Statistics, Econometrics and Forecasting available in Hardcover, Paperback
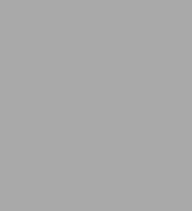
- ISBN-10:
- 0521540445
- ISBN-13:
- 9780521540445
- Pub. Date:
- 02/19/2004
- Publisher:
- Cambridge University Press
- ISBN-10:
- 0521540445
- ISBN-13:
- 9780521540445
- Pub. Date:
- 02/19/2004
- Publisher:
- Cambridge University Press
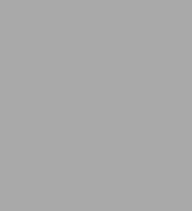
Buy New
$41.99Buy Used
$27.38-
-
SHIP THIS ITEM
Temporarily Out of Stock Online
Please check back later for updated availability.
-
Overview
Product Details
ISBN-13: | 9780521540445 |
---|---|
Publisher: | Cambridge University Press |
Publication date: | 02/19/2004 |
Series: | The Stone Lectures in Economics |
Edition description: | New Edition |
Pages: | 184 |
Product dimensions: | 5.47(w) x 8.54(h) x 0.55(d) |
About the Author
Read an Excerpt
Cambridge University Press
052183287X - Statistics, Econometrics and Forecasting - by Arnold Zellner
Excerpt
LECTURE 1 Bank of England
MAY 8, 2001
1.1 Introduction
There can be no doubt but that Sir Richard Stone is a true economic scientist, one of those who contributed importantly to the transition of economics from being an art to being a science. Significantly, he emphasized and practiced "measurement with theory," not "measurement without theory" nor "theory without measurement." In doing so, he set an excellent example for many others who followed his lead. His careful and thorough measurement procedures and use of sophisticatedly simple theoretical economic models and impressive statistical techniques in analyses of important problems brought him worldwide recognition, as recognized by many, including Angus Deaton1 in the following words:
Sir Richard Stone, knighted in 1978, and Nobel Laureate in Economics in 1984, is the outstanding figure in post-war British applied economics . . . Under Keynes' stimulus, the Cambridge Department of Applied Economics was founded and Richard Stone was appointed its first director with an indefinite tenure in the position. Stone brought enormous distinction and worldwide recognition to the department . . . He was president of the Econometric Society in 1955 and president of the Royal Economic Society from 1978-90.
Further, in an article, "The life and work of John Richard Nicholas Stone 1913-1991," that appeared in the Economic Journal, M. H. Pesaran and G. C. Harcourt (2000, p. 146) wrote:
Sir Richard Stone . . . was one of the pioneers of national income and social accounts, and one of the few economists of his generation to have faced the challenge of economics as a science by combining theory and measurement within a cohesive framework. Awarded the Nobel Prize for his 'fundamental contributions to the development of national accounts', he made equally significant contributions to the empirical analysis of consumer behaviour. His work on the 'Growth Project' was instrumental in the development of econometric methodology for the construction and analysis of large disaggregated macroeconometric models.
Also, they presented the following excerpt from Stone's research proposal for the now famous Department of Applied Economics at Cambridge with Stone as its first director in 1945 (pp. 149-150):
The ultimate aim of applied economics is to increase human welfare by the investigation and analysis of economic problems of the real world. It is the view of the Department that this can best be achieved by the synthesis of three types of study which now tend to be pursued in isolation. The Department will concentrate simultaneously on the work of observations, i.e. the discovery and preparation of data; the theoretical appraisal of problems, i.e. the framing of hypotheses in a form suitable for quantitative testing; and the development of statistical methods appropriate to the special problems of economic information. The special character of the Department's approach to problems of the real world will lie in this attempt at systematic synthesis.
From what has been presented above, it is clear that Stone had a deep appreciation of methodological issues and an approach that was very productive. Note that Pearson, Jeffreys, Fisher, I2 and many others are in broad agreement with Stone's position and have emphasized the "unity of science" principle, namely that any area of study (e.g., economics, physics, business, psychology, sports, etc.) can be a science if scientific methods are employed in learning from data and experience to explain the past, predict and make wise decisions - fundamental objectives of science. To achieve these objectives, scientists use methods that will now briefly be reviewed.
It has been recognized that scientists generally employ the process of induction, which involves (a) measurement and description and (b) use of generalizations or theories to explain, predict and make decisions. This view of induction is much broader than that of Mach's, which involves equating induction to empirical measurement. In doing so, Mach missed the very important activities of explanation, prediction and decision-making that are involved in the above, broader definition of induction. Similarly, attempts by others to equate science, particularly economic science, to deduction is a fundamental mistake since in deduction just limiting statements of proof, disproof or ignorance are possible. Scientists need and use statements reflecting degrees of confidence in propositions or generalizations that cannot be analyzed using only deductive methods. For example, it is impossible to prove deductively that the sun will rise tomorrow. This point is very important with respect to those who, in contrast to Stone and many others, hold the view that economics is a purely deductive science. Deduction, including mathematical proof, plays a role but the broader process of induction is needed in science. Later I shall discuss how probabilities to represent degrees of confidence in propositions or theories can be utilized in the process of learning from data and making decisions. Finally, there is the area of "reduction," in which work is undertaken to produce generalizations or theories that explain the past, predict well and are useful in making decisions; this will be discussed below.
A key element in the inductive process is measurement and description, as Stone, Jeffreys, Fisher and others have recognized. As widely appreciated, it is important to measure well important variables such as unemployment, output, prices, income, saving, etc. for a variety of purposes. Fortunately, much progress over the years has been made in improving the quality of measurements, e.g. quality-corrected price indices, consistent national income and product accounts, etc. However, many other improvements can be made, e.g. in the measurement of the output of government and education sectors and of personal saving. Such measurements are important inputs to those who study economies' past performance and attempt to forecast future outcomes- that is, professional and amateur forecasters. Also, these measurements are important inputs to those engaged in the process of "reduction" - that is, creation of theories to explain past data and help to predict as yet unobserved data. For example, the famous Kuznets research finding that the US savings rate was relatively constant over the first half of the twentieth century in spite of huge increases in real income was a surprising empirical result, in sharp contradiction to the Keynesian prediction that the savings rate would rise.3 Several, including Friedman, Modigliani, Tobin and others, created new theories to explain the surprising empirically observed constancy of the savings rate. Further, as Hadamard4 reported in his study of creative work in mathematics, new breakthroughs in mathematics and other fields are often produced after observing "unusual facts." In view of this connection between productive reductive activity and unusual facts, in past work I have described5 a number of ways to produce unusual facts: e.g., study unusual historical periods - say, periods of hyperinflation or great depression; study unusual groups, e.g. very poor producers and consumers; push current theories to extremes and empirically check their predictions, etc. Also, my advice to empirical workers in economics is: produce unusual facts that need explanation and ugly facts (which Thomas Huxley emphasized as being important, namely facts that sharply contradict current theories), instead of humdrum, boring facts.
Above, I mentioned that forecasters are vitally interested in inductive measurement problems and require good data with which to develop effective statistical forecasting procedures and models. For many years, forecasting models, e.g. the univariate autoregressive moving average (ARMA) forecasting models of Box and Jenkins and the multivariate ARMA models of Quenouille,6 which include a vector autoregressive (VAR) model as a special case, were considered to be distinct from the structural econometric models (SEMs) constructed by economists such as Tinbergen, Klein, Stone and many others. In a 1974 paper,7 Palm and I not only demonstrated the relationship between univariate and multivariate ARMA models and structural econometric models but also illustrated how that relationship can be exploited to produce improved SEMs in the structural econometric, time series analysis approach. This combination of forecasting and structural modeling approaches has been very fruitful and will be illustrated below.
In addition, I, along with many others, have emphasized the importance of sophisticated simplicity in modeling. Note that, in industry, there is the expression KISS: that is, "Keep It Simple, Stupid." Since some simple models are stupid, I decided to reinterpret KISS to mean: "Keep It Sophisticatedly Simple." Indeed, there are many sophisticatedly simple models that work reasonably well in many sciences, e.g. s = ½gt2, F = ma, PV = RT, E = mc2, the laws of demand and supply, etc. Over the years I have challenged many audiences to tell me about one complicated model that works well in explanation and prediction and have not heard of a single one. Certainly, large-scale econometric models, VARs and other complex models have not worked very well in explanation and prediction in economics. For evidence on these points, see, e.g., McNees, Zarnowitz, Fair, and Fisher and Whitley.8
Further, after years of application, the "general to specific" approach that involves starting with a complicated large model, often a linear VAR, and testing downward to obtain a good model has not as yet been successful. There are many, many general models and if one chooses the wrong one, as is usually the case, results are doomed to be unsatisfactory. Starting simply and complicating if necessary is an approach that has worked well in many sciences and is favored by Jeffreys, Friedman, Tobin and many others. For further consideration of these issues of simplicity versus complexity and methods of measuring the simplicity of economic models, see the papers in the recent Cambridge University Press monograph, Simplicity, Inference and Modeling.9
With this said about some key philosophical issues involved in statistics, econometrics and forecasting, we now come to the fundamental Bayes/non-Bayes statistical/econometric methodological controversies that have been raging since the publication of Bayes' 1763 paper.10 These controversies are focused on the issues of (1) how to learn from data effectively, (2) how to estimate effects and test for their presence or absence, (3) how to use data to make good forecasts and decisions, and (4) how to produce models or laws that work well in explanation, prediction and decision-making. Many leading workers worldwide, including Laplace, Edgeworth, Jeffreys, Fisher, Neyman, Pearson, de Finetti, Savage, Box, Lindley, Good and many others, have been involved in these controversies, which are still ongoing. What is at issue in such debates and discussions is, fundamentally: "how do we operationally perform scientific inference - that is, induction and reduction- that involves effective learning from data, making wise decisions, and producing good models or laws that are helpful in explanation, prediction and decision-making?" It is my view that the Bayesian approach is emerging as the principal paradigm for use in science and decision-making. For recent information on the explosive upward movement in the volume of Bayesian publications with many references, some to free downloadable Bayesian computer software, including the University of Cambridge's famous "BUGS" program, see Berger's December 2000 Journal of the American Statistical Association article and material and references on the homepages of the International Society for Bayesian Analysis website, and of the Section on Bayesian Statistical Science (SBSS) of the American Statistical Association (ASA) website. Also, the annual SBSS/ISBA proceedings volumes contain many valuable articles in which Bayesian methods are developed and applied to forecasting, financial portfolio and other problems. Then, too, the recent proceedings volume for the ISBA 2000 world meeting, which was published in 2001 by Eurostat (the statistical office of the European Communities),11 includes Bayesian papers dealing with many basic theoretical and applied problems.
1.2 Overview of the Bayesian approach
Basic to the Bayesian approach is the Bayesian learning model, Bayes' theorem, which has been applied successfully to a wide range of problems encountered in statistics, econometrics, forecasting and other areas. Generally we utilize the Bayesian model to learn about values of parameters - say, appearing in forecasting or demand equations- as follows: in step 1, our initial or "prior" information, denoted by I, regarding possible values of the parameters in the vector θ′ = (θ1,θ2, . . . , θm) is summarized in a prior probability density function, denoted by π(θ | I). In step 2, we represent the information in our current data, y, by use of a likelihood function, denoted by L(θ | y, I). In step 3, we combine our prior information and our sample information, using Bayes' theorem, to obtain a posterior distribution for the parameters, as follows:
g(θ | y, I) = cπ(θ | I)L(θ | y, I) (1.1)
where c, the factor of proportionality, is a normalizing constant such that ∫ g(θ | y, I) dθ = 1. It is the case that g in equation (1.1) contains all the information, prior and sample, and thus (1.1) is a 100 percent efficient information processing procedure, as I have shown in the recent literature12 regarding a new information theoretic derivation of (1.1). What was done was to consider the problem as an engineer might do, namely to consider measures of the input information and of the output information and to find a proper output density, g, that minimizes the difference between the output information and the input information. Using conventional information measures, surprisingly, the solution to this problem is given in equation (1.1), Bayes' theorem. See discussion of this result by Jaynes, Hill, Kullback, Bernardo and Smith after my first paper on this topic.13 In particular, Jaynes remarked that this demonstration was the first to show a direct connection between information theory (or entropy theory) and Bayes' theorem, and that there was much more work that could be done to produce other, perhaps more general, learning rules. In my response to Jaynes and others, and in my later work, some such extensions have been derived to provide a variety of static and dynamic learning models, which are generalized versions of Bayes' theorem reflecting additional conditions and constraints.
In addition to the above information theoretic derivation of Bayes' theorem, a traditional probability theory proof of Bayes' theorem is available to justify (1.1). That is, from the joint probability density function (pdf), p(y, θ) for the observations, y, and the parameter vector, θ, we have, given our prior information I, p(θ, y | I) = π(θ | I) f(y, | θ, I) = h(y | I)g(θ | y, I) from the product rule of probability, where f(y | θ, I) is the pdf for the observations given the parameters and prior information and h(y | I) is the marginal density of the observations. Then on solving for g(θ | y, I), we have:
g(θ | y, I) = π(θ | I)L(θ | y, I)/h(y, I) (1.2)
with the likelihood function defined by L(θ | y, I) ≡ f(y | θ, I) and 1/h(y | I) is the factor of proportionality c in equation (1.1). Note that the derivation of (1.2) via probability theory relies on the product rule of probability; see Jeffreys14 for a penetrating discussion of the assumptions needed for proof of the product rule of probability, which he points out may not be satisfied in all circumstances, and his admission that he was not able to derive an alternative proof under weaker conditions, which led him to introduce the product rule not as a theorem but as an axiom in his theory of probability. Thus it is interesting to note, as pointed out above, that Bayes' theorem or learning model, and generalizations of it, can also be derived as solutions to optimization problems.
Having the posterior density in (1.2), it is well known that it can be employed to calculate the probability that a parameter's value lies between a and b, two given numbers, and to construct posterior confidence intervals and regions for a parameter or set of parameters. Also, an optimal estimate for parameters is obtained by choosing such an estimate to minimize the posterior expectation of a given loss function. For example, for a quadratic loss function, it is well known that the optimal estimate for θ is the posterior mean, θ* = Eθ | D = ∫ θg(θ | D) dθ, where D = (y, I); for an absolute error loss function, it is the median; and, for a zero-one loss function, it is the modal value. Optimal estimates have been derived for many other loss functions, including "two-part" loss functions,15 e.g. with one part emphasizing goodness of fit, as in least squares, and the other precision of estimation. It has also been recognized in many problems, including medical, real estate assessment, policy and forecasting, that asymmetry of loss functions is of great practical importance. For example, in forecasting it is often the case that over-forecasting by a certain amount can be much more serious than an under-forecast of the same magnitude. The same is true in the medical area. There are now many papers in the literature dealing with the solution of problems involving asymmetric loss functions.16 That estimates and predictions that are optimal relative to asymmetric loss functions can be easily computed and often differ substantially from those that are optimal relative to symmetric loss functions is most noteworthy. In non-Bayesian approaches to inference, it is not as direct to derive estimates, predictions and forecasts that are optimal relative to asymmetric and many other loss functions.
Further, analytically and empirically, it has been established that optimal Bayesian estimates have rather good sampling properties - that is, good average performance in repeated trials, as when a procedure is built into a computer program and used over and over again on similar problems. Of course, if we are concerned about just one decision, the criterion of performance in repeated samples may not be very relevant, just as in visiting a restaurant on a particular evening. Many times we are just concerned with the performance of the restaurant on one evening, not on average. However, in some contexts average performance - say, in ranking restaurants, or wines, or statistical procedures - is relevant, and it is fortunate that Bayesian procedures have good average performance or risk properties, as shown analytically and in Monte Carlo experiments. For some recent striking examples of the outstanding performance of Bayesian estimators vis-à-vis non-Bayesian estimators in the case of estimating the parameters of the widely used Nerlove agricultural supply model, see papers by Diebold and Lamb, and Shen and Perloff, and - for other models - Tsurumi, Park, Gao and Lahiri, and Zellner.17 In these studies, the sampling performance of various Bayesian estimation procedures was shown to be better than that of leading non-Bayesian estimation procedures, including maximum likelihood, Fuller's modified maximum likelihood, two-stage least squares, ordinary least squares, etc.
Further, Bayesian methods have been utilized to produce Stein-like shrinkage estimates and forecasts that have rather good sampling and forecasting properties; see, e.g., Berger, Jorion, Min and Zellner, Quintana, Putnam and their colleagues, Zellner, Hong and Min, Zellner and Vandaele18 and references cited in these works. Quintana and Putnam talk about "shrinking forecasts to stretch returns" in connection with their work in forecasting returns to form financial portfolios using Bayesian optimization procedures. It is indeed the case that improved estimation and forecasting techniques are not only of theoretical interest but are having an impact on practical applications in financial portfolio formation, forecasting and other areas.
Now, suppose that we partition the vector of parameters in the posterior density in equation (1.2) as follows, θ = (θ1, θ2), and that we are interested in learning about the value of θ1 and regard the parameters in θ2 to be "nuisance" parameters. How do we get rid of the nuisance parameters? The answer is very simple; we just integrate them out of the joint posterior density - a standard procedure in the calculus that can be performed analytically or numerically. That is, the marginal density for θ1 is simply given by integrating θ2 out of the joint density to obtain the marginal density for θ1 as follows:
g(θ1 | D) | = | ∫ g(θ1, g(θ2 | D) dθ2 | |||
= | ∫ g(θ1|θ2, D)g(θ2 | D) dθ2 | (1.3) |
where, in the second line of (1.3), g(θ1 | θ2, D) is the conditional density for θ1 given θ2 and the data, D, and g(θ2 | D) is the marginal density of θ2 given the data.
© Cambridge University Press