Stochastic Distribution Control System Design: A Convex Optimization Approach / Edition 1 available in Hardcover
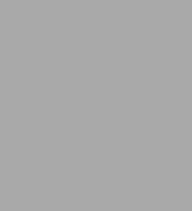
Stochastic Distribution Control System Design: A Convex Optimization Approach / Edition 1
- ISBN-10:
- 1849960291
- ISBN-13:
- 9781849960298
- Pub. Date:
- 05/24/2010
- Publisher:
- Springer London
- ISBN-10:
- 1849960291
- ISBN-13:
- 9781849960298
- Pub. Date:
- 05/24/2010
- Publisher:
- Springer London
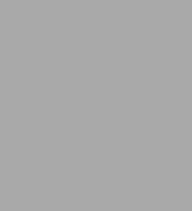
Stochastic Distribution Control System Design: A Convex Optimization Approach / Edition 1
Hardcover
Buy New
$109.99Overview
Product Details
ISBN-13: | 9781849960298 |
---|---|
Publisher: | Springer London |
Publication date: | 05/24/2010 |
Series: | Advances in Industrial Control |
Edition description: | 2010 |
Pages: | 196 |
Product dimensions: | 6.10(w) x 9.25(h) x 0.02(d) |
About the Author
2003–present: Full professor with research activities on shastic control, nonlinear control, filter design and fault detection, in Institute of Automation, BUAA, Beijing, P R China. He is also affiliated as a full professor with Research Institute of Automation, Southeast University, China.
2002–2003: Research fellow at Dept. Paper Science, UMIST, Manchester, UK.
2001–2002: Research associate in Department of Automobile and Aeronautical Engineering, Loughborough University, UK;
2000–2001: Research associate in Department of Mechanical Engineering, Glasgow University, UK;
1999–2000: Postdoctoral research fellow in IRCCyN, CNRS, Nantes, France, sponsored by Pays de la Loire project.
Following Professor Guo's previous work on shastic distribution control at UMIST, his recent research is mainly focused on the new developments of non-Gaussian filtering algorithms for signal processing and the shape control of shastic distributions using LMIs. This includes the developments of nonlinear observers and LMI techniques for adaptive tuning rules for nonlinear systems.
Professor Lei Guo will (with John Bailleul of Boston University) be general chair of the IEEE Conference on Decision and Control and Chinese Control Conference being held jointly in Shanghai in December 2009.
Author 2: Professor Hong Wang:
1982: Received the BSc (first class) degree in Electrical Engineering from Huainan University of Technology, Anhui, P.R. China
1984: Received the MEng (first class) in Automatic Control from Huazhong Univ.Science & Tech, Wuhan, P.R. China
1987: Received the PhD degree in Power Systems Automation from Huazhong Univ. Science & Tech., Wuhan, P.R. China, received an outstanding PhD thesis award and three best papers awards.
2004–present: Professor in Process Control, Director of the Control Systems Centre, School of Electrical and Electronics Engineering, The University of Manchester (formally UMIST), Manchester, working on the control of shastic distributions for shastic systems, fault diagnosis and fault tolerant control andcomplex systems modeling.
2002–2003: Professor in Process Control, Control Systems Centre, UMIST, Manchester.
1999–2002: Reader in Process Control at UMIST, working on shastic distribution control, fault diagnosis and complex systems modeling.
1997–1999: Senior lecturer in Process Control at UMIST, working on shastic distribution control, fault diagnosis and complex systems modeling.
Prof Wang is a fellow of IEE, fellow of InstMC and IEEE Senior Member, and acted as an associate editor for the leading control theory journal (IEEE Transactions on Automatic Control), board member for 4 international journals, and a member of the IFAC Safeprocess Committee, the IFAC Adaptive and Learning Systems Commitee and a member of the IFAC Shastic Systems Committee. He is the originator of probability density function shape control and has published 190 papers in international journals and conferences (25 invited papers). He is the leading author of 3 books.
His research activites have been focused on: i. shastic distribution control and filtering of general non-Gaussian dynamic shastic systems and closed-loop entropy minimization; ii. fault detection, diagnosis and fault-tolerant control for dynamic systems; iii. artificial-neural-network-based control systems design and applications to complex systems such as papermaking, combusion systems and systems biology; and iv. plant-wide modeling, fault diagnosis and optimization.
Table of Contents
1 Developments in Stochastic Distribution Control Systems 1
1.1 Introduction 1
1.1.1 Paper Web Formation Systems 3
1.1.2 Flame Distribution Control 4
1.1.3 Challenging Issues 6
1.2 Stochastic Distribution Control when Output PDFs are Measurable 8
1.3 Stochastic Distribution Control when Output PDFs are Unmeasurable 11
1.4 Minimum Entropy Control 12
1.5 Stochastic Distribution Filtering Design 13
1.6 Conclusions 14
Part I Structural Controller Design for Stochastic Distribution Control Systems
2 Proportional Integral Derivative Control for Continuous-time Stochastic Systems 17
2.1 Introduction 17
2.2 Problem Formulation 18
2.2.1 Model Representation 18
2.2.2 Pseudo-PID Controller Structure 19
2.3 Pseudo-PID Controller Design 21
2.3.1 Solvability Condition 21
2.3.2 Feasible Design Procedures 23
2.3.3 Robust Pseudo-PID Controller 26
2.4 Simulations 27
2.5 Conclusions 29
3 Constrained Continuous-time Proportional Integral Derivative Control Based on Convex Algorithms 31
3.1 Introduction 31
3.2 Problem Formulation 32
3.2.1 B-spline Expansion and Dynamic Weight Model 32
3.2.2 Generalized PID Controller Design 34
3.3 Constrained PID Controller Design Based on LMIs 35
3.3.1 Peak-to-peak Performance Control 35
3.3.2 Peak-to-peak Tracking Performance 38
3.3.3 Peak-to-peak Constrained Tracking Control 40
3.4 Simulations 41
3.5 Conclusions 42
4 Constrained Discrete-time Proportional Integral Control Based on Convex Algorithms 45
4.1 Introduction 45
4.2 Problem Formulation 45
4.2.1 B-spline Expansion and Discrete-time Weight Dynamical Model 45
4.2.2 Target Weight Model and Discrete-time PI Controller 47
4.3 Robust Constrained Tracking Control 48
4.3.1 Solvability for Peak-to-peak Performance 48
4.3.2 Peak-to-peak Tracking Performance 51
4.3.3 Constrained Peak-to-peak Tracking Control 53
4.4 Simulations 54
4.5 Conclusions 55
Part II Two-step Intelligent Optimization Modeling and Control for Stochastic Distribution Control Systems
5 Adaptive Tracking Stochastic Distribution Control for Two-step Neural Network Models 63
5.1 Introduction 63
5.2 Output PDF Model Using B-spline Neural Network 64
5.3 Dynamic Neural Network Identification 65
5.4 Adaptive Tracking Control for Weight Reference Model 68
5.5 Illustrative Examples 71
5.5.1 Example 1 71
5.5.2 Example 2 72
5.6 Conclusions 76
6 Constrained Adaptive Proportional Integral Tracking Control for Two-step Neural Network Models with Delays 81
6.1 Introduction 81
6.2 Output PDF Model Using B-spline Neural Network 82
6.3 Time Delay DNNs Identification 83
6.4 Constrained PI Tracking Control for Weight Vector 87
6.5 Illustrative Examples 91
6.5.1 Example 1 91
6.5.2 Example 2 93
6.6 Conclusions 97
7 Constrained Proportional Integral Tracking Control for Takagi-Sugeno Fuzzy Model 101
7.1 Introduction 101
7.2 Problem Statement and Preliminaries 101
7.2.1 Output PDF Model Using B-spline Expansion 101
7.2.2 PI Controller Design Based on T-S Fuzzy Model 103
7.3 Main Results 104
7.3.1 Stability Analysis with L1 Measure Index 104
7.3.2 Peak-to-peak Tracking Performance 106
7.3.3 Constrained Peak-to-peak Tracking Control 108
7.4 An Illustrative Example 109
7.5 Conclusions 110
Part III Statistical Tracking Control - Driven by Output Statistical Information Set
8 Multiple-objective Statistical Tracking Control Based on Linear Matrix Inequalities 115
8.1 Introduction 115
8.2 Problem Formulation and Preliminaries 115
8.3 Formulation for the H2/H∞ Tracking Problem 117
8.4 Illustrative Examples 122
8.5 Conclusions 125
9 Adaptive Statistical Tracking Control Based on Two-step Neural Networks with Time Delays 129
9.1 Introduction 129
9.2 Problem Formulation and Preliminaries 129
9.3 Time Delay DNNs Identification 130
9.4 Variable Structure Tracking Control for Weight Vectors 132
9.5 An Illustrative Example 136
9.6 Conclusions 138
Part IV Fault Detection and Diagnosis for Stochastic Distribution Control Systems
10 Optimal Continuous-time Fault Detection Filtering 145
10.1 Introduction 145
10.2 Problem Formulation 146
10.3 Main Result 147
10.4 Numerical Examples 152
10.5 Conclusions 155
11 Optimal Discrete-time Fault Detection and Diagnosis Filtering 157
11.1 Introduction 157
11.2 Formulation of the FDD Problem with Guaranteed Cost Performance 158
11.3 The Optimal Fault Detection Filter Design 160
11.4 Fault Diagnosis Filter Design 162
11.5 Simulation Examples 165
11.6 Conclusions 166
Part V Conclusions
12 Summary and Potential Applications 173
12.1 Summary 173
12.2 Potential Applications of PDF Control 174
12.2.1 PDF Control in Data-driven Modeling 174
12.2.2 PDF Control in Data Dimension Reduction 175
12.2.3 PDF Control in Multi-scale Plant-wide Modeling for Fault Diagnosis and Fault Tolerant Control 175
References 179
Index 193