A First Course in Probability / Edition 6 available in Hardcover
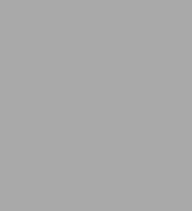
- ISBN-10:
- 0130338516
- ISBN-13:
- 9780130338518
- Pub. Date:
- 07/01/2001
- Publisher:
- Prentice Hall
- ISBN-10:
- 0130338516
- ISBN-13:
- 9780130338518
- Pub. Date:
- 07/01/2001
- Publisher:
- Prentice Hall
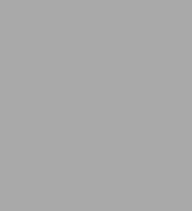
Buy New
$106.67Buy Used
$100.00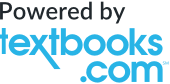
-
SHIP THIS ITEM— This item is available online through Marketplace sellers.
-
PICK UP IN STORECheck Availability at Nearby Stores
Available within 2 business hours
This item is available online through Marketplace sellers.
-
SHIP THIS ITEM
Temporarily Out of Stock Online
Please check back later for updated availability.
This item is available online through Marketplace sellers.
Overview
Product Details
ISBN-13: | 9780130338518 |
---|---|
Publisher: | Prentice Hall |
Publication date: | 07/01/2001 |
Edition description: | Older Edition |
Pages: | 528 |
Product dimensions: | 7.24(w) x 9.30(h) x 0.98(d) |
Table of Contents
Preface | vi | |
1 | Combinatorial Analysis | 1 |
1.1 | Introduction | 1 |
1.2 | The Basic Principle of Counting | 2 |
1.3 | Permutations | 3 |
1.4 | Combinations | 5 |
1.5 | Multinomial Coefficients | 10 |
1.6 | The Number of Integer Solutions of Equations | 12 |
Summary | 15 | |
Problems | 15 | |
Theoretical Exercises | 18 | |
Self-Test Problems and Exercises | 22 | |
2 | Axioms of Probability | 24 |
2.1 | Introduction | 24 |
2.2 | Sample Space and Events | 24 |
2.3 | Axioms of Probability | 28 |
2.4 | Some Simple Propositions | 31 |
2.5 | Sample Spaces Having Equally Likely Outcomes | 35 |
2.6 | Probability As a Continuous Set Function | 47 |
2.7 | Probability As a Measure of Belief | 51 |
Summary | 52 | |
Problems | 53 | |
Theoretical Exercises | 59 | |
Self-Test Problems and Exercises | 61 | |
3 | Conditional Probability and Independence | 64 |
3.1 | Introduction | 64 |
3.2 | Conditional Probabilities | 64 |
3.3 | Bayes' Formula | 69 |
3.4 | Independent Events | 83 |
3.5 | P(-[middle dot]F) is a Probability | 96 |
Summary | 103 | |
Problems | 104 | |
Theoretical Exercises | 115 | |
Self-Test Problems and Exercises | 119 | |
4 | Random Variables | 122 |
4.1 | Random Variables | 122 |
4.2 | Discrete Random Variables | 127 |
4.3 | Expected Value | 130 |
4.4 | Expectatio of a Function of a Random Variable | 133 |
4.5 | Variance | 137 |
4.6 | The Bernoulli and Binomial Random Variables | 139 |
4.6.1 | Properties of Binomial Random Variables | 144 |
4.6.2 | Computing the Binomial Distribution Function | 147 |
4.7 | The Poisson Random Variable | 149 |
4.7.1 | Computing the Poisson Distribution Function | 157 |
4.8 | Other Discrete Probability Distribution | 158 |
4.8.1 | The Geometric Random Variable | 158 |
4.8.2 | The Negative Binomial Random Variable | 160 |
4.8.3 | The Hypergeometric Random Variable | 162 |
4.8.4 | The Zeta (or Zipf) distribution | 166 |
4.9 | Properties of the Cumulative Distribution Function | 166 |
Summary | 169 | |
Problems | 171 | |
Theoretical Exercises | 180 | |
Self-Test Problems and Exercises | 184 | |
5 | Continuous Random Variables | 187 |
5.1 | Introduction | 187 |
5.2 | Expectation and Variance of Continuous Random Variables | 190 |
5.3 | The Uniform Random Variable | 195 |
5.4 | Normal Random Variables | 199 |
5.4.1 | The Normal Approximation to the Binomial Distribution | 206 |
5.5 | Exponential Random Variables | 210 |
5.5.1 | Hazard Rate Functions | 215 |
5.6 | Other Continuous Distributions | 217 |
5.6.1 | The Gamma Distribution | 217 |
5.6.2 | The Weibull Distribution | 220 |
5.6.3 | The Cauchy Distribution | 220 |
5.6.4 | The Beta Distribution | 221 |
5.7 | The Distribution of a Function of a Random Variable | 223 |
Summary | 225 | |
Problems | 228 | |
Theoretical Exercises | 232 | |
Self-Test Problems and Exercises | 235 | |
6 | Jointly Distributed Random Variables | 239 |
6.1 | Joint Distribution Functions | 239 |
6.2 | Independent Random Variables | 248 |
6.3 | Sums of Independent Random Variables | 260 |
6.4 | Conditional Distributions: Discrete Case | 268 |
6.5 | Conditional Distributions: Continuous Case | 270 |
6.6 | Order Statistics | 273 |
6.7 | Joint Probability Distribution of Functions of Random Variables | 277 |
6.8 | Exchangeable Random Variables | 285 |
Summary | 288 | |
Problems | 290 | |
Theoretical Exercises | 296 | |
Self-Test Problem and Exercises | 299 | |
7 | Properties of Expectation | 304 |
7.1 | Introduction | 304 |
7.2 | Expectation of Sums of Random Variables | 305 |
7.2.1 | Obtaining Bounds from Expectations via the Probabilistic Method | 321 |
7.2.2 | The Maximum-Minimums Identity | 324 |
7.3 | Covariance, Variance of Sums, and Correlations | 327 |
7.4 | Conditional Expectation | 340 |
7.4.1 | Definitions | 340 |
7.4.2 | Computing Expectations by Conditioning | 343 |
7.4.3 | Computing Probabilities by Conditioning | 350 |
7.4.4 | Conditional Variance | 354 |
7.5 | Conditional Expectation and Prediction | 356 |
7.6 | Moment Generating Functions | 361 |
7.6.1 | Joint Moment Generating Functions | 371 |
7.7 | Additional Properties of Normal Random Variables | 373 |
7.7.1 | The Multivariate Normal Distribution | 373 |
7.7.2 | The Joint Distribution of the Sample Mean and Sample Variance | 374 |
7.8 | General Definition of Expectation | 375 |
Summary | 377 | |
Problems | 379 | |
Theoretical Exercises | 389 | |
Self-Test Problems and Exercises | 397 | |
8 | Limit Theorems | 400 |
8.1 | Introduction | 400 |
8.2 | Chebyshev's Inequality and the Weak Law of Large Numbers | 400 |
8.3 | The Central Limit Theorem | 403 |
8.4 | The Strong Law of Large Numbers | 412 |
8.5 | Other Inequalities | 417 |
8.6 | Bounding the Error Probability When Approximating a Sum of Independent Bernoulli Random Variables by a Poisson | 424 |
Summary | 426 | |
Problems | 427 | |
Theoretical Exercises | 429 | |
Self-Test Problems and Exercises | 430 | |
9 | Additional Topics in Probability | 432 |
9.1 | The Poisson Process | 432 |
9.2 | Markov Chains | 435 |
9.3 | Surprise, Uncertainty, and Entropy | 440 |
9.4 | Coding Theory and Entropy | 445 |
Summary | 451 | |
Theoretical Exercises and Problems | 452 | |
Self-Test Problems and Exercises | 454 | |
References | 454 | |
10 | Simulation | 455 |
10.1 | Introduction | 455 |
10.2 | General Techniques for Simulating Continuous Random Variables | 458 |
10.2.1 | The Inverse Transformation Method | 458 |
10.2.2 | The Rejection Method | 459 |
10.3 | Simulating from Discrete Distributions | 465 |
10.4 | Variance Reduction Techniques | 467 |
10.4.1 | Use of Antithetic Variables | 468 |
10.4.2 | Variance Reduction by Conditioning | 468 |
10.4.3 | Control Variates | 470 |
Summary | 471 | |
Problems | 471 | |
Self-Test Problems and Exercises | 474 | |
References | 474 | |
Appendix A | Answers to Selected Problems | 475 |
Appendix B | Solutions to Self-Test Problems and Exercises | 478 |
Index | 519 |
Preface
This book is intended as an elementary introduction to the theory of probability for students in mathematics, statistics, engineering, and the sciences (including computer science, the social sciences and management science) who possess the prerequisite knowledge of elementary calculus. It attempts to present not only the mathematics of probability theory, but also, through numerous examples, the many diverse possible applications of this subject.
In Chapter 1 we present the basic principles of combinatorial analysis, which are most useful in computing probabilities.
In Chapter 2 we consider theaxioms of probability theory and show how they can be applied to compute various probabilities of interest.
Chapter 3 deals with the extremely important subjects of conditional probability and independence of events. By a series of examples we illustrate how conditional probabilities come into play not only when some partial information is available, but also as a tool to enable us to compute probabilities more easily, even when no partial information is present. This extremely important technique of obtaining probabilities by "conditioning" reappears in Chapter 7, where we use it to obtain expectations.
In Chapters 4, 5, and 6 we introduce the concept of random variables. Discrete random variables are dealt with in Chapter 4, continuous random variables in Chapter 5, and jointly distributed random variables in Chapter 6. The important concepts of the expected value and the variance of a random variable are introduced in Chapters 4 and 5: These quantities are then determined for many of the common types of random variables.
Additional properties of the expected value are considered in Chapter 7. Many examples illustrating the usefulness of the result that the expected value of a sum of random variables is equal to the sum of their expected values are presented. Sections on conditional expectation, including its use in prediction, and moment generating functions are contained in this chapter. In addition, the final section introduces the multi-variate normal distribution and presents a simple proof concerning the joint distribution of the sample mean and sample variance of a sample from a normal distribution.
In Chapter 8 we present the major theoretical results of probability theory. In particular, we prove the strong law of large numbers and the central limit theorem. Our proof of the strong law is a relatively simple one which assumes that the random variables have a finite fourth moment, and our proof of the central limit theorem assumes Levy's continuity theorem. Also in this chapter we present such probability inequalities as Markov's inequality, Chebyshev's inequality, and Chernoff bounds. The final section of Chapter 8 gives a bound on the error involved when a probability concerning a sum of independent Bernoulli random variables is approximated by the corresponding probability for a Poisson random variable having the same expected value.
Chapter 9 presents some additional topics, such as Markov chains, the Poisson process, and an introduction to information and coding theory, and Chapter 10 considers simulation.
The sixth edition continues the evolution and fine tuning of the text. There are many new exercises and examples. Among the latter are examples on utility (Example 4c of Chapter 4), on normal approximations (Example 4i of Chapter 5), on applying the lognormal distribution to finance (Example 3d of Chapter 6), and on coupon collecting with general collection probabilities (Example 2v of Chapter 7). There are also new optional subsections in Chapter 7 dealing with the probabilistic method (Subsection 7.2.1), and with the maximum-minimums identity (Subsection 7.2.2).
As in the previous edition, three sets of exercises are given at the end of each chapter. They are designated as Problems, Theoretical Exercises, and Self-Test Problems and Exercises. This last set of exercises, for which complete solutions appear in Appendix B, is designed to help students test their comprehension and study for exams.
Using the website students will be able to perform calculations and simulations quickly and easily in six key areas:- Three of the modules derive probabilities for, respectively, binomial, Poisson, and normal random variables.
- Another module illustrates the central limit theorem. It considers random variables that take on one of the values 0,1, 2, 3, 4 and allows the user to enter the probabilities for these values along with a number n. The module then plots the probability mass function of the sum of n independent random variables of this type. By increasing n one can "see" the mass function converge to the shape of a normal density function.
- The other two modules illustrate the strong law of large numbers. Again the user enters probabilities for the five possible values of the random variable along with an integer n. The program then uses random numbers to simulate n random variables having the prescribed distribution. The modules graph the number of times each outcome occurs along with the average of all outcomes. The modules differ in how they graph the results of the trials.