At the vanguard of the Internet revolution are two computer scientists from Minnesota who are pioneers of Collaborative Filtering (CF). CF is a technology that enables companies to understand their customers and in turn sell products, goods, and services with remarkable success. To test CF, John Riedl and Joseph Konstan built two Internet sites, MovieLens and GroupLens, that allowed users to customize their preferences for movies and news.
The results were astounding -- MovieLens demonstrated amazing accuracy, almost ensuring that the recommendation would prove enjoyable. In "Word of Mouse," the authors analyze dozens of companies from Best Buy to Amazon to TiVo -- and show what these companies are doing right -- and what they are doing wrong.
At the vanguard of the Internet revolution are two computer scientists from Minnesota who are pioneers of Collaborative Filtering (CF). CF is a technology that enables companies to understand their customers and in turn sell products, goods, and services with remarkable success. To test CF, John Riedl and Joseph Konstan built two Internet sites, MovieLens and GroupLens, that allowed users to customize their preferences for movies and news.
The results were astounding -- MovieLens demonstrated amazing accuracy, almost ensuring that the recommendation would prove enjoyable. In "Word of Mouse," the authors analyze dozens of companies from Best Buy to Amazon to TiVo -- and show what these companies are doing right -- and what they are doing wrong.
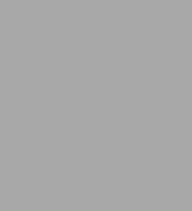
Word of Mouse: The Marketing Power of Collaborative Filtering
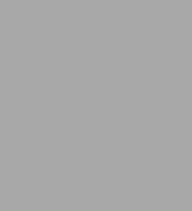
Word of Mouse: The Marketing Power of Collaborative Filtering
eBook
Available on Compatible NOOK devices, the free NOOK App and in My Digital Library.
Related collections and offers
Overview
At the vanguard of the Internet revolution are two computer scientists from Minnesota who are pioneers of Collaborative Filtering (CF). CF is a technology that enables companies to understand their customers and in turn sell products, goods, and services with remarkable success. To test CF, John Riedl and Joseph Konstan built two Internet sites, MovieLens and GroupLens, that allowed users to customize their preferences for movies and news.
The results were astounding -- MovieLens demonstrated amazing accuracy, almost ensuring that the recommendation would prove enjoyable. In "Word of Mouse," the authors analyze dozens of companies from Best Buy to Amazon to TiVo -- and show what these companies are doing right -- and what they are doing wrong.
Product Details
ISBN-13: | 9780759527270 |
---|---|
Publisher: | Grand Central Publishing |
Publication date: | 08/23/2002 |
Sold by: | Hachette Digital, Inc. |
Format: | eBook |
File size: | 771 KB |
About the Author
Read an Excerpt
Word of Mouse
By John Riedl Joseph Konstan and Eric Vrooman
Warner Books
Copyright © 2002 Professors John Riedl and Joseph KonstanAll right reserved.
ISBN: 0-446-69082-1
Chapter One
The Insider's Guide to Collaborative Filtering and Recommender SystemsAny sufficiently advanced technology is indistinguishable from magic. -ARTHUR C. CLARKE, PROFILES OF THE FUTURE (1962)
Man is a tool-using animal.... Without tools he is nothing, with tools, he is all. -THOMAS CARLYLE, SARTOR RESARTUS (1833-34)
A Brief History of Collaborative Filtering
Collaborative filtering is at the same time very new and very old. At its core, collaborative filtering is any mechanism whereby members of a community collaborate to identify what is good and what is bad. Even in prehistoric days, our species relied upon informal collaborative filtering. When a tribe encountered some new berry or root, they didn't all eat it simultaneously. Some people watched to see if the others became ill. If those who had eaten did get sick, the others would use this strong negative recommendation by avoiding that food (e.g., the deadly nightshade); if not, they would eat it themselves.
All sorts of knowledge was gained through observation of our neighbors. We learned which animals were dangerous and which were tasty. Then, as we developed into societies where people had time for art, philosophy, and science, the same process of collaborative filtering helped us decide which creations and theories were worth our time and money.
We imagine that many listeners sat transfixed by Homer's stories. Others might have considered him overrated and lobbied for their own favorite storyteller. These people, though they may not have known it at the time, were critics. As early as we had choices to make, we found critics to guide us. Today, as then, we can choose from among a variety of critics. Film critics help us decide which movies to see, theater critics lead us to the right play, and restaurant critics suggest a place to eat beforehand or afterward. In the financial world, analysts, brokers, and advisers recommend places to invest our money, and then members of the press critique the critics, helping us to better select our analysts, brokers, and advisers.
In addition to critics, we have editors and publishers to filter material for us. Because printing and distributing information can be expensive, commercial editors and publishers assess the marketability of a submitted work. On the opposite end of the spectrum, university presses, religion-affiliated publishers, and other not-for-profits are charged with forwarding an agenda. In either case, the editor and publisher work to identify content that they feel is worth distributing and, by implication, worth reading.
All of these-editors, publishers, critics, and even cave people -are examples of collaborative filtering. Collaborative filtering exists wherever people help filter out the wheat from the chaff, so the rest of us don't have to. In return, we reward them (all except the cave people) with our patronage and purchases.
The examples we've described above are all manual processes of collaborative filtering, however. Editors, publishers, and critics pick products based upon their expert opinions. In other words, by hand. They don't use automated systems to make these decisions for them. As a result, these editors and critics can't tailor their products and reviews for each individual customer. The New Yorker, for instance, doesn't publish a different magazine for each customer based upon his or her fields of interest. Instead, the New Yorker's audience is universal (their entire customer base and beyond). Sometimes, though, people want and need personalized material. This is where automated information-gathering systems come in: namely, collaborative filtering and its two predecessors, information retrieval and information filtering.
Information Retrieval
Collaborative filtering is not the only, nor even the most prominent, technology for helping people find what they want. As soon as large collections were created, people organized them for better search performance. From the Great Library at Alexandria to the Library of Congress, organization and cataloging have made content more accessible. Through combinations of author, title, and subject indexes, library patrons can quickly and easily find books that match explicit search criteria.
Indeed, the problem of information retrieval-finding information in a large catalog-lent itself well to computerization. Gerard Salton's seminal 1968 book, Automatic Information Organization and Retrieval, set forth the mechanisms for automatically indexing documents by examining the terms used within them (or within titles and summaries). Once a collection of documents is indexed in this way, a user can search for specific terms and retrieve documents of interest. Today, we see wide proliferation of such systems, including the widely used Web search engines. As the demand for search continues to grow, the quality of information-retrieval systems escalates as well.
Information Filtering
Information-retrieval systems address a particular information niche: cases where users have ephemeral information needs and want to meet those needs by using a relatively stable, indexed collection. In addition to Web searches and library catalogs, this niche includes a wide variety of search tasks from finding a file on your The Insider's Guide to Collaborative Filtering and Recommender Systems computer's hard drive (when you've forgotten where you stored it) to searching through newspaper archives or corporate records.
Sometimes, however, the situation is reversed. Users may have a relatively stable information need, and want to check new information content to see if any of it meets that need. Information-filtering systems address this niche by either being told or learning the user's need, and then examining a stream of new content to select items that meet it. The simplest information-filtering examples are clipping services. A corporate executive may want to see any newspaper articles that mention his company or its competitors. More sophisticated information-filtering systems help travelers find out when flights to a particular city go on sale, or avid readers discover when a favorite author has published a new book. Internally, information-filtering systems look like the mirror image of information-retrieval systems. The database stores a wide range of user profiles (or queries), and each new document gets passed through this database to see which profiles are triggered.
Computerized Collaborative Filtering
Both information retrieval and information filtering help people manage the problem of information overload by directing them to items that match their interests. Until recently, that seemed like enough, but the quantity of content available keeps increasing. In 1970, it may well have been possible for a corporate officer to read every article mentioning his company. By the year 2002, with the wide distribution of content on the Internet, it would take a team of officers to keep up. Something needed to be done to help people find items not only by topic, but also by quality or taste-and that something was the automation of collaborative filtering.
Automated collaborative filtering sprouted in three directions in quick succession, resulting in three different but compatible systems: pull-active CF, push-active CF, and automated CF. Since the three systems perform different tasks, you might even find all three on the same Web site.
PULL-ACTIVE CF
Tapestry is widely recognized as the first computerized collaborative filtering system. Developed at Xerox PARC as a research project, Tapestry was designed to help small workgroups team up to figure out which articles (usually electronic-bulletin-board articles) were worth reading. Tapestry users could annotate articles, for example, by marking them as "Fred should look at this" or "Excellent!!!" Other users could ask the system to find articles that met specific criteria, including the keywords in the article (à la information retrieval and filtering), the annotations left by others, and even the actions others took when seeing the article. For example, a user might say, "I want to see all the articles that Mary replied to, since if Mary replied to them, they must have been interesting." This type of collaborative filtering has become known as pull-active collaborative filtering because a user takes an active role in pulling recommendations out of the system (by forming queries).
PUSH-ACTIVE CF
Soon afterwards, David Maltz and Kate Ehrlich at Lotus Research developed a prototype push-active collaborative-filtering system. In their unnamed system, users who read interesting messages could easily "push" the content to others who might also value it. In some ways, this resembles today's joke-distribution chains, where jokes are forwarded to friends who (hopefully) share the same sense of humor. In organizations, a select number of people share the responsibility of gathering information and distributing it to the right people. These people, either officially or unofficially, serve as information hubs. Push-active CF made their tasks much easier.
AUTOMATED CF
At about the same time, the GroupLens project was developing automated collaborative filtering. The major difference between active and automated collaborative filtering is that active collaborative filtering requires human effort to establish the relationship between the people making and the people receiving recommendations. Accordingly, active solutions work best in small communities (workgroups, friends, or family) where people already know each other and their tastes.
Automated collaborative filtering uses each individual's history of interaction with the system to identify good recommenders for that individual. In the simplest form, automated collaborative-filtering systems keep track of each item a user has rated, along with how well the user liked that item. The system then figures out which users are good predictors for each other by examining the similarity between user tastes. Finally, the system uses these good predictors to find new items to recommend.
Soon after GroupLens appeared, a number of other automated collaborative-filtering systems emerged-clearly systems that were developed in parallel. MIT's Media Lab debuted the Ringo (later Firefly) music recommender, which used collaborative filtering to help people find music. And Bellcore created the Video Recommender, in which people rated movies by E-mail and received recommendations in reply. The number of independently generated collaborative-filtering systems suggests that its time had surely come.
The Role of Today's Marketer
With all this new recommender technology, some marketers are understandably concerned about their future. Will they be replaced the way tollbooth operators are being replaced by E-ZPass? Or as assembly-line workers have been replaced by machines? No. Recommenders need the right data, placement, and follow-through. They need human insight and direction. And they're only part of an overall marketing strategy. Recommenders are like tools on a carpenter's belt. So can marketers sleep easy? Yes, provided they know how, when, and where to employ recommenders.
This book examines many different recommender systems, in addition to collaborative filtering, so that marketers can initiate, update, or overhaul their recommendation practices. First, though, we should explain what we mean by marketing. The role of marketing and the marketer has evolved to keep pace with technological advances. Now, when we think of marketing, two separate fields emerge:
1. Manufacturer and wholesale marketing
2. Retailer marketing
Manufacturer and wholesale marketing refers to the efforts undertaken by manufacturers and distributors to promote products to merchants and the public, increase brand awareness, and generally position, price, and otherwise define the brand identity of a product. This is not the type of marketing we're addressing here.
Retailer marketing, which for small marketers has often been synonymous with merchandising, focuses on the smaller, more local decisions of how to promote, price, bundle, and sell products. Because this type of marketing can engage individual customers, it is the most ripe for recommender systems. This has been our area of study.
For small retailers, sales and marketing may overlap. A bikestore owner might decide that she needs to sell more Cannondales because of their high profit margins, so she advertises a free helmet with the purchase of a Cannondale mountain bike. When her customers come in, she can recommend the bike-and-helmet specials based upon biking preferences they've demonstrated in the past. And if she knows that the customers prefer recumbent bikes, she won't waste their time on Cannondale's Jekylls and Scalpels.
Marketers for large retailers have lost touch with the customer; they make decisions that guide and drive sales from a distance. To deliver the personalized service customers demand, they need to narrow the gap. We're not here to suggest that marketers become salespeople. We simply want them to deploy marketing techniques to serve each customer personally, the way good salespeople do.
The first step away from sales was mass marketing-the idea that a catalog, advertisement, or flyer could be sent to an extremely wide audience to get them to come buy. These marketing tools were necessarily untargeted but relatively cheap to produce. In an age of few alternatives (Sears or Montgomery Ward?), mass marketing The Insider's Guide to Collaborative Filtering and Recommender Systems works fairly well. But generic advertising doesn't reach out to people who are different from average. And it doesn't work when customers have many shopping alternatives to choose from.
The chinks in mass marketing's armor revealed themselves as media became more specialized. In the latter half of the twentieth century, a media explosion allowed marketers to narrowcast to audiences described by income, age, sex, race, religion, geography, and interest area. An advertisement for a product in a young women's magazine, for example, could address a different audience than an advertisement in a men's or parents' venue. In addition, the availability of categorized mailing lists made it possible to send different mailings and offers to smaller groups of people. Instead of getting a generic message, people received messages they were more likely to identify with.
Even demographic-based marketing has its limits, though. Real people don't fit cleanly into catalogs or simple categories.
Continues...
Excerpted from Word of Mouse by John Riedl Joseph Konstan and Eric Vrooman Copyright © 2002 by Professors John Riedl and Joseph Konstan . Excerpted by permission.
All rights reserved. No part of this excerpt may be reproduced or reprinted without permission in writing from the publisher.
Excerpts are provided by Dial-A-Book Inc. solely for the personal use of visitors to this web site.
Table of Contents
Foreword | vii | |
Introduction | xv | |
The Insider's Guide to Collaborative Filtering and Recommender Systems | 1 | |
Principle #1 | Demonstrate Product Expertise | 19 |
Principle #2 | Be a Customer Agent | 49 |
Principle #3 | Maintain Excellent Service Across Touchpoints | 73 |
Principle #4 | Box Products, Not People | 109 |
Principle #5 | Watch What I Do | 137 |
Principle #6 | Revolutionize Knowledge Management | 157 |
Principle #7 | Use Communities to Create Content | 179 |
Principle #8 | Turn Communities into Content | 203 |
The Future of Collaborative Filtering and Recommender Systems | 223 | |
Afterword | 235 | |
Acknowledgments | 239 | |
Index | 245 |