The book consists of two parts: Pattern Classification and Function Approximation. In the first part, based on the synthesis principle of the neural-network classifier: A new learning paradigm is discussed and classification performance and training time of the new paradigm for several real-world data sets are compared with those of the widely-used back-propagation algorithm; Fuzzy classifiers of different architectures based on fuzzy rules can be defined with hyperbox, polyhedral, or ellipsoidal regions. The book discusses the unified approach for training these fuzzy classifiers; The performance of the newly-developed fuzzy classifiers and the conventional classifiers such as nearest-neighbor classifiers and support vector machines are evaluated using several real-world data sets and their advantages and disadvantages are clarified.
In the second part: Function approximation is discussed extending the discussions in the first part; Performance of the function approximators is compared.
This book is aimed primarily at researchers and practitioners in the field of artificial intelligence and neural networks.
The book consists of two parts: Pattern Classification and Function Approximation. In the first part, based on the synthesis principle of the neural-network classifier: A new learning paradigm is discussed and classification performance and training time of the new paradigm for several real-world data sets are compared with those of the widely-used back-propagation algorithm; Fuzzy classifiers of different architectures based on fuzzy rules can be defined with hyperbox, polyhedral, or ellipsoidal regions. The book discusses the unified approach for training these fuzzy classifiers; The performance of the newly-developed fuzzy classifiers and the conventional classifiers such as nearest-neighbor classifiers and support vector machines are evaluated using several real-world data sets and their advantages and disadvantages are clarified.
In the second part: Function approximation is discussed extending the discussions in the first part; Performance of the function approximators is compared.
This book is aimed primarily at researchers and practitioners in the field of artificial intelligence and neural networks.
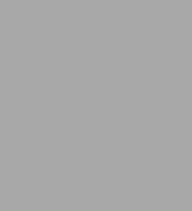
Pattern Classification: Neuro-fuzzy Methods and Their Comparison
327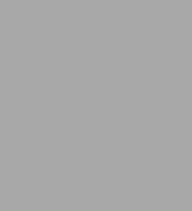
Pattern Classification: Neuro-fuzzy Methods and Their Comparison
327Paperback(Softcover reprint of the original 1st ed. 2001)
Product Details
ISBN-13: | 9781447110774 |
---|---|
Publisher: | Springer London |
Publication date: | 10/04/2012 |
Edition description: | Softcover reprint of the original 1st ed. 2001 |
Pages: | 327 |
Product dimensions: | 6.10(w) x 9.25(h) x 0.03(d) |