Planning, Proposing, and Presenting Science Effectively: A Guide for Graduate Students and Researchers in the Behavioral Sciences and Biology
This concise guide to planning, writing, and presenting research is intended for biology students of all levels, especially those in behavioral ecology, The reader is guided through a discussion of the nature of scientific research, how to plan research, and how to obtain funding. The authors give advice and guidelines for presenting results at research seminars and scientific meetings, and also provide useful tips on preparing abstracts and posters for scientific meetings. They discuss how to write an effective C.V. and give general tips on how to write clearly. The book is illuminated throughout with personal examples from the authors' own experiences and emphasis is placed on problems associated with field studies. All biologists will find this a valuable resource and guide for the early years of their scientific careers and established faculty will find it an essential instructional tool.
1100940002
Planning, Proposing, and Presenting Science Effectively: A Guide for Graduate Students and Researchers in the Behavioral Sciences and Biology
This concise guide to planning, writing, and presenting research is intended for biology students of all levels, especially those in behavioral ecology, The reader is guided through a discussion of the nature of scientific research, how to plan research, and how to obtain funding. The authors give advice and guidelines for presenting results at research seminars and scientific meetings, and also provide useful tips on preparing abstracts and posters for scientific meetings. They discuss how to write an effective C.V. and give general tips on how to write clearly. The book is illuminated throughout with personal examples from the authors' own experiences and emphasis is placed on problems associated with field studies. All biologists will find this a valuable resource and guide for the early years of their scientific careers and established faculty will find it an essential instructional tool.
53.0
In Stock
5
1
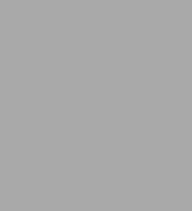
Planning, Proposing, and Presenting Science Effectively: A Guide for Graduate Students and Researchers in the Behavioral Sciences and Biology
248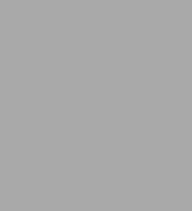
Planning, Proposing, and Presenting Science Effectively: A Guide for Graduate Students and Researchers in the Behavioral Sciences and Biology
248Paperback(Revised)
$53.00
53.0
In Stock
Product Details
ISBN-13: | 9780521533881 |
---|---|
Publisher: | Cambridge University Press |
Publication date: | 11/02/2006 |
Edition description: | Revised |
Pages: | 248 |
Product dimensions: | 5.98(w) x 9.02(h) x 0.51(d) |
About the Author
From the B&N Reads Blog