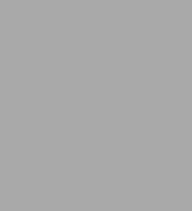
Database Design: Know It All
368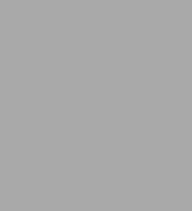
Database Design: Know It All
368eBook
Available on Compatible NOOK devices, the free NOOK App and in My Digital Library.
Related collections and offers
Overview
Product Details
ISBN-13: | 9780080877891 |
---|---|
Publisher: | Morgan Kaufmann Publishers |
Publication date: | 10/23/2008 |
Sold by: | Barnes & Noble |
Format: | eBook |
Pages: | 368 |
File size: | 5 MB |
About the Author
Toby J. Teorey is a professor in the Electrical Engineering and Computer Science Department at the University of Michigan, Ann Arbor. He received his B.S. and M.S. degrees in electrical engineering from the University of Arizona, Tucson, and a Ph.D. in computer sciences from the University of Wisconsin, Madison. He was general chair of the 1981 ACM SIGMOD Conference and program chair for the 1991 Entity-Relationship Conference. Professor Teorey’s current research focuses on database design and data warehousing, OLAP, advanced database systems, and performance of computer networks. He is a member of the ACM and the IEEE Computer Society.
Dr. Tony Morgan is a British computer scientist, data modeling consultant, and Professor in Computer Science at INTI International University, Malaysia. Dr. Morgan obtained his BA in Earth Sciences from The Open University, his BSc in Computer Systems Engineering from Coventry University, where in 1984 he also obtained his MSc in Control Engineering. In 1988 he obtained his PhD in Computer Science from University of Cambridge with a thesis on automated decision-making using qualitative reasoning. Dr. Morgan has done extensive work in industry with companies such as Unisys, EDS, and other corporations across transport, aerospace, government, and financial services, including the UK’s National Computing Centre in Manchester. Dr. Morgan has published several articles on AI and simulation. In 2003 he was appointed Professor of Computer Science and Vice President of Enterprise Informatics at Neumont University, Utah, USA. His research interests focus on business rules and business processes and the rapid development of high-quality information systems. Along with Dr. Halpin, he is the co-author of Information Modeling and Relational Databases, Second Edition, Elsevier/Morgan Kaufmann.
Graham C. Witt is an independent consultant with over 30 years of experience in assisting enterprises to acquire relevant and effective IT solutions. His clients include major banks and other financial institutions; businesses in the insurance, utilities, transport and telecommunications sectors; and a wide variety of government agencies. A former guest lecturer on Database Systems at University of Melbourne, he is a frequent presenter at international data management conferences.
Stephen Buxton is Director of Product Management at Mark Logic Corporation. Stephen is a member of the W3C XQuery Working Group and a founder/member of the XQuery Full-Text Task Force. Stephen has written a number of papers and articles on XQuery and SQL/XML, and is an editor of several W3C XQuery Full-Text specs. Before joining Mark Logic, Stephen was Director of Product Management for Text and XML at Oracle Corporation.
Lowell is responsible for directing thought leadership and advisory services in the Customer Success practice of Collibra. He has been a practitioner in the data management industry for three decades and is recognized as a leader in data governance, analytics and data quality having hands-on experience with implementations across most industries. Lowell is a co-author of the book “Business Metadata; Capturing Enterprise Knowledge. Lowell is a past adjunct professor at Daniels College of Business, Denver University, a past President and current VP of Education for DAMA-I Rocky Mountain Chapter (RMC), a DAMA-I Charter member and member of the Data Governance Professionals Organization. He is also an author and reviewer on the DAMA-I Data Management Book of Knowledge (DMBOK). He focuses on practical data governance practices and has trained thousands of professionals in data governance, data warehousing, data management and data quality techniques. You can read his Data Governance Blogs at https://www.collibra.com/blog/
Dr. Terry Halpin, is a Principal Scientist at LogicBlox, headquartered in Atlanta, USA, and a Professor at INTI International University, Malaysia. After many years in academia, he worked on data modeling technology at Asymetrix Corporation, InfoModelers Inc., Visio Corporation, and Microsoft Corporation, before returning to academia as Distinguished Professor at Neumont University (Utah, USA), and then once again returning to industry at LogicBlox and also taking a professorship at INTI. His research focuses on conceptual modeling and conceptual query technology. Dr. Halpin is the recipient of the DAMA International Academic Achievement Award and the IFIP Outstanding Service Award. He is a member of IFIP WG 8.1 (Design and Evaluation of Information Systems), is an editor or reviewer for several academic journals and international program committees, has co-chaired several international workshops on modeling, and has presented at dozens of international conferences in both industry and academia. For many years, his research has focused on conceptual modeling and conceptual query technology for information systems, using a business rules approach. His doctoral thesis formalized Object-Role Modeling (ORM/NIAM), and his publications include over 160 technical papers, and six books, including Information Modeling and Relational Databases, Second Edition, Elsevier/Morgan Kaufmann.
Jan L. Harrington, author of more than 35 books on a variety of technical subjects, has been writing about databases since 1984. She retired in 2013 from her position as professor and chair of the Department of Computing Technology at Marist College, where she taught database design and management, data communications, computer architecture, and the impact of technology on society for 25 years.
Best known as the “Father of Data Warehousing," Bill Inmon has become the most prolific and well-known author worldwide in the big data analysis, data warehousing and business intelligence arena. In addition to authoring more than 50 books and 650 articles, Bill has been a monthly columnist with the Business Intelligence Network, EIM Institute and Data Management Review. In 2007, Bill was named by Computerworld as one of the “Ten IT People Who Mattered in the Last 40 Years of the computer profession. Having 35 years of experience in database technology and data warehouse design, he is known globally for his seminars on developing data warehouses and information architectures. Bill has been a keynote speaker in demand for numerous computing associations, industry conferences and trade shows. Bill Inmon also has an extensive entrepreneurial background: He founded Pine Cone Systems, later named Ambeo in 1995, and founded, and took public, Prism Solutions in 1991. Bill consults with a large number of Fortune 1000 clients, and leading IT executives on Data Warehousing, Business Intelligence, and Database Management, offering data warehouse design and database management services, as well as producing methodologies and technologies that advance the enterprise architectures of large and small organizations world-wide. He has worked for American Management Systems and Coopers & Lybrand. Bill received his Bachelor of Science degree in Mathematics from Yale University, and his Master of Science degree in Computer Science from New Mexico State University.
Sam Lightstone is a Senior Technical Staff Member and Development Manager with IBM’s DB2 product development team. His work includes numerous topics in autonomic computing and relational database management systems. He is cofounder and leader of DB2’s autonomic computing R&D effort. He is Chair of the IEEE Data Engineering Workgroup on Self Managing Database Systems and a member of the IEEE Computer Society Task Force on Autonomous and Autonomic Computing. In 2003 he was elected to the Canadian Technical Excellence Council, the Canadian affiliate of the IBM Academy of Technology. He is an IBM Master Inventor with over 25 patents and patents pending; he has published widely on autonomic computing for relational database systems. He has been with IBM since 1991.
Jim Melton is editor of all parts of ISO/IEC 9075 (SQL) and is a representative for database standards at Oracle Corporation. Since 1986, he has been his company's representative to ANSI INCITS Technical Committee H2 for Database and a US representative to ISO/IEC JTC1/SC32/WG3 (Database Languages). In addition, Jim has participated in the W3C's XML Query Working Group since 1998 and is currently co-Chair of that Working Group. He is also Chair of the WG's Full-Text Task Force, co-Chair of the Update Language Task Force, and co-editor of two XQuery-related specifications. He is the author of several SQL books.
Read an Excerpt
Database Design
Know It AllBy Stephen Buxton Lowell Fryman Ralf Hartmut Güting Terry Halpin Jan L. Harrington William H. Inmon Sam S. Lightstone Jim Melton Tony Morgan Thomas P. Nadeau Bonnie O'Neil Elizabeth O'Neil Patrick O'Neil Markus Schneider Graeme Simsion Toby J. Teorey Graham Witt
MORGAN KAUFMANN
Copyright © 2009 Elsevier Inc.All right reserved.
ISBN: 978-0-08-087789-1
Chapter One
Introduction
Database technology has evolved rapidly in the three decades since the rise and eventual dominance of relational database systems. While many specialized database systems (object-oriented, spatial, multim, etc.) have found substantial user communities in the science and engineering fields, relational systems remain the dominant database technology for business enterprises.
Relational database design has evolved from an art to a science that has been made partially implementable as a set of software design aids. Many of these design aids have appeared as the database component of computer-aided software engineering (CASE) tools, and many of them offer interactive modeling capability using a simplified data modeling approach. Logical design—that is, the structure of basic data relationships and their definition in a particular database system—is largely the domain of application designers. These designers can work effectively with tools such as ERwin Data Modeler or Rational Rose with UML, as well as with a purely manual approach. Physical design, the creation of efficient data storage and retrieval mechanisms on the computing platform being used, is typically the domain of the database administrator (DBA). Today's DBAs have a variety of vendor-supplied tools available to help them design the most efficient databases. This book is devoted to the logical design methodologies and tools most popular for relational databases today. This chapter reviews the basic concepts of database management and introduce the role of data modeling and database design in the database life cycle.
1.1 DATA AND DATABASE MANAGEMENT
The basic component of a file in a file system is a data item, which is the smallest named unit of data that has meaning in the real world—for example, last name, first name, street address, ID number, or political party. A group of related data items treated as a single unit by an application is called a record. Examples of types of records are order, salesperson, customer, product, and department. A file is a collection of records of a single type. Database systems have built upon and expanded these definitions: In a relational database, a data item is called a column or attribute; a record is called a row or tuple; and a file is called a table.
A database is a more complex object. It is a collection of interrelated stored data—that is, interrelated collections of many different types of tables—that serves the needs of multiple users within one or more organizations. The motivations for using databases rather than files include greater availability to a diverse set of users, integration of data for easier access to and updating of complex transactions, and less redundancy of data.
A database management system (DBMS) is a generalized software system for manipulating databases. A DBMS supports a logical view (schema, subschema); physical view (access methods, data clustering); data definition language; data manipulation language; and important utilities, such as transaction management and concurrency control, data integrity, crash recovery, and security. Relational database systems, the dominant type of systems for well-formatted business databases, also provide a greater degree of data independence than the earlier hierarchical and network (CODASYL) database management systems. Data independence is the ability to make changes in either the logical or physical structure of the database without requiring reprogramming of application programs. It also makes database conversion and reorganization much easier. Relational DBMSs provide a much higher degree of data independence than previous systems; they are the focus of our discussion on data modeling.
1.2 THE DATABASE LIFE CYCLE
The database life cycle incorporates the basic steps involved in designing a global schema of the logical database, allocating data across a computer network, and defining local DBMS-specific schemas. Once the design is completed, the life cycle continues with database implementation and maintenance. This chapter contains an overview of the database life cycle, as shown in Figure 1.1. The result of each step of the life cycle is illustrated with a series of diagrams in Figure 1.2. Each diagram shows a possible form of the output of each step, so the reader can see the progression of the design process from an idea to actual database implementation.
I. Requirements analysis. The database requirements are determined by interviewing both the producers and users of data and using the information to produce a formal requirements specification. That specification includes the data required for processing, the natural data relationships, and the software platform for the database implementation. As an example, Figure 1.2 (step I) shows the concepts of products, customers, salespersons, and orders being formulated in the mind of the end user during the interview process.
II. Logical design. The global schema, a conceptual data model diagram that shows all the data and their relationships, is developed using techniques such as entity–relationship (ER) or UML. The data model constructs must ultimately be transformed into normalized (global) relations, or tables. The global schema development methodology is the same for either a distributed or centralized database.
a. Conceptual data modeling. The data requirements are analyzed and modeled using an ER or UML diagram that includes, for example, semantics for optional relationships, ternary relationships, supertypes, and subtypes (categories). Processing requirements are typically specified using natural language expressions or SQL commands, along with the frequency of occurrence. Figure 1.2 (step II(a)) shows a possible ER model representation of the product/customer database in the mind of the end user.
b. View integration. Usually, when the design is large and more than one person is involved in requirements analysis, multiple views of data and relationships result. To eliminate redundancy and inconsistency from the model, these views eventually must be "rationalized" (resolving inconsistencies due to variance in taxonomy, context, or perception) and then consolidated into a single global view. View integration requires the use of ER semantic tools such as identification of synonyms, aggregation, and generalization. In Figure 1.2 (step II(b)), two possible views of the product/ customer database are merged into a single global view based on common data for customer and order. View integration is also important for application integration.
c. Transformation of the conceptual data model to SQL tables. Based on a categorization of data modeling constructs and a set of mapping rules, each relationship and its associated entities are transformed into a set of DBMS-specific candidate relational tables. Redundant tables are eliminated as part of this process. In our example, the tables in step II(c) of Figure 1.2 are the result of transformation of the integrated ER model in step II(b).
d. Normalization of tables. Functional dependencies (FDs) are derived from the conceptual data model diagram and the semantics of data relationships in the requirements analysis. They represent the dependencies among data elements that are unique identifiers (keys) of entities. Additional FDs that represent the dependencies among key and nonkey attributes within entities can be derived from the requirements specification. Candidate relational tables associated with all derived FDs are normalized (i.e., modified by decomposing or splitting tables into smaller tables) using standard techniques. Finally, redundancies in the data in normalized candidate tables are analyzed further for possible elimination, with the constraint that data integrity must be preserved. An example of normalization of the Salesperson table into the new Salesperson and Sales-vacations tables is shown in Figure 1.2 from step II(c) to step II(d).
We note here that database tool vendors tend to use the term logical model to refer to the conceptual data model, and they use the term physical model to refer to the DBMS-specific implementation model (e.g., SQL tables). Note also that many conceptual data models are obtained not from scratch, but from the process of reverse engineering from an existing DBMS-specific schema (Silberschatz, Korth, & Sudarshan, 2002).
III. Physical design. The physical design step involves the selection of indexes (access methods), partitioning, and clustering of data. The logical design methodology in step II simplifies the approach to designing large relational databases by reducing the number of data dependencies that need to be analyzed. This is accomplished by inserting conceptual data modeling and integration steps (II(a) and II(b) of Figure 1.2) into the traditional relational design approach. The objective of these steps is an accurate representation of reality. Data integrity is preserved through normalization of the candidate tables created when the conceptual data model is transformed into a relational model. The purpose of physical design is to optimize performance as closely as possible.
As part of the physical design, the global schema can sometimes be refined in limited ways to reflect processing (query and transaction) requirements if there are obvious, large gains to be made in efficiency. This is called denormalization. It consists of selecting dominant processes on the basis of high frequency, high volume, or explicit priority; defining simple extensions to tables that will improve query performance; evaluating total cost for query, update, and storage; and considering the side effects, such as possible loss of integrity. This is particularly important for Online Analytical Processing (OLAP) applications.
IV. Database implementation, monitoring, and modification. Once the design is completed, the database can be created through implementation of the formal schema using the data definition language (DDL) of a DBMS. Then the data manipulation language (DML) can be used to query and update the database, as well as to set up indexes and establish constraints, such as referential integrity. The language SQL contains both DDL and DML constructs; for example, the create table command represents DDL, and the select command represents DML.
As the database begins operation, monitoring indicates whether performance requirements are being met. If they are not being satisfied, modifications should be made to improve performance. Other modifications may be necessary when requirements change or when the end users' expectations increase with good performance. Thus, the life cycle continues with monitoring, redesign, and modifications.
1.3 CONCEPTUAL DATA MODELING
Conceptual data modeling is the driving component of logical database design. Let us take a look at how this component came about, and why it is important. Schema diagrams were formalized in the 1960s by Charles Bachman. He used rectangles to denote record types and directed arrows from one record type to another to denote a one-to-many relationship among instances of records of the two types. The ER approach for conceptual data modeling was first presented in 1976 by Peter Chen. The Chen form of the ER model uses rectangles to specify entities, which are somewhat analogous to records. It also uses diamond-shaped objects to represent the various types of relationships, which are differentiated by numbers or letters placed on the lines connecting the diamonds to the rectangles.
The Unified Modeling Language (UML) was introduced in 1997 by Grady Booch and James Rumbaugh and has become a standard graphical language for specifying and documenting large-scale software systems. The data modeling component of UML (now UML 2.0) has a great deal of similarity with the ER model, and will be presented in detail in Chapter 3. We will use both the ER model and UML to illustrate the data modeling and logical database design examples.
(Continues...)
Excerpted from Database Design by Stephen Buxton Lowell Fryman Ralf Hartmut Güting Terry Halpin Jan L. Harrington William H. Inmon Sam S. Lightstone Jim Melton Tony Morgan Thomas P. Nadeau Bonnie O'Neil Elizabeth O'Neil Patrick O'Neil Markus Schneider Graeme Simsion Toby J. Teorey Graham Witt Copyright © 2009 by Elsevier Inc. . Excerpted by permission of MORGAN KAUFMANN. All rights reserved. No part of this excerpt may be reproduced or reprinted without permission in writing from the publisher.
Excerpts are provided by Dial-A-Book Inc. solely for the personal use of visitors to this web site.
Table of Contents
Chapter 1: The Database Life CycleChapter 2: Entity-Relationship ConceptsChapter 3: Data Modeling in UML Chapter 4: Requirements Analysis and Conceptual Data Modeling Chapter 5: Logical Database Design Chapter 6: Normalization Chapter 7: Physical Database Design Chapter 8: Denormalization Chapter 9: Business Metadata Infrastructure Chapter 10: Storing XML Chapter 11: Modeling and Querying Current MovementWhat People are Saying About This
All of the elements of database design together in a single volume written by the best and brightest experts in the field!