The book then details the thought behind CUDA and teaches how to create, analyze, and debug CUDA applications. Throughout, the focus is on software engineering issues: how to use CUDA in the context of existing application code, with existing compilers, languages, software tools, and industry-standard API libraries.
Using an approach refined in a series of well-received articles at Dr Dobb's Journal, author Rob Farber takes the reader step-by-step from fundamentals to implementation, moving from language theory to practical coding.
The book then details the thought behind CUDA and teaches how to create, analyze, and debug CUDA applications. Throughout, the focus is on software engineering issues: how to use CUDA in the context of existing application code, with existing compilers, languages, software tools, and industry-standard API libraries.
Using an approach refined in a series of well-received articles at Dr Dobb's Journal, author Rob Farber takes the reader step-by-step from fundamentals to implementation, moving from language theory to practical coding.
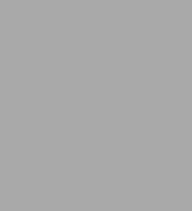
CUDA Application Design and Development
336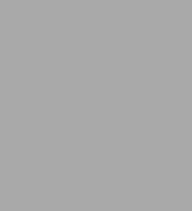
CUDA Application Design and Development
336Product Details
ISBN-13: | 9780123884268 |
---|---|
Publisher: | Elsevier Science |
Publication date: | 10/31/2011 |
Pages: | 336 |
Product dimensions: | 7.46(w) x 9.18(h) x 0.82(d) |