Machine Learning and Knowledge Discovery in Databases: European Conference, ECML PKDD 2009, Bled, Slovenia, September 7-11, 2009, Proceedings, Part I
This book constitutes the refereed proceedings of the joint conference on Machine Learning and Knowledge Discovery in Databases: ECML PKDD 2009, held in Bled, Slovenia, in September 2009. The 106 papers presented in two volumes, together with 5 invited talks, were carefully reviewed and selected from 422 paper submissions. In addition to the regular papers the volume contains 14 abstracts of papers appearing in full version in the Machine Learning Journal and the Knowledge Discovery and Databases Journal of Springer. The conference intends to provide an international forum for the discussion of the latest high quality research results in all areas related to machine learning and knowledge discovery in databases. The topics addressed are application of machine learning and data mining methods to real-world problems, particularly exploratory research that describes novel learning and mining tasks and applications requiring non-standard techniques.
1114171029
Machine Learning and Knowledge Discovery in Databases: European Conference, ECML PKDD 2009, Bled, Slovenia, September 7-11, 2009, Proceedings, Part I
This book constitutes the refereed proceedings of the joint conference on Machine Learning and Knowledge Discovery in Databases: ECML PKDD 2009, held in Bled, Slovenia, in September 2009. The 106 papers presented in two volumes, together with 5 invited talks, were carefully reviewed and selected from 422 paper submissions. In addition to the regular papers the volume contains 14 abstracts of papers appearing in full version in the Machine Learning Journal and the Knowledge Discovery and Databases Journal of Springer. The conference intends to provide an international forum for the discussion of the latest high quality research results in all areas related to machine learning and knowledge discovery in databases. The topics addressed are application of machine learning and data mining methods to real-world problems, particularly exploratory research that describes novel learning and mining tasks and applications requiring non-standard techniques.
109.99
In Stock
5
1
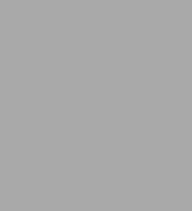
Machine Learning and Knowledge Discovery in Databases: European Conference, ECML PKDD 2009, Bled, Slovenia, September 7-11, 2009, Proceedings, Part I
756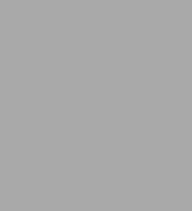
Machine Learning and Knowledge Discovery in Databases: European Conference, ECML PKDD 2009, Bled, Slovenia, September 7-11, 2009, Proceedings, Part I
756Paperback(2009)
$109.99
109.99
In Stock
Product Details
ISBN-13: | 9783642041792 |
---|---|
Publisher: | Springer Berlin Heidelberg |
Publication date: | 10/07/2009 |
Series: | Lecture Notes in Computer Science , #5781 |
Edition description: | 2009 |
Pages: | 756 |
Product dimensions: | 6.10(w) x 9.10(h) x 1.20(d) |
From the B&N Reads Blog