Deep Learning with Structured Data teaches you powerful data analysis techniques for tabular data and relational databases.
Summary
Deep learning offers the potential to identify complex patterns and relationships hidden in data of all sorts. Deep Learning with Structured Data shows you how to apply powerful deep learning analysis techniques to the kind of structured, tabular data you'll find in the relational databases that real-world businesses depend on. Filled with practical, relevant applications, this book teaches you how deep learning can augment your existing machine learning and business intelligence systems.
Purchase of the print book includes a free eBook in PDF, Kindle, and ePub formats from Manning Publications.
About the technology
Here’s a dirty secret: Half of the time in most data science projects is spent cleaning and preparing data. But there’s a better way: Deep learning techniques optimized for tabular data and relational databases deliver insights and analysis without requiring intense feature engineering. Learn the skills to unlock deep learning performance with much less data filtering, validating, and scrubbing.
About the book
Deep Learning with Structured Data teaches you powerful data analysis techniques for tabular data and relational databases. Get started using a dataset based on the Toronto transit system. As you work through the book, you’ll learn how easy it is to set up tabular data for deep learning, while solving crucial production concerns like deployment and performance monitoring.
What's inside
When and where to use deep learning
The architecture of a Keras deep learning model
Training, deploying, and maintaining models
Measuring performance
About the reader
For readers with intermediate Python and machine learning skills.
About the author
Mark Ryan is a Data Science Manager at Intact Insurance. He holds a Master's degree in Computer Science from the University of Toronto.
Table of Contents
1 Why deep learning with structured data?
2 Introduction to the example problem and Pandas dataframes
3 Preparing the data, part 1: Exploring and cleansing the data
4 Preparing the data, part 2: Transforming the data
5 Preparing and building the model
6 Training the model and running experiments
7 More experiments with the trained model
8 Deploying the model
9 Recommended next steps
1135022736
Summary
Deep learning offers the potential to identify complex patterns and relationships hidden in data of all sorts. Deep Learning with Structured Data shows you how to apply powerful deep learning analysis techniques to the kind of structured, tabular data you'll find in the relational databases that real-world businesses depend on. Filled with practical, relevant applications, this book teaches you how deep learning can augment your existing machine learning and business intelligence systems.
Purchase of the print book includes a free eBook in PDF, Kindle, and ePub formats from Manning Publications.
About the technology
Here’s a dirty secret: Half of the time in most data science projects is spent cleaning and preparing data. But there’s a better way: Deep learning techniques optimized for tabular data and relational databases deliver insights and analysis without requiring intense feature engineering. Learn the skills to unlock deep learning performance with much less data filtering, validating, and scrubbing.
About the book
Deep Learning with Structured Data teaches you powerful data analysis techniques for tabular data and relational databases. Get started using a dataset based on the Toronto transit system. As you work through the book, you’ll learn how easy it is to set up tabular data for deep learning, while solving crucial production concerns like deployment and performance monitoring.
What's inside
When and where to use deep learning
The architecture of a Keras deep learning model
Training, deploying, and maintaining models
Measuring performance
About the reader
For readers with intermediate Python and machine learning skills.
About the author
Mark Ryan is a Data Science Manager at Intact Insurance. He holds a Master's degree in Computer Science from the University of Toronto.
Table of Contents
1 Why deep learning with structured data?
2 Introduction to the example problem and Pandas dataframes
3 Preparing the data, part 1: Exploring and cleansing the data
4 Preparing the data, part 2: Transforming the data
5 Preparing and building the model
6 Training the model and running experiments
7 More experiments with the trained model
8 Deploying the model
9 Recommended next steps
Deep Learning with Structured Data
Deep Learning with Structured Data teaches you powerful data analysis techniques for tabular data and relational databases.
Summary
Deep learning offers the potential to identify complex patterns and relationships hidden in data of all sorts. Deep Learning with Structured Data shows you how to apply powerful deep learning analysis techniques to the kind of structured, tabular data you'll find in the relational databases that real-world businesses depend on. Filled with practical, relevant applications, this book teaches you how deep learning can augment your existing machine learning and business intelligence systems.
Purchase of the print book includes a free eBook in PDF, Kindle, and ePub formats from Manning Publications.
About the technology
Here’s a dirty secret: Half of the time in most data science projects is spent cleaning and preparing data. But there’s a better way: Deep learning techniques optimized for tabular data and relational databases deliver insights and analysis without requiring intense feature engineering. Learn the skills to unlock deep learning performance with much less data filtering, validating, and scrubbing.
About the book
Deep Learning with Structured Data teaches you powerful data analysis techniques for tabular data and relational databases. Get started using a dataset based on the Toronto transit system. As you work through the book, you’ll learn how easy it is to set up tabular data for deep learning, while solving crucial production concerns like deployment and performance monitoring.
What's inside
When and where to use deep learning
The architecture of a Keras deep learning model
Training, deploying, and maintaining models
Measuring performance
About the reader
For readers with intermediate Python and machine learning skills.
About the author
Mark Ryan is a Data Science Manager at Intact Insurance. He holds a Master's degree in Computer Science from the University of Toronto.
Table of Contents
1 Why deep learning with structured data?
2 Introduction to the example problem and Pandas dataframes
3 Preparing the data, part 1: Exploring and cleansing the data
4 Preparing the data, part 2: Transforming the data
5 Preparing and building the model
6 Training the model and running experiments
7 More experiments with the trained model
8 Deploying the model
9 Recommended next steps
Summary
Deep learning offers the potential to identify complex patterns and relationships hidden in data of all sorts. Deep Learning with Structured Data shows you how to apply powerful deep learning analysis techniques to the kind of structured, tabular data you'll find in the relational databases that real-world businesses depend on. Filled with practical, relevant applications, this book teaches you how deep learning can augment your existing machine learning and business intelligence systems.
Purchase of the print book includes a free eBook in PDF, Kindle, and ePub formats from Manning Publications.
About the technology
Here’s a dirty secret: Half of the time in most data science projects is spent cleaning and preparing data. But there’s a better way: Deep learning techniques optimized for tabular data and relational databases deliver insights and analysis without requiring intense feature engineering. Learn the skills to unlock deep learning performance with much less data filtering, validating, and scrubbing.
About the book
Deep Learning with Structured Data teaches you powerful data analysis techniques for tabular data and relational databases. Get started using a dataset based on the Toronto transit system. As you work through the book, you’ll learn how easy it is to set up tabular data for deep learning, while solving crucial production concerns like deployment and performance monitoring.
What's inside
When and where to use deep learning
The architecture of a Keras deep learning model
Training, deploying, and maintaining models
Measuring performance
About the reader
For readers with intermediate Python and machine learning skills.
About the author
Mark Ryan is a Data Science Manager at Intact Insurance. He holds a Master's degree in Computer Science from the University of Toronto.
Table of Contents
1 Why deep learning with structured data?
2 Introduction to the example problem and Pandas dataframes
3 Preparing the data, part 1: Exploring and cleansing the data
4 Preparing the data, part 2: Transforming the data
5 Preparing and building the model
6 Training the model and running experiments
7 More experiments with the trained model
8 Deploying the model
9 Recommended next steps
43.99
In Stock
5
1
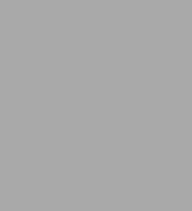
Deep Learning with Structured Data
264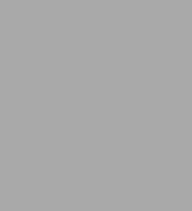
Deep Learning with Structured Data
264Related collections and offers
43.99
In Stock
From the B&N Reads Blog