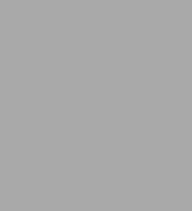
Computational and Structural Approaches to Drug Discovery: Ligand-Protein Interactions
400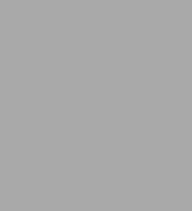
Computational and Structural Approaches to Drug Discovery: Ligand-Protein Interactions
400Hardcover
-
SHIP THIS ITEMIn stock. Ships in 1-2 days.PICK UP IN STORE
Your local store may have stock of this item.
Available within 2 business hours
Related collections and offers
Overview
Product Details
ISBN-13: | 9780854043651 |
---|---|
Publisher: | RSC |
Publication date: | 10/26/2007 |
Series: | RSC Biomolecular Sciences , #8 |
Pages: | 400 |
Product dimensions: | 6.30(w) x 9.30(h) x 1.00(d) |
About the Author
Read an Excerpt
Computational and Structural Approaches to Drug Discovery
Ligand Protein Interactions
By Robert M Stroud, Janet Finer-Moore
The Royal Society of Chemistry
Copyright © 2008 The Royal Society of ChemistryAll rights reserved.
ISBN: 978-0-85404-365-1
CHAPTER 1
Facing the Wall in Computationally Based Approaches to Drug Discovery
JANET S. FINER-MOORE, JEFF BLANEY AND ROBERT M. STROUD*
1.1 The Promise, and the Problem
It has been 36 years since the first protein structure was determined and the promise that structure could guide drug discovery was born.' Yet today even the ability to design a molecule that will target a nominated site and bind there still remains a tantalizing and intellectually enticing prospect. Most good lead compounds fail for reasons to do with lack of efficacy, toxicity, or interference with metabolic pathways. These properties, too, are ripe for computational evaluation before synthesis rather than after. These important areas are being addressed by computational approaches. But the real challenge for drug design is in the intellectual process of appreciating what is actually coded within macromolecular interactions of the target with small ligands, and the nature of specificity for metabolic enzymes that degrade the compound. This code is at the heart of biology, as it is of chemistry.
Let us first recognize the impact that just one, rather incremental, computational approach could have on the process. If it were possible, we might be able to take the crystal structure of a good lead compound and predict where introducing a specific substituent would increase affinity of the compound with even a 10% chance of moving affinity in the favorable direction. This could provide for successive improvements in affinity of the lead compound with just 10 alternative chemical synthetic modifications at each round, one of which would be an improvement (presuming the 10% chance of success). But there is a wall beyond which the probability of success becomes very low. If we could even recognize the cusp at which that will happen ahead of hitting 'the wall', alternative scaffolds could be introduced. This would save enormous resources in chemistry. The same is true for many steps, such as predicting metabolic fate or toxicity of compounds. A small improvement coded in computational screening can reduce downstream efforts by factors of hundreds to thousands. Hence the rational encoding of valid principles, and even empirical wisdom, into the process will remain a key to human health, and one of the highest intellectual challenges of the next decade.
Another area of drug discovery that is hungry for computational assistance is the personalization of medicine. Why is it that some persons have devastating, even life-threatening, responses to Celebrex or Vioxx, while others find them to be the best treatment? Genetic screening is required, but the translation of this into therapeutic strategy is paramount to the salvage of vast potential in the efforts that have already been made to develop potentially good drugs, that could be highly valuable, though for only a defined cohort of the population. If we could anticipate the patients who would respond badly we would save billion dollar markets for present and future drugs. In many ways we are at an exciting frontier in drug discovery, and a frontier where computational biology will need to take the lead.
Lead discovery in the traditional pharmaceutical industry typically involves screening of up to 5 million chemical entities in a few weeks using high-throughput methods, at a cost of $0.50-$ 1.00 for each compound. A screen of that local library could easily cost $5000000. There is consequently real motivation to maximize the return on investment by finding fast and more effective ways to screen. The time and money are compounded by the need to test lead compounds and derivatives for absorption, distribution, metabolism, and excretion (ADME) and toxicity. The spectrum of activity against off-target proteins is something rarely built into even computational screens, and is often left simply to trials on cells, animals, and then humans. With such a wealth of knowledge gathered over decades, why are we not further along in proscribing affinity and favorable properties?
We are in the adolescence of drug discovery. As even empirical rules emerge it is increasingly pertinent that those rules be incorporated into computational analysis, then understood, and then translated into rational rules and algorithms. Ultimately the computer will extract everything we learn in our laboratories, and translate that knowledge into 'wisdom'. Can we blame the industrial leader who shuns the knowledge-based approach for the tried-and-true screening approach to lead discovery? As the knowledge base explodes, the intellectual ability to understand that knowledge and translate it into improved drug discovery has to catch up and demonstrate its contribution to enhanced drug design. Unfortunately much of the knowledge of the binding affinities of series of compounds is sometimes protected, or otherwise unpublished in the knowledge bases of pharmaceutical companies. Another great challenge for the industry and for computational approaches is to release and collate the combined knowledge across the scientific community, for that could help chart the course between scaffolds or elaborations that we should avoid. Some of these areas are already in good hands, though are not yet established, recognized, or used routinely. Like the turn of the tide in an inland sea, the tide often turns long before the reverse flow begins.
The difference between a lead compound and a drug-like compound, or a drug, involves an increase in complexity, and can be encoded in a Venn diagram. This reminds us that optimizing a lead generally develops a compound of modest affinity for its target protein site, to one with drug-like properties, to one with increased affinity and specificity for the target. The requirements for each of these three types of compounds are inherently different. A tool compound for elaboration has different requirements to the drug-like compound, or the final drug. Likewise the context of use for a drug presents different requirements depending on clinical circumstances or the chosen mode of delivery. The classic paper of Lipinski et al. sought to address the frustration at Pfizer on finding that many hits were not optimizable. Thus new and separate criteria can now be described for leads, and for drug-like compounds, and these criteria can be phased in during development.
The challenge to make drug discovery a more rational process is balanced by the need to develop new drugs. The theorist enjoys some freedom to evolve and test ideas and produce algorithms for 'docking', 'fragment linking', scoring theoretical libraries, defining algorithms that predict ADME/Toxicity well, etc. The real thermometer of how well we can do relies on heavy commitment from the pharmaceutical industry to take the best ideas and algorithms of the moment and test them in a way that pushes them against the real criteria of making drugs. Even the most conservative chemists will readily take advantage of protein structure as a guide, but perhaps not as a driving plan for the next design, in part because of the limitations in its 'interpretation'. And the insights from a crystal structure are chemical and not well coordinated with perspectives of toxicity or other physiological properties. The balance of the 'tide' here reflects both the needs of the professional medicinal chemist to produce compounds, and the need to better generate understanding and rules that can at least bias toward improved properties. Thus the intellectual quest for rationality and the practical need to produce something quantifiable beautifully constrain each other into one of the grand challenges of our generation. There is a beautiful synergy of ideas and objective evaluation. The solution requires a much closer open connection between the vast experience of the pharmaceutical industry and the academic, since industry has less incentive for interpreting failure at any iteration, and academics lack the primary knowledge that is largely inaccessible.
1.2 Current Limitations in Structure-guided Lead Design
The structure of a lead compound bound to a drug target protein is powerful in directing alterations to the lead compound that produce greater affinity, but only up to a point. There seems to be a bounded space within which current schemes prevail, even when one accounts for protein flexibility in some manner. What constitutes the wall beyond which further alteration does not improve affinity, or 'efficiency' (the free energy divided by the number of atoms)? Surely if we understood the intermolecular code, we should be able to continue to optimize a lead, at least in affinity for its target, by logical addition of functional groups. In many ways getting past this 'wall' in optimization remains one of the most promising, rewarding, and challenging goals in pharmaceutical chemistry, and in chemical biology.
The process of interpreting the structure of a protein target with a bound fragment, and using that knowledge to design an improvement in binding affinity (arguably one of the easier parts of drug discovery) will be understood and encoded in time. There are some obvious weaknesses in our current analyses. Free energies of associations are not additive because binding entropy is not additive, and the contribution of entropy to the binding free energy is hard to encode, especially the entropy contributions from water molecules that are displaced from drug and site on drug binding to the site. This can be seen, for example, in mutational analysis that removes one, then another interaction. In a case where the crystal structures indicate no significant change in the overall interaction or dynamics, the loss of the first, no matter which one, is small, while the second produces a large effect. Also the hydrophobic effect is a major determinant of binding that is not easily incorporated into the exact 'Newtonian' approach to molecular mechanics. Combination of 'atomic solvation parameters' with the force fields that attempt to describe the 'Newtonian' forces yields improved results. Yet the necessity for empirical solvation parameters also reminds us that molecular mechanics does not yet adequately deal with the solvation and desolvation that occurs when a ligand binds to a macromolecule.
In principle, freeing of an ordered water molecule while an interface is made can yield an entropy-driven advantage of about ΔG ≈ TΔS = RT In W where W is the number of degrees of freedom generated. Thus W ≈ 6 for a freed water molecule (three translational and three rotational degrees of freedom) so ΔG ≈ 0.6 In 6 ≈ 1.2 kcal mol-1, or a factor of 10 in Kd. Displacement of water contributed to the binding affinity of the human immunodeficiency virus (HIV) protease cyclic urea drugs produced by Merck. However, other water molecules become ordered to make the interface. We are not yet able to pay enough attention to the enormous contribution of water. Free energy perturbation methods provide one of the more exact ways of taking what we know of molecular interactions and testing small alterations to ask what will be the consequence.
One limitation to structure-based guidance of drug discovery lies in crystallography itself. Clearly in order to predict molecular behavior we need more than a single set of coordinates that represents the electronic structure of a molecular complex, which is what the usual interpretation of a crystal structure most readily provides. Flexibility is both a key to the ability of the protein to adapt to and bind different chemical moieties, and a basis for relatively poor compatibility of others. Thus it is an essential element in computational approaches. From its inception protein crystallography has struggled to minimize the parameters used to define structure, in order to maintain a good data to parameter ratio, a typical ratio being — 10:1 at a resolution of ~2 Å. The results are usually a median structure, perhaps with occasional alternative conformations in certain positions, and a set of isotropic B factors, which are used to account for thermal vibrations. The parsimonious set of parameters defining the structure is all that can be justified based on the number of observations. With restraints in refinement there are perhaps four to five torsional parameters and eight 'B factors' per amino acid. The B factors really represent the radius of a distribution of trapped states in their librational trajectories that are far from harmonic, or anharmonic. The focus of crystallography has been on developing technology that ensures the quality of the atomic-level median structures. Rather different issues are important for drug development. Drug discovery needs an understanding of the dynamic trajectories of the protein chain, the change in the drug lead on forming a complex with the target, and the change in solvent organization, in order to estimate the various steric, strain, and entropic contributions to binding. Very few of us have interpreted X-ray structures in a way that would allow incorporation of anharmonic motion and of changes in entropy of the side chains and water into structure-guided ligand design. This remains a 21st century challenge that is within reach even now. At the very least protein structures used for these purposes should refine a manifold of conformers in the region of any binding site.
One of the ways to appreciate the nature of the prospects and limitations is to track the history of efforts in one highly validated drug target of high impact for human health. These criteria guarantee that the best attempts from all routes available for drug discovery have been stretched and harnessed cooperatively with each other. Therefore they provide tracks to successful drugs, cautionary tales, lessons for how methods can contribute to the process, and a focus on where new improvements are most needed. Thymidylate synthase (TS) is one such example. It has been recognized as a target for antiproliferative anticancer drugs for 50 years, the first structure was determined 20 years ago, and the landscape has been comprehensively mapped ever since. Its mechanism is among the most thoroughly characterized by chemistry, mutational analysis, and crystal structures.'
1.3 Lessons in Structure-based Drug Design from Thymidylate Synthase
1.3.1 Mechanism-based Inhibitors and Enzyme-catalyzed Therapeutics
In our own laboratory and elsewhere TS has been a rewarding test bed for computational methods of structure-guided drug discovery. TS is essential for DNA replication, especially in transformed cells, and so has been one of the key targets for anticancer drugs. It became one of the first targets for mechanism-based drug design in 1983, with Heidelberger's discovery of 5-fluorouracil. 5-Fluorouracil is converted into 5-fluoro-2'-deoxyuridine-5'-monophosphate (FdUMP), an analog of the TS substrate 2'-deoxyuridine-5'-monophosphate (dUMP), inside cells. Like dUMP, FdUMP forms a covalent ternary complex with the enzyme and cofactor, but is unable to proceed through subsequent catalytic steps. Structure-activity relationships (SARs) for other dUMP analogs indicated that only C5 on the dUMP base could be extensively modified without loss of affinity for TS. The reasons for this became clear when we determined the crystal structure of TS. The preformed dUMP-binding site included side chains or backbone amides within hydrogen-bonding distance of every heteroatom of the pyrimidine ring, and only C5, the position that would become methylated to produce thymidine, was surrounded by enough space to accommodate a bulky substituent.
The structure of TS allowed rational design of a new class of mechanism-based drugs, named 'enzyme-catalyzed therapeutic agents' (ECTAs). These compounds were non-toxic C5-substituted dUMP analogs that were metabolized to release toxic compounds in the rapidly dividing cancer cells via the initial steps of TS catalysis. Cancer cells that had developed resistance to TS inhibitors through overproduction of TS were most susceptible to ECTAs.
(Continues...)
Excerpted from Computational and Structural Approaches to Drug Discovery by Robert M Stroud, Janet Finer-Moore. Copyright © 2008 The Royal Society of Chemistry. Excerpted by permission of The Royal Society of Chemistry.
All rights reserved. No part of this excerpt may be reproduced or reprinted without permission in writing from the publisher.
Excerpts are provided by Dial-A-Book Inc. solely for the personal use of visitors to this web site.
Table of Contents
Preface: Section 1 Overview: 1 Facing the Wall in Computationally Based Approaches to Drug Discovery;1.1 The promise, and the problem;
1.2 Current limitations in structure-guided lead design;
1.3 Lessons in structure-based drug design from thymidylate synthase research;
1.3.1 Mechanism-based inhibitors and enzyme-catalyzed therapeutics;
1.3.2 Iterative structure-based drug design;
1.3.3 Docking, fragments and optimizability;
1.4 New developments in structure-based drug-design methods;
1.4.1 Fragment-based methods;
1.4.2 Identifying drug target sites on a protein;
1.4.3 Targeting protein-protein interactions;
1.4.4 Computational docking to nominated sites;
1.5 Conclusion;
References;
2 The Changing Landscape in Drug Discovery;
2.1 Introduction;
2.2 QSAR - understanding without prediction;
2.3 Gene technology - from mice to humans;
2.4 Combinatorial library design - driven by medicinal chemistry;
2.5 Docking and scoring - solved and unsolved problems;
2.6 Virtual screening - the road to success;
2.7 Fragment-based and combinatorial design - a new challenge;
2.8 Summary and conclusions;
References;
Section 2: Structure-Based Design;
3 Purine Nucleoside Phosphorylase;
3.1 Introduction;
3.2 Three-dimensional structures of PNPs;
3.3 Related enzymes of the PNP family;
3.4 PNP active sites;
3.5 Human PNP inhibitors;
3.6 Other applications of molecular design to PNP;
3.7 Applications of molecular design to enzymes related to PNP;
3.8 PNP inhibitors and clinical trials;
3.9 Conclusions and future directions;
References;
4 Application and Limitations of X-Ray Crystallographic Data in Structure-Based Ligand and Drug Design;
4.1. Introduction;
4.2. Structure-based ligand design and drug design;
4.3 Some limitations in the use of X-ray data;
4.3.1 Basic crystallography terms;
4.3.2 Uncertainty in the identity or location of protein or ligand atoms;
4.3.3 Effect of crystallization conditions;
4.3.4 Identification and location of water;
4.4 Macromolecular structures to determine small-molecule structures;
4.5 Assessing the validity of structure models;
4.6 Summary and Outlook;
References;
5 Dealing with Bound Waters in a Site: Do they Leave or Stay?;
5.1 Introduction;
5.2 Localized water molecules in binding sites of proteins;
5.3 Identifying localized water molecules from computer simulations;
5.4 Calculation of free-energy cost of displacing a site-bound water molecule;
5.5 Inclusion of explicit water molecules in drug discovery;
Acknowledgements;
References;
6 Knowledge-Based Methods in Structure-Based Design;
6.1 Introduction;
6.2 Atom-based potentials;
6.3 Group-based potentials;
6.4 Methodologies;
6.4.1 The reference state;
6.4.2 Volume corrections;
6.5 Applications;
6.5.1 Visualization and interaction 'hot spots';
6.5.2 Docking and scoring;
6.5.3 De novo design;
6.5.4 Targeted scoring functions;
6.6 Discussion;
6.7 Conclusion;
References;
7 Combating Drug Resistance -Identifying Resilient Molecular Targets and Robust Drugs;
7.1 Introduction;
7.2 Resilient targets and robust drugs;
7.3 Example of HIV-1 protease: substrate recognition vs. drug resistance;
7.4 Implications for future structure-based drug design;
Acknowledgements;
References;
Section 3: Docking;
8 Docking Algorithms and Scoring Functions;
State-of-the-Art and Current Limitations;
8.1 Introduction;
8.1.1 Binding mode prediction;
8.1.2 Virtual screening for lead identification;
8.1.3 Potency prediction for lead optimization;
8.2 A brief review of recent docking evaluations;
8.3 What these evaluations tell us about the performance of docking algorithms;
8.3.1 Binding mode prediction;
8.3.2 Virtual screening;
8.3.3 Affinity prediction;
8.4 How an ideal evaluation data set might be structured;
8.4.1 Binding mode prediction;
8.4.2 Virtual screening;
8.4.3 Affinity prediction;
8.5 Concluding remarks;
8.5.1 Binding mode prediction;
8.5.2 Virtual screening;
8.5.3 Rank order by affinity;
8.5.4 The state-of-the-art;
References;
9 Application of Docking Methods to Structure-Based Drug Design;
9.1 Introduction;
9.2.1 Molecule preparation;
9.2.2 Sampling methods;
9.2.3 Scoring methods;
9.2.4 Managing errors in docking;
9.4 Docking methods, capabilities and limitations;
9.5 Summary;
References;
10 Strength in Flexibility: Modeling Side-Chain Conformational Change in Docking and Screening;
10.1 Introduction;
10.2 Background;
10.2.1 Improving docking and screening through side-chain flexibility modeling;
10.2.2 Enhancing target specificity through flexibility modeling;
10.3 Approaches;
10.3.1 The state of the art in modeling protein side-chain flexibility;
10.3.2 Learning from nature: observing side-chain motions upon ligand binding;
10.4 The future: knowledge-based modeling of side-chain motions;
Acknowledgements;
References;
11 Avoiding the rigid receptor: side chain rotamers;
11.1 Introduction;
11.2 Rotamer libraries;
11.3 Successful applications of rotamer libraries in drug design;
11.3.1 Aspartic acid protease inhibitors;
11.3.2 Matrix metalloproteinase-1 inhibitors;
11.3.3 Thymidylate synthase inhibitors;
11.3.4 Protein tyrosine phosphatase 1B inhibitors;
11.3.5 HIV protease drug-resistant mutants bound to inhibitors;
11.3.6 Trypsin-benzamidine and phosphocholine-McPC 603;
11.4 Conclusions;
Acknowledgements;
References;
Section 4: Screening;
12 Computational Prediction of Aqueous Solubility, Oral Bioavailability, P450 Activity and hERG Channel Blockade;
12.1 Introduction;
12.2 Aqueous solubility;
12.3 Oral bioavailability;
12.4 P450 activity;
12.5 hERG channel blockade;
12.6 Conclusions;
References;
13 Shadows on Screens;
13.1 Introduction;
13.2 Phenomenology of aggregation;
13.3 What sort of compounds aggregate?;
13.4 Mechanism of aggregation-based inhibition;
13.5 A rapid counter-screen for aggregation-based inhibitors;
13.6 Biological implications?;
13.7 The spirit-haunted world of screening;
Acknowledgements;
References;
14 Iterative Docking Strategies for Virtual Ligand Screening;
14.1 Introduction;
14.2 AutoDock background;
14.2.1 Scoring function;
14.2.2 Search function;
14.2.3 AutoDockTools;
14.2.4 AutoDockTools analysis;
14.3 Diversity-based virtual ligand screening;
14.3.1 AICAR transformylase;
14.3.2 Protein phosphatase 2C;
14.4 Comparison with existing VLS strategies;
14.4.1 Hierarchical VLS;
14.4.2 Monolithic VLS strategy;
14.5 Other AutoDock VLS strategies;
14.5.1 Acetylcholine esterase peripheral anionic site;
14.5.2 Human P2Y1 receptor;
14.6 Diversity-based VLS issues;
14.6.1 Library choice;
14.6.2 Similarity search;
14.6.3 Apo versus ligand-bound docking models;
14.6.4 Binding site choices;
14.7 Future work;
References;
15Challenges and Progresses in Calculations of Binding Free Energies;
What Does it Take to Quantify Electrostatic Contributions to Protein-Ligand Interactions;
15.1 Introduction;
15.2 Computational strategies;
15.2.1 Free-energy perturbation, linear response approximation and potential of mean force calculations by all-atom models;
15.2.2 Proper and improper treatments of long-range effects in all-atom models;
15.2.3 Calculations of electrostatic energies by simplified models;
15.3 Calculating binding free energies;
15.3.1 Studies of drug mutations by FEP approaches;
15.3.2 Evaluation of absolute binding energies by the LRA and LIE approaches;
15.3.3 Using semi-macroscopic and macroscopic approaches in studies of ligand binding;
15.3.4 Protein-protein interactions;
15.4 Challenges and new advances;
15.5 Perspectives;
Acknowledgements;
References;
Section 5: Fragment-Based Design;
16 Discovery and Extrapolation of Fragment Structures towards Drug Design;
16.1 Structure-based approaches to drug discovery;
16.2 Properties of molecular fragments;
16.3 From molecular fragments to drug leads;
16.3.1 Fragment growing;
16.3.2 Fragment linking;
16.3.3 Fragment assembly;
16.4 Screening and identification of fragments;
16.5 X-Ray crystallography for fragment-based lead identification;
16.6 NMR spectroscopy;
16.6.1 Protein-based methods: structure-activity relationship by NMR;
16.6.2 Ligand-based methods;
16.7 Mass spectrometry;
16.7.1 Covalent mass spectrometric methods;
16.7.2 Non-covalent mass spectrometric methods;
16.7.3 Looking at the protein or the ligand;
16.8 Thermal shift;
16.9 Isothermal titration calorimetry;
16.10 Surface plasmon resonance;
16.11 Concluding remarks;
Acknowledgements;
References;
17 A Link Means a Lot: Disulfide Tethering in Structure-Based Drug Design;
17.1 Introduction: what is disulfide Tethering?;
17.2 Successes of native cysteine Tethering;
17.3 Role of structure in engineered-cysteine Tethering;
17.4 Cooperative Tethering;
17.5 Extended Tethering;
17.6 Break-away Tethering;
17.7 Discovery of novel allosteric sites with Tethering;
17.8 Tethering as a validation tool;
17.9 Tethering vs. traditional medicinal chemistry;
17.10 Tethering in structure determination;
17.11 The challenge of covalency;
17.12 Hydrophobic binders;
17.13 Conclusions: the future of Tethering;
References;
18 The Impact of Protein Kinase Structures on Drug Discovery;
18.1 Introduction;
18.2 The hinge region and the concept of kinase inhibitor scaffold;
18.3 High-throughput crystallography for the discovery of novel scaffolds;
18.3.1;
High-throughput crystallography for the discovery of novel scaffolds;
18.3.2 Low potency, low specificity, and low molecular weight screening;
18.4 The gatekeeper residue and the selectivity pocket;
18.5 The conformational states of the DFG motif and the opening of the back pocket;
18.6 Allosteric inhibitors, non-ATP competitive inhibitors, and irreversible inhibitors;
18.7 Discovering kinase inhibitors in a 500-dimensional space;
Acknowledgements;
References;
What People are Saying About This
The book has been carefully constructed as a series of essays, each addressing an important topic, and even better, topics, that there are many misunderstandings and misconceptions about. Each chapter is well referenced and serves as a good entry point into the literature. I would also strongly recommend this book to medicinal chemists leading project teams that use structural information.