Implement scikit-learn into every step of the data science pipeline
About This Book- Use Python and scikit-learn to create intelligent applications
- Discover how to apply algorithms in a variety of situations to tackle common and not-so common challenges in the machine learning domain
- A practical, example-based guide to help you gain expertise in implementing and evaluating machine learning systems using scikit-learn
If you are a programmer and want to explore machine learning and data-based methods to build intelligent applications and enhance your programming skills, this is the course for you. No previous experience with machine-learning algorithms is required.
What You Will Learn- Review fundamental concepts including supervised and unsupervised experiences, common tasks, and performance metrics
- Classify objects (from documents to human faces and flower species) based on some of their features, using a variety of methods from Support Vector Machines to Naive Bayes
- Use Decision Trees to explain the main causes of certain phenomena such as passenger survival on the Titanic
- Evaluate the performance of machine learning systems in common tasks
- Master algorithms of various levels of complexity and learn how to analyze data at the same time
- Learn just enough math to think about the connections between various algorithms
- Customize machine learning algorithms to fit your problem, and learn how to modify them when the situation calls for it
- Incorporate other packages from the Python ecosystem to munge and visualize your dataset
- Improve the way you build your models using parallelization techniques
Machine learning, the art of creating applications that learn from experience and data, has been around for many years. Python is quickly becoming the go-to language for analysts and data scientists due to its simplicity and flexibility; moreover, within the Python data space, scikit-learn is the unequivocal choice for machine learning. The course combines an introduction to some of the main concepts and methods in machine learning with practical, hands-on examples of real-world problems. The course starts by walking through different methods to prepare your data—be it a dataset with missing values or text columns that require the categories to be turned into indicator variables. After the data is ready, you'll learn different techniques aligned with different objectives—be it a dataset with known outcomes such as sales by state, or more complicated problems such as clustering similar customers. Finally, you'll learn how to polish your algorithm to ensure that it's both accurate and resilient to new datasets. You will learn to incorporate machine learning in your applications. Ranging from handwritten digit recognition to document classification, examples are solved step-by-step using scikit-learn and Python. By the end of this course you will have learned how to build applications that learn from experience, by applying the main concepts and techniques of machine learning.
Style and ApproachImplement scikit-learn using engaging examples and fun exercises, and with a gentle and friendly but comprehensive "learn-by-doing" approach. This is a practical course, which analyzes compelling data about life, health, and death with the help of tutorials. It offers you a useful way of interpreting the data that's specific to this course, but that can also be applied to any other data. This course is designed to be both a guide and a reference for moving beyond the basics of scikit-learn.
Implement scikit-learn into every step of the data science pipeline
About This Book- Use Python and scikit-learn to create intelligent applications
- Discover how to apply algorithms in a variety of situations to tackle common and not-so common challenges in the machine learning domain
- A practical, example-based guide to help you gain expertise in implementing and evaluating machine learning systems using scikit-learn
If you are a programmer and want to explore machine learning and data-based methods to build intelligent applications and enhance your programming skills, this is the course for you. No previous experience with machine-learning algorithms is required.
What You Will Learn- Review fundamental concepts including supervised and unsupervised experiences, common tasks, and performance metrics
- Classify objects (from documents to human faces and flower species) based on some of their features, using a variety of methods from Support Vector Machines to Naive Bayes
- Use Decision Trees to explain the main causes of certain phenomena such as passenger survival on the Titanic
- Evaluate the performance of machine learning systems in common tasks
- Master algorithms of various levels of complexity and learn how to analyze data at the same time
- Learn just enough math to think about the connections between various algorithms
- Customize machine learning algorithms to fit your problem, and learn how to modify them when the situation calls for it
- Incorporate other packages from the Python ecosystem to munge and visualize your dataset
- Improve the way you build your models using parallelization techniques
Machine learning, the art of creating applications that learn from experience and data, has been around for many years. Python is quickly becoming the go-to language for analysts and data scientists due to its simplicity and flexibility; moreover, within the Python data space, scikit-learn is the unequivocal choice for machine learning. The course combines an introduction to some of the main concepts and methods in machine learning with practical, hands-on examples of real-world problems. The course starts by walking through different methods to prepare your data—be it a dataset with missing values or text columns that require the categories to be turned into indicator variables. After the data is ready, you'll learn different techniques aligned with different objectives—be it a dataset with known outcomes such as sales by state, or more complicated problems such as clustering similar customers. Finally, you'll learn how to polish your algorithm to ensure that it's both accurate and resilient to new datasets. You will learn to incorporate machine learning in your applications. Ranging from handwritten digit recognition to document classification, examples are solved step-by-step using scikit-learn and Python. By the end of this course you will have learned how to build applications that learn from experience, by applying the main concepts and techniques of machine learning.
Style and ApproachImplement scikit-learn using engaging examples and fun exercises, and with a gentle and friendly but comprehensive "learn-by-doing" approach. This is a practical course, which analyzes compelling data about life, health, and death with the help of tutorials. It offers you a useful way of interpreting the data that's specific to this course, but that can also be applied to any other data. This course is designed to be both a guide and a reference for moving beyond the basics of scikit-learn.
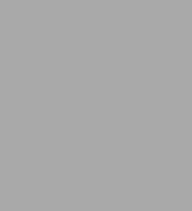
scikit-learn : Machine Learning Simplified
530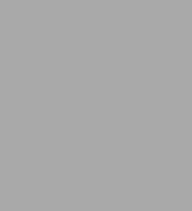
scikit-learn : Machine Learning Simplified
530Product Details
ISBN-13: | 9781788831529 |
---|---|
Publisher: | Packt Publishing |
Publication date: | 11/10/2017 |
Sold by: | Barnes & Noble |
Format: | eBook |
Pages: | 530 |
File size: | 11 MB |
Note: | This product may take a few minutes to download. |