The book’s chief features are as follows: It highlights how deep neural networks can be used to address new questions and protocols, and to tackle current challenges in medical image computing; presents a comprehensive review of the latest research and literature; and describes a range of different methods that employ deep learning for object or landmark detection tasks in 2D and 3D medical imaging. In addition, the book examines a broad selection of techniques for semantic segmentation using deep learning principles in medical imaging; introduces a novel approach to text and image deep embedding for a large-scale chest x-ray image database; and discusses how deep learning relational graphs can be used to organize a sizable collection of radiology findings from real clinical practice, allowing semantic similarity-based retrieval.
The intended reader of this edited book is a professional engineer, scientist or a graduate student who is able to comprehend general concepts of image processing, computer vision and medical image analysis. They can apply computer science and mathematical principles into problem solving practices. It may be necessary to have a certain level of familiarity with a number of more advanced subjects: image formation and enhancement, image understanding, visual recognition in medical applications, statistical learning, deep neural networks, structured prediction and image segmentation.
The book’s chief features are as follows: It highlights how deep neural networks can be used to address new questions and protocols, and to tackle current challenges in medical image computing; presents a comprehensive review of the latest research and literature; and describes a range of different methods that employ deep learning for object or landmark detection tasks in 2D and 3D medical imaging. In addition, the book examines a broad selection of techniques for semantic segmentation using deep learning principles in medical imaging; introduces a novel approach to text and image deep embedding for a large-scale chest x-ray image database; and discusses how deep learning relational graphs can be used to organize a sizable collection of radiology findings from real clinical practice, allowing semantic similarity-based retrieval.
The intended reader of this edited book is a professional engineer, scientist or a graduate student who is able to comprehend general concepts of image processing, computer vision and medical image analysis. They can apply computer science and mathematical principles into problem solving practices. It may be necessary to have a certain level of familiarity with a number of more advanced subjects: image formation and enhancement, image understanding, visual recognition in medical applications, statistical learning, deep neural networks, structured prediction and image segmentation.
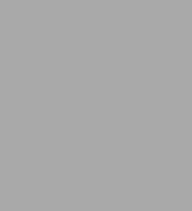
Deep Learning and Convolutional Neural Networks for Medical Imaging and Clinical Informatics
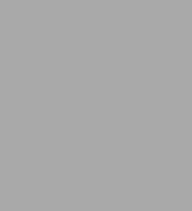
Deep Learning and Convolutional Neural Networks for Medical Imaging and Clinical Informatics
eBook(1st ed. 2019)
Related collections and offers
Product Details
ISBN-13: | 9783030139698 |
---|---|
Publisher: | Springer-Verlag New York, LLC |
Publication date: | 09/19/2019 |
Series: | Advances in Computer Vision and Pattern Recognition |
Sold by: | Barnes & Noble |
Format: | eBook |
File size: | 97 MB |
Note: | This product may take a few minutes to download. |