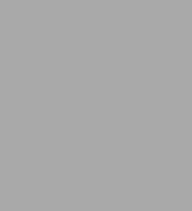
Boosting: Foundations and Algorithms
544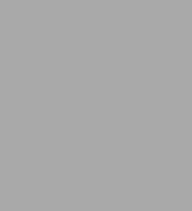
Boosting: Foundations and Algorithms
544Paperback
Product Details
ISBN-13: | 9780262526036 |
---|---|
Publisher: | MIT Press |
Publication date: | 01/10/2014 |
Series: | Adaptive Computation and Machine Learning series |
Pages: | 544 |
Product dimensions: | 6.60(w) x 8.60(h) x 1.00(d) |
Age Range: | 18 Years |