Designed for the applied practitioner, this book is a compact, entry-level guide to modeling and analyzing non-Gaussian and correlated data. Many practitioners work with data that fail the assumptions of the common linear regression models, necessitating more advanced modeling techniques. This Handbook presents clearly explained modeling options for such situations, along with extensive example data analyses. The book explains core models such as logistic regression, count regression, longitudinal regression, survival analysis, and structural equation modelling without relying on mathematical derivations. All data analyses are performed on real and publicly available data sets, which are revisited multiple times to show differing results using various modeling options. Common pitfalls, data issues, and interpretation of model results are also addressed. Programs in both R and SAS are made available for all results presented in the text so that readers can emulate and adapt analyses for their own data analysis needs. Data, R, and SAS scripts can be found online at http://www.spesi.org.
1133678455
Handbook for Applied Modeling: Non-Gaussian and Correlated Data
Designed for the applied practitioner, this book is a compact, entry-level guide to modeling and analyzing non-Gaussian and correlated data. Many practitioners work with data that fail the assumptions of the common linear regression models, necessitating more advanced modeling techniques. This Handbook presents clearly explained modeling options for such situations, along with extensive example data analyses. The book explains core models such as logistic regression, count regression, longitudinal regression, survival analysis, and structural equation modelling without relying on mathematical derivations. All data analyses are performed on real and publicly available data sets, which are revisited multiple times to show differing results using various modeling options. Common pitfalls, data issues, and interpretation of model results are also addressed. Programs in both R and SAS are made available for all results presented in the text so that readers can emulate and adapt analyses for their own data analysis needs. Data, R, and SAS scripts can be found online at http://www.spesi.org.
47.99
In Stock
5
1
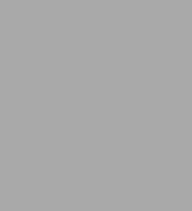
Handbook for Applied Modeling: Non-Gaussian and Correlated Data
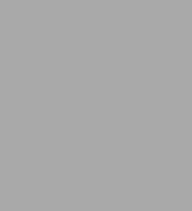
Handbook for Applied Modeling: Non-Gaussian and Correlated Data
Related collections and offers
47.99
In Stock
Product Details
ISBN-13: | 9781108206914 |
---|---|
Publisher: | Cambridge University Press |
Publication date: | 07/14/2017 |
Sold by: | Barnes & Noble |
Format: | eBook |
File size: | 4 MB |
About the Author
From the B&N Reads Blog