Martin argues that the task of social statistics isn't to estimate parameters, but to reject false theory. He illustrates common pitfalls that can keep researchers from doing just that using a combination of visualizations, re-analyses, and simulations. Thinking Through Statistics gives social science practitioners accessible insight into troves of wisdom that would normally have to be earned through arduous trial and error, and it does so with a lighthearted approach that ensures this field guide is anything but stodgy.
Martin argues that the task of social statistics isn't to estimate parameters, but to reject false theory. He illustrates common pitfalls that can keep researchers from doing just that using a combination of visualizations, re-analyses, and simulations. Thinking Through Statistics gives social science practitioners accessible insight into troves of wisdom that would normally have to be earned through arduous trial and error, and it does so with a lighthearted approach that ensures this field guide is anything but stodgy.
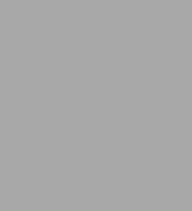
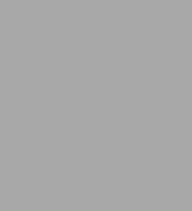
eBook
Available on Compatible NOOK devices, the free NOOK App and in My Digital Library.
Related collections and offers
Overview
Martin argues that the task of social statistics isn't to estimate parameters, but to reject false theory. He illustrates common pitfalls that can keep researchers from doing just that using a combination of visualizations, re-analyses, and simulations. Thinking Through Statistics gives social science practitioners accessible insight into troves of wisdom that would normally have to be earned through arduous trial and error, and it does so with a lighthearted approach that ensures this field guide is anything but stodgy.
Product Details
ISBN-13: | 9780226567778 |
---|---|
Publisher: | University of Chicago Press |
Publication date: | 05/31/2024 |
Sold by: | Barnes & Noble |
Format: | eBook |
Pages: | 376 |
Sales rank: | 310,192 |
File size: | 3 MB |
About the Author
Read an Excerpt
CHAPTER 1
Introduction
Map: I'm going to start by identifying a meta-problem: most of what we learn in "statistics" class doesn't solve our actual problems, which have to do with the fact that we don't know what the true model is — not that we don't know how best to fit it. This book can help with that — but first, we need to understand how we can use statistics to learn about the social world. I will draw on pragmatism — and falsificationism — to sketch out what I think is the most plausible justification for statistical practice.
Statistics and the Social Sciences
What Is Wrong with Statistics
Most of statistics is irrelevant for us. What we need are methods to help us adjudicate between substantively different claims about the world. In a very few cases, refining the estimates from one model, or from one class of models, is relevant to that undertaking. In most cases, it isn't. Here's an analogy: there's a lot of criticism of medical science for using up a lot of resources (and a lot of monkeys and rabbits) trying to do something we know it can't do — make us live forever. Why do researchers concentrate their attention on this impossible project, when there are so many more substantively important ones? I don't deny that this might be where the money is, but still, there are all sorts of interesting biochemical questions in how you keep a ninety-nine-year-old millionaire spry. But if you look worldwide, and not only where the "effective demand" is, you note that the major medical problems, in contrast, are simple. They're things like nutrition, exercise, environmental hazards, things we've known about for years. But those things, simple though they are, are difficult to solve in practice. It's a lot more fun to concentrate on complex problems for which we can imagine a magic bullet.
So too with statistical work. Almost all of the discipline of statistics is about getting the absolutely best estimates of parameters from true models (which I'll call "bestimates"). Statisticians will always admit that they consider their job only this — to figure out how to estimate parameters given that we already know the most important things about the world, namely the model we should be using. (Yes, there is also work on model selection that I'll get to later, and work on diagnostics for having the wrong model that I won't be able to discuss.) Unfortunately, usually, if we knew the right model, we wouldn't bother doing the statistics. The problem that we have isn't getting the bestimates of parameters from true models, it's about not having model results mislead us. Because what we need to do is to propose ideas about the social world, and then have the world be able to tell us that we're wrong ... and having it do this more often when we are wrong than when we aren't.
How do we do this? At a few points in this book, I'll use a metaphor of carpentry. To get truth from data is a craft, and you need to learn your craft. And one part of this is knowing when not to get fancy. If you were writing a book on how to make a chair, you wouldn't tell someone to start right in after sawing up pieces of wood with 280 grit, extra fine, sandpaper. You'd tell them to first use a rasp, then 80 grit, then 120, then 180, then 220, and so on. But most of our statistics books are pushing you right to the 280. If you've got your piece in that kind of shape, be my guest. But if you're staring at a pile of lumber, read on.
Many readers will object that it simply isn't true that statisticians always assume that you have the right model. In fact, much of the excitement right now involves adopting methods for classes of models, some of which don't even require that the true model be in the set you are examining (Burnham and Anderson 2004: 276). These approaches can be used to select a best model from a set, or to come up with a better estimate of a parameter across models, or to get a better estimate of parameter uncertainty given our model uncertainty. In sociology, this is going to be associated with Bayesian statistics, although there are also related information-theoretic approaches. The Bayesian notion starts from the idea that we are thinking about a range of models, and attempting to compare a posteriori to a priori probability distributions — before and after we look at the data.
Like almost everyone else, I've been enthusiastic about this work (take a look at Raftery 1985; Western 1996). But we have to bear in mind that even with these criteria, we are only looking at a teeny fraction of all possible models. (There are some Bayesian statistics that don't require a set of models, but those don't solve the problem I'm discussing here.) When we do model selection or model averaging, we usually have a fixed set of possible variables (closer to the order of 10 than that of 100), and we usually don't even look at all possible combinations of variables. And we usually restrict ourselves to a single family of specifications (link functions and error distributions, in the old GLM [General Linear Models] lingo).
Now I don't in any way mean to lessen the importance of this sort of work. And I think because of the ease of computerization, we're going to see more and more such exhaustive search through families of models. This should, I believe, increasingly be understood as "best practices," and it can be done outside of Bayesian framework to examine the robustness of our methods to other sorts of decisions. (For example, in an awesome paper recently, Frank et al. [2013] compared their preferred model to all possible permutations of all possible collapsings of certain variables to choose the best model.) But it doesn't solve our basic problem, which is not being able to be sure we're somewhere even close to the true model.
You might think that even if it doesn't solve our biggest problems, at least it can't hurt to have statisticians developing more rigorously defined estimates of model parameters. If we're lucky enough to be close to the true model, then our estimates will be way better, and if they aren't, no harm done. But in fact, it is often — though, happily, not invariably — the case that the approaches that are best for the perfect model can be worse for the wrong model.
When I was in graduate school, there was a lot of dumping on Ordinary Least Squares (OLS) regression. Almost never was it appropriate, we thought, and so it was, we concluded, the thing that thoughtless people would do and really, the smartest people wouldn't be caught dead within miles from a linear model anyway. We loved to list the assumptions of regression analysis, thereby (we thought) demonstrating how implausible it was to believe the results.
I once had two motorcycles. One was a truly drop dead gorgeous, 850 cc parallel twin Norton Commando, the last of the kick start only big British twins, with separate motor, gearbox, and primary chain, and a roar that was like music. The other was a Honda CB 400 T2 — boring, straight ahead, what at the time was jokingly called a UJM — a "Universal Japanese Motorcycle." No character whatsoever.
I knew nearly every inch of that Commando — from stripping it down to replace parts, from poring over exploded parts diagrams to figure out what incredibly weird special wrench might be needed to get at some insignificant part. And my wife never worried about the danger of me having a somewhat antiquated motorcycle when we had young children. The worst that happened was that sometimes I'd scrape my knuckles on a particularly stuck nut. Because it basically stayed in the garage, sheltering a pool of oil on the floor, while I worked on it.
The Honda, on the other hand, was very boring. You just pressed a button, it started, you put it in gear, it went forward, until you got where you were going and turned it off. If I needed to make an impression, I'd fire up the Norton. But if I needed to be somewhere "right now," I'd jump on the Honda. OLS regression is like that UJM. Easy to scorn, hard to appreciate — until you really need something to get done.
Proof by Anecdote
I find motorcycle metaphors pretty convincing. But if you don't, here's a simple example from some actual data, coming from the American National Election Study (ANES) from 1976. Let's say that you were interested in consciousness raising at around this time, and you're wondering whether the parties' different stances on women's issues made some sort of difference in voting behavior, so you look at congressional voting as a simple dichotomy, with 1 = Republican and 0 = Democrat. You're interested in the gender difference primarily, but with the idea that education may also make a difference. So you start out with a normal OLS regression. And we get what is in table 1.1 as model 1 (the R code is R1.1).
Gender isn't significant — there goes that theory — but education is. That's a finding! You write up a nice paper for submission, and show it to a statistician friend, very interested that those with more education are more likely to vote Republican. He smiles, and says that makes a lot of sense given that education would make people better able to understand the economic issues at hand (I think he is a Republican), but he tells you that you have made a major error. Your dependent variable is a dichotomy, and so you have run the wrong model. You need to instead use a logistic regression. He gives you the manual.
You go back, and re-run it as model 2. You know enough not to make the mistake of thinking that your coefficients from model 1 and model 2 are directly comparable. You note, to your satisfaction, that your basic findings are the same: the gender coefficient is around half its standard error, and the education coefficient around four times its standard error. So you add this to your paper, and go show it to an even more sophisticated statistician friend, and he says that your results make a lot of sense (I think he too is a Republican) but that you've made a methodological error. Actually, your cases are not statistically independent. ANES samples congressional districts, and persons in the same congressional district have a non-independent chance of getting in the sample. This is especially weighty because that means that they are voting for the same congressperson. "What do I do?" you ask, befuddled. He says, well, a robust standard error model could help with the non-independence of observations, but the "common congressperson" issue suggests that the best way is to add a random intercept at the district level.
So you take a minicourse on mixed models, and finally, you are able to fit what is in model 3: a hierarchical generalized linear model (HGLM). Your statistician friend (some friend!) was right — your coefficient for education has changed a little bit, and now its standard error is a bit bigger. But your results are all good! Pretty robust! But then you show it to me. It doesn't make sense to me that education would increase Republican vote by making people smarter (strike one) or by helping you understand economic issues (strike two). I tell you that I bet the problem is that educated people tend to be richer, not smarter. Your problem is a misspecification one, not a statistical one.
I have the data and quickly run an OLS and toss income in the mix (model 4). The row marked "SECRET" is the income measure (I didn't want you to guess where this is going — but you probably did anyway). Oh no! Now your education coefficient has been reduced to a thirteenth of its original size! It really looks like it's income, and not education, that predicts voting. Your paper may need to go right in the trash. "Hold on!" you think. "Steady now. None of these numbers are right. I need to run a binary logistic HGLM model instead! That might be the ticket and save my finding!" So you do model 5. And it basically tells you the exact same thing.
At this point, you are seriously thinking about murdering your various statistician friends. But it's not their fault. They did their jobs. But never send in a statistician to do a sociologist's job. They're only able to help you get bestimates of the right parameters. But you don't know what they are. The lesson — I know you get it, but it needs to stick — is that it rarely makes any sense to spend a lot of time worrying about the bells and whistles, being like the fool mentioned by Denis Diderot, who was afraid of pissing in the ocean because he didn't want to contribute to drowning someone. Worry about the omitted variables. That's what's really drowning you.
So moving away from OLS might be important for you, but in most cases, that isn't your problem. Indeed, OLS turns out to be pretty robust to violations of its assumptions. Sure, it doesn't give you the best estimates, but it doesn't go bonkers when you have restricted count data, even (often) just 0s and 1s. Further, and more important, it has a close relation to some model-independent characteristics of the data. You can interpret a "slope" coefficient as some sort of estimate of a causal effect, if you really want to ... or you can see it as a re-scaled partial correlation coefficient. And those descriptive interpretations can come in handy. Most methodologists these days are going to tell you to work closer and closer to a behavioral model. And I'm going to say that's one half of the story. Just like some politicians will say, "Work toward peace, prepare for war," I will say, "Work toward models, but prepare for description." And so I'm going to take a moment to lay out the theory of the use of data that guides the current work. But first, a little terminology.
Models, Measures, and Description
We're often a bit casual in talking about "models," "measures" and so on — statisticians aren't, and I think we should follow them here. A model is a statement about the real world that has testable implications: it can be a set of statements about independencies — certain variables can be treated as having no intrinsic association in the population — as in Leo Goodman's loglinear system. Or it can be a statement about mechanisms or processes — either causal pathways, or behavioral patterns.
Models usually have parameters in them. If the model is correct, these parameters may have a "real worldly" interpretation. For example, one of them might indicate the probability that persons of a certain type will do a certain type of thing. They might indicate the "elasticity" of an exchange between two types of resource. But they don't always need to have a direct real-world analogue. And our estimates of these parameters can be useful even when they aren't really very interpretable. In many cases, we use as a rule-of-thumb the statistical test of whether a parameter is likely non-zero in the population as a way of constraining the stories we tell about data. This approach has come in for a lot of hard knocks recently, perhaps deservedly, but I'll defend it below. For now, the point is that not all parameters have to be real-world interpretable for us to do something with them.
Let's go on and make a distinction between the estimate of a real-world parameter (should there be any) and measures that (as said in TTM) I'll use to refer to a process whereby we interact with the units of measurement (individually and singly, one might say) and walk away with information. Finally, when possible, we'll try to distinguish model parameters (and measurements) from descriptive statistics. While this distinction may get fuzzy in a few cases, the key is that descriptions are ways of summarizing information in a set of data that are model-independent. No matter what is going on in the world, a mean is a mean. Not to be intentionally confusing, but what a mean means stays the same. In contrast, a parameter in a complex model (like a structural equation measurement model) has no meaning if the model is seriously off.
That's the nature of good description. What you do is figure out ways of summarizing your data to simplify it, give you a handle on it, and the best descriptive methods structure the data to help you understand some especially useful aspects, ones that have to do with the nature of your data and the nature of your questions, but still without making use of particular assumptions about the world. When it comes to continuous data expressed in a correlation matrix (which is what OLS among others deals with), conventional factor analysis is a classic descriptive approach.
Now when I was in graduate school, factor analysis was the one thing we despised even more than OLS. It was, as Mike Hout called it, "voodoo" — magic, in contrast to a theoretically informed model. That's because something always came out, and, as I'll argue in chapter 9, when something "always comes out" it's usually very bad. But in contrast to other techniques that always give an interpretable result, factor analysis turns out to be pretty robust. Is it perfect? Of course not. Should you accept it as a model of the data? Of course not — because it isn't a model. It's a description, a reduction of the data. And chances are, it's going to point out something about the nature of the data you have.
(Continues…)
Excerpted from "Thinking Through Statistics"
by .
Copyright © 2018 The University of Chicago.
Excerpted by permission of The University of Chicago Press.
All rights reserved. No part of this excerpt may be reproduced or reprinted without permission in writing from the publisher.
Excerpts are provided by Dial-A-Book Inc. solely for the personal use of visitors to this web site.
Table of Contents
PrefaceChapter 1: Introduction
Chapter 2: Know Your Data
Chapter 3: Selectivity
Chapter 4: Misspecification and Control
Chapter 5: Where Is the Variance?
Chapter 6: Opportunity Knocks
Chapter 7: Time and Space
Chapter 8: When the World Knows More about the Processes than You Do
Chapter 9: Too Good to Be True
Conclusion
References
Index