The Informational Complexity of Learning: Perspectives on Neural Networks and Generative Grammar
Among other topics, The Informational Complexity of Learning: Perspectives on Neural Networks and Generative Grammar brings together two important but very different learning problems within the same analytical framework. The first concerns the problem of learning functional mappings using neural networks, followed by learning natural language grammars in the principles and parameters tradition of Chomsky. These two learning problems are seemingly very different. Neural networks are real-valued, infinite-dimensional, continuous mappings. On the other hand, grammars are boolean-valued, finite-dimensional, discrete (symbolic) mappings. Furthermore the research communities that work in the two areas almost never overlap. The book's objective is to bridge this gap. It uses the formal techniques developed in statistical learning theory and theoretical computer science over the last decade to analyze both kinds of learning problems. By asking the same question - how much information does it take to learn? - of both problems, it highlights their similarities and differences. Specific results include model selection in neural networks, active learning, language learning and evolutionary models of language change. The Informational Complexity of Learning: Perspectives on Neural Networks and Generative Grammar is a very interdisciplinary work. Anyone interested in the interaction of computer science and cognitive science should enjoy the book. Researchers in artificial intelligence, neural networks, linguistics, theoretical computer science, and statistics will find it particularly relevant.
1113896187
The Informational Complexity of Learning: Perspectives on Neural Networks and Generative Grammar
Among other topics, The Informational Complexity of Learning: Perspectives on Neural Networks and Generative Grammar brings together two important but very different learning problems within the same analytical framework. The first concerns the problem of learning functional mappings using neural networks, followed by learning natural language grammars in the principles and parameters tradition of Chomsky. These two learning problems are seemingly very different. Neural networks are real-valued, infinite-dimensional, continuous mappings. On the other hand, grammars are boolean-valued, finite-dimensional, discrete (symbolic) mappings. Furthermore the research communities that work in the two areas almost never overlap. The book's objective is to bridge this gap. It uses the formal techniques developed in statistical learning theory and theoretical computer science over the last decade to analyze both kinds of learning problems. By asking the same question - how much information does it take to learn? - of both problems, it highlights their similarities and differences. Specific results include model selection in neural networks, active learning, language learning and evolutionary models of language change. The Informational Complexity of Learning: Perspectives on Neural Networks and Generative Grammar is a very interdisciplinary work. Anyone interested in the interaction of computer science and cognitive science should enjoy the book. Researchers in artificial intelligence, neural networks, linguistics, theoretical computer science, and statistics will find it particularly relevant.
109.99
In Stock
5
1
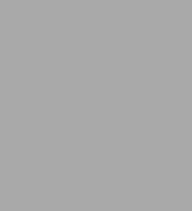
The Informational Complexity of Learning: Perspectives on Neural Networks and Generative Grammar
224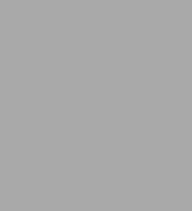
The Informational Complexity of Learning: Perspectives on Neural Networks and Generative Grammar
224Hardcover(1998)
$109.99
109.99
In Stock
Product Details
ISBN-13: | 9780792380818 |
---|---|
Publisher: | Springer US |
Publication date: | 11/30/1997 |
Edition description: | 1998 |
Pages: | 224 |
Product dimensions: | 6.10(w) x 9.25(h) x 0.03(d) |
From the B&N Reads Blog