A First Course in Statistical Programming with R
This third edition of Braun and Murdoch's bestselling textbook now includes discussion of the use and design principles of the tidyverse packages in R, including expanded coverage of ggplot2, and R Markdown. The expanded simulation chapter introduces the Box–Muller and Metropolis–Hastings algorithms. New examples and exercises have been added throughout. This is the only introduction you'll need to start programming in R, the computing standard for analyzing data. This book comes with real R code that teaches the standards of the language. Unlike other introductory books on the R system, this book emphasizes portable programming skills that apply to most computing languages and techniques used to develop more complex projects. Solutions, datasets, and any errata are available from www.statprogr.science. Worked examples - from real applications - hundreds of exercises, and downloadable code, datasets, and solutions make a complete package for anyone working in or learning practical data science.
1119578082
A First Course in Statistical Programming with R
This third edition of Braun and Murdoch's bestselling textbook now includes discussion of the use and design principles of the tidyverse packages in R, including expanded coverage of ggplot2, and R Markdown. The expanded simulation chapter introduces the Box–Muller and Metropolis–Hastings algorithms. New examples and exercises have been added throughout. This is the only introduction you'll need to start programming in R, the computing standard for analyzing data. This book comes with real R code that teaches the standards of the language. Unlike other introductory books on the R system, this book emphasizes portable programming skills that apply to most computing languages and techniques used to develop more complex projects. Solutions, datasets, and any errata are available from www.statprogr.science. Worked examples - from real applications - hundreds of exercises, and downloadable code, datasets, and solutions make a complete package for anyone working in or learning practical data science.
46.99
In Stock
5
1
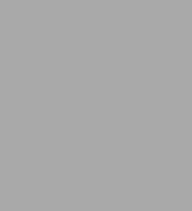
A First Course in Statistical Programming with R
280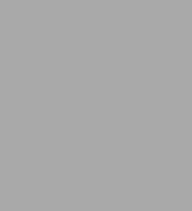
A First Course in Statistical Programming with R
280Paperback(3rd Revised ed.)
$46.99
46.99
In Stock
Product Details
ISBN-13: | 9781108995146 |
---|---|
Publisher: | Cambridge University Press |
Publication date: | 05/20/2021 |
Edition description: | 3rd Revised ed. |
Pages: | 280 |
Product dimensions: | 7.40(w) x 9.65(h) x 0.59(d) |
About the Author
From the B&N Reads Blog