Measuring Data Quality for Ongoing Improvement: A Data Quality Assessment Framework
The Data Quality Assessment Framework shows you how to measure and monitor data quality, ensuring quality over time. You'll start with general concepts of measurement and work your way through a detailed framework of more than three dozen measurement types related to five objective dimensions of quality: completeness, timeliness, consistency, validity, and integrity. Ongoing measurement, rather than one time activities will help your organization reach a new level of data quality. This plain-language approach to measuring data can be understood by both business and IT and provides practical guidance on how to apply the DQAF within any organization enabling you to prioritize measurements and effectively report on results. Strategies for using data measurement to govern and improve the quality of data and guidelines for applying the framework within a data asset are included. You'll come away able to prioritize which measurement types to implement, knowing where to place them in a data flow and how frequently to measure. Common conceptual models for defining and storing of data quality results for purposes of trend analysis are also included as well as generic business requirements for ongoing measuring and monitoring including calculations and comparisons that make the measurements meaningful and help understand trends and detect anomalies. - Demonstrates how to leverage a technology independent data quality measurement framework for your specific business priorities and data quality challenges - Enables discussions between business and IT with a non-technical vocabulary for data quality measurement - Describes how to measure data quality on an ongoing basis with generic measurement types that can be applied to any situation
1118726836
Measuring Data Quality for Ongoing Improvement: A Data Quality Assessment Framework
The Data Quality Assessment Framework shows you how to measure and monitor data quality, ensuring quality over time. You'll start with general concepts of measurement and work your way through a detailed framework of more than three dozen measurement types related to five objective dimensions of quality: completeness, timeliness, consistency, validity, and integrity. Ongoing measurement, rather than one time activities will help your organization reach a new level of data quality. This plain-language approach to measuring data can be understood by both business and IT and provides practical guidance on how to apply the DQAF within any organization enabling you to prioritize measurements and effectively report on results. Strategies for using data measurement to govern and improve the quality of data and guidelines for applying the framework within a data asset are included. You'll come away able to prioritize which measurement types to implement, knowing where to place them in a data flow and how frequently to measure. Common conceptual models for defining and storing of data quality results for purposes of trend analysis are also included as well as generic business requirements for ongoing measuring and monitoring including calculations and comparisons that make the measurements meaningful and help understand trends and detect anomalies. - Demonstrates how to leverage a technology independent data quality measurement framework for your specific business priorities and data quality challenges - Enables discussions between business and IT with a non-technical vocabulary for data quality measurement - Describes how to measure data quality on an ongoing basis with generic measurement types that can be applied to any situation
49.95
In Stock
5
1
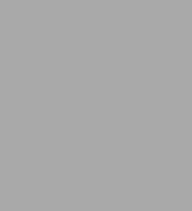
Measuring Data Quality for Ongoing Improvement: A Data Quality Assessment Framework
376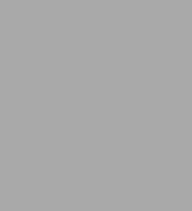
Measuring Data Quality for Ongoing Improvement: A Data Quality Assessment Framework
376
49.95
In Stock
Product Details
ISBN-13: | 9780123977540 |
---|---|
Publisher: | Morgan Kaufmann Publishers |
Publication date: | 12/31/2012 |
Series: | The Morgan Kaufmann Series on Business Intelligence |
Sold by: | Barnes & Noble |
Format: | eBook |
Pages: | 376 |
File size: | 6 MB |
About the Author
What People are Saying About This
From the B&N Reads Blog