5
1
9781412970761
Computational Modeling in Cognition: Principles and Practice / Edition 1 available in Paperback, eBook
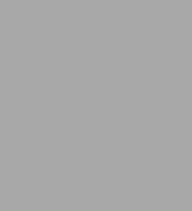
Computational Modeling in Cognition: Principles and Practice / Edition 1
- ISBN-10:
- 1412970768
- ISBN-13:
- 9781412970761
- Pub. Date:
- 11/29/2010
- Publisher:
- SAGE Publications
- ISBN-10:
- 1412970768
- ISBN-13:
- 9781412970761
- Pub. Date:
- 11/29/2010
- Publisher:
- SAGE Publications
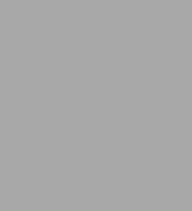
Computational Modeling in Cognition: Principles and Practice / Edition 1
$129.0
Current price is , Original price is $129.0. You
$129.00
Temporarily Out of Stock Online
Temporarily Out of Stock Online
129.0
Out Of Stock
Product Details
ISBN-13: | 9781412970761 |
---|---|
Publisher: | SAGE Publications |
Publication date: | 11/29/2010 |
Pages: | 359 |
Product dimensions: | 6.00(w) x 8.90(h) x 0.90(d) |
From the B&N Reads Blog