Dynamic Flexible Constraint Satisfaction and its Application to AI Planning
First, I would like to thank my principal supervisor Dr Qiang Shen for all his help, advice and friendship throughout. Many thanks also to my second supervisor Dr Peter Jarvis for his enthusiasm, help and friendship. I would also like to thank the other members of the Approximate and Qualitative Reasoning group at Edinburgh who have also helped and inspired me. This project has been funded by an EPSRC studentship, award number 97305803. I would like, therefore, to extend my gratitude to EPSRC for supporting this work. Many thanks to the staff at Edinburgh University for all their help and support and for promptly fixing any technical problems that I have had . My whole family have been both encouraging and supportive throughout the completion of this book, for which I am forever indebted. York, April 2003 Ian Miguel Contents List of Figures XV 1 Introduction. . . . . . . . . . . . . . . . . . . . . . . . . . . . . . . . . . . . . . . . . . . . . . 1 1. 1 Solving Classical CSPs . . . . . . . . . . . . . . . . . . . . . . . . . . . . . . . . . . 2 1. 2 Applicat ions of Classical CSP . . . . . . . . . . . . . . . . . . . . . . . . . . . . 3 1. 3 Limitations of Classical CSP . . . . . . . . . . . . . . . . . . . . . . . . . . . . . 6 1. 3. 1 Flexible CSP 6 1. 3. 2 Dynamic CSP . . . . . . . . . . . . . . . . . . . . . . . . . . . . . . . . . . . . 7 1. 4 Dynamic Flexible CSP . . . . . . . . . . . . . . . . . . . . . . . . . . . . . . . . . . 7 1. 5 Flexible Planning: a DFCSP Application . . . . . . . . . . . . . . . . . . 8 1. 6 Structure . . . . . . . . . . . . . . . . . . . . . . . . . . . . . . . . . . . . . . . . . . . . . . 9 1. 7 Contributions and their Significance 11 2 The Constraint Satisfaction Problem 13 2. 1 Constraints and Constraint Graphs . . . . . . . . .. . . . . . . . . . . . . . 13 2. 2 Tree Search Solution Techniques for Classical CSP . . . . . . . . . . 16 2. 2. 1 Backtrack . . . . . . . . . . . . . . . . . . . . . . . . . . . . . . . . . . . . . . . 17 2. 2. 2 Backjumping . . . . . . . . . . . . . . . . . . . . . . . . . . . . . . . . . . . . 18 2. 2. 3 Conflict-Directed Backjumping . . . . . . . . . . . . . . . . . . . . . 19 2. 2. 4 Backmarking . . . . . . . . . . . . . . . . . . . . . . . . . . . . . . . . . . . .
1101512831
Dynamic Flexible Constraint Satisfaction and its Application to AI Planning
First, I would like to thank my principal supervisor Dr Qiang Shen for all his help, advice and friendship throughout. Many thanks also to my second supervisor Dr Peter Jarvis for his enthusiasm, help and friendship. I would also like to thank the other members of the Approximate and Qualitative Reasoning group at Edinburgh who have also helped and inspired me. This project has been funded by an EPSRC studentship, award number 97305803. I would like, therefore, to extend my gratitude to EPSRC for supporting this work. Many thanks to the staff at Edinburgh University for all their help and support and for promptly fixing any technical problems that I have had . My whole family have been both encouraging and supportive throughout the completion of this book, for which I am forever indebted. York, April 2003 Ian Miguel Contents List of Figures XV 1 Introduction. . . . . . . . . . . . . . . . . . . . . . . . . . . . . . . . . . . . . . . . . . . . . . 1 1. 1 Solving Classical CSPs . . . . . . . . . . . . . . . . . . . . . . . . . . . . . . . . . . 2 1. 2 Applicat ions of Classical CSP . . . . . . . . . . . . . . . . . . . . . . . . . . . . 3 1. 3 Limitations of Classical CSP . . . . . . . . . . . . . . . . . . . . . . . . . . . . . 6 1. 3. 1 Flexible CSP 6 1. 3. 2 Dynamic CSP . . . . . . . . . . . . . . . . . . . . . . . . . . . . . . . . . . . . 7 1. 4 Dynamic Flexible CSP . . . . . . . . . . . . . . . . . . . . . . . . . . . . . . . . . . 7 1. 5 Flexible Planning: a DFCSP Application . . . . . . . . . . . . . . . . . . 8 1. 6 Structure . . . . . . . . . . . . . . . . . . . . . . . . . . . . . . . . . . . . . . . . . . . . . . 9 1. 7 Contributions and their Significance 11 2 The Constraint Satisfaction Problem 13 2. 1 Constraints and Constraint Graphs . . . . . . . . .. . . . . . . . . . . . . . 13 2. 2 Tree Search Solution Techniques for Classical CSP . . . . . . . . . . 16 2. 2. 1 Backtrack . . . . . . . . . . . . . . . . . . . . . . . . . . . . . . . . . . . . . . . 17 2. 2. 2 Backjumping . . . . . . . . . . . . . . . . . . . . . . . . . . . . . . . . . . . . 18 2. 2. 3 Conflict-Directed Backjumping . . . . . . . . . . . . . . . . . . . . . 19 2. 2. 4 Backmarking . . . . . . . . . . . . . . . . . . . . . . . . . . . . . . . . . . . .
109.99
In Stock
5
1
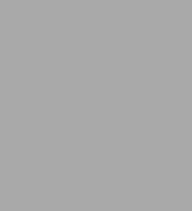
Dynamic Flexible Constraint Satisfaction and its Application to AI Planning
318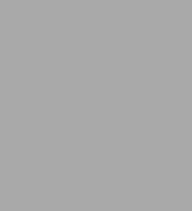
Dynamic Flexible Constraint Satisfaction and its Application to AI Planning
318Hardcover(2004)
$109.99
109.99
In Stock
Product Details
ISBN-13: | 9781852337643 |
---|---|
Publisher: | Springer London |
Publication date: | 10/21/2003 |
Series: | Distinguished Dissertations |
Edition description: | 2004 |
Pages: | 318 |
Product dimensions: | 6.10(w) x 9.25(h) x 0.36(d) |
From the B&N Reads Blog