A Wharton professor and tech entrepreneur examines how algorithms and artificial intelligence are starting to run every aspect of our lives, and how we can shape the way they impact us
Through the technology embedded in almost every major tech platform and every web-enabled device, algorithms and the artificial intelligence that underlies them make a staggering number of everyday decisions for us, from what products we buy, to where we decide to eat, to how we consume our news, to whom we date, and how we find a job. We've even delegated life-and-death decisions to algorithmsdecisions once made by doctors, pilots, and judges. In his new book, Kartik Hosanagar surveys the brave new world of algorithmic decision-making and reveals the potentially dangerous biases they can give rise to as they increasingly run our lives. He makes the compelling case that we need to arm ourselves with a better, deeper, more nuanced understanding of the phenomenon of algorithmic thinking. And he gives us a route in, pointing out that algorithms often think a lot like their creatorsthat is, like you and me.
Hosanagar draws on his experiences designing algorithms professionallyas well as on history, computer science, and psychologyto explore how algorithms work and why they occasionally go rogue, what drives our trust in them, and the many ramifications of algorithmic decision-making. He examines episodes like Microsoft's chatbot Tay, which was designed to converse on social media like a teenage girl, but instead turned sexist and racist; the fatal accidents of self-driving cars; and even our own common, and often frustrating, experiences on services like Netflix and Amazon. A Human's Guide to Machine Intelligence is an entertaining and provocative look at one of the most important developments of our time and a practical user's guide to this first wave of practical artificial intelligence.
"1129615959"
Through the technology embedded in almost every major tech platform and every web-enabled device, algorithms and the artificial intelligence that underlies them make a staggering number of everyday decisions for us, from what products we buy, to where we decide to eat, to how we consume our news, to whom we date, and how we find a job. We've even delegated life-and-death decisions to algorithmsdecisions once made by doctors, pilots, and judges. In his new book, Kartik Hosanagar surveys the brave new world of algorithmic decision-making and reveals the potentially dangerous biases they can give rise to as they increasingly run our lives. He makes the compelling case that we need to arm ourselves with a better, deeper, more nuanced understanding of the phenomenon of algorithmic thinking. And he gives us a route in, pointing out that algorithms often think a lot like their creatorsthat is, like you and me.
Hosanagar draws on his experiences designing algorithms professionallyas well as on history, computer science, and psychologyto explore how algorithms work and why they occasionally go rogue, what drives our trust in them, and the many ramifications of algorithmic decision-making. He examines episodes like Microsoft's chatbot Tay, which was designed to converse on social media like a teenage girl, but instead turned sexist and racist; the fatal accidents of self-driving cars; and even our own common, and often frustrating, experiences on services like Netflix and Amazon. A Human's Guide to Machine Intelligence is an entertaining and provocative look at one of the most important developments of our time and a practical user's guide to this first wave of practical artificial intelligence.
A Human's Guide to Machine Intelligence: How Algorithms Are Shaping Our Lives and How We Can Stay in Control
A Wharton professor and tech entrepreneur examines how algorithms and artificial intelligence are starting to run every aspect of our lives, and how we can shape the way they impact us
Through the technology embedded in almost every major tech platform and every web-enabled device, algorithms and the artificial intelligence that underlies them make a staggering number of everyday decisions for us, from what products we buy, to where we decide to eat, to how we consume our news, to whom we date, and how we find a job. We've even delegated life-and-death decisions to algorithmsdecisions once made by doctors, pilots, and judges. In his new book, Kartik Hosanagar surveys the brave new world of algorithmic decision-making and reveals the potentially dangerous biases they can give rise to as they increasingly run our lives. He makes the compelling case that we need to arm ourselves with a better, deeper, more nuanced understanding of the phenomenon of algorithmic thinking. And he gives us a route in, pointing out that algorithms often think a lot like their creatorsthat is, like you and me.
Hosanagar draws on his experiences designing algorithms professionallyas well as on history, computer science, and psychologyto explore how algorithms work and why they occasionally go rogue, what drives our trust in them, and the many ramifications of algorithmic decision-making. He examines episodes like Microsoft's chatbot Tay, which was designed to converse on social media like a teenage girl, but instead turned sexist and racist; the fatal accidents of self-driving cars; and even our own common, and often frustrating, experiences on services like Netflix and Amazon. A Human's Guide to Machine Intelligence is an entertaining and provocative look at one of the most important developments of our time and a practical user's guide to this first wave of practical artificial intelligence.
Through the technology embedded in almost every major tech platform and every web-enabled device, algorithms and the artificial intelligence that underlies them make a staggering number of everyday decisions for us, from what products we buy, to where we decide to eat, to how we consume our news, to whom we date, and how we find a job. We've even delegated life-and-death decisions to algorithmsdecisions once made by doctors, pilots, and judges. In his new book, Kartik Hosanagar surveys the brave new world of algorithmic decision-making and reveals the potentially dangerous biases they can give rise to as they increasingly run our lives. He makes the compelling case that we need to arm ourselves with a better, deeper, more nuanced understanding of the phenomenon of algorithmic thinking. And he gives us a route in, pointing out that algorithms often think a lot like their creatorsthat is, like you and me.
Hosanagar draws on his experiences designing algorithms professionallyas well as on history, computer science, and psychologyto explore how algorithms work and why they occasionally go rogue, what drives our trust in them, and the many ramifications of algorithmic decision-making. He examines episodes like Microsoft's chatbot Tay, which was designed to converse on social media like a teenage girl, but instead turned sexist and racist; the fatal accidents of self-driving cars; and even our own common, and often frustrating, experiences on services like Netflix and Amazon. A Human's Guide to Machine Intelligence is an entertaining and provocative look at one of the most important developments of our time and a practical user's guide to this first wave of practical artificial intelligence.
15.99
In Stock
5
1
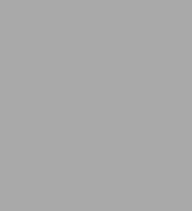
A Human's Guide to Machine Intelligence: How Algorithms Are Shaping Our Lives and How We Can Stay in Control
272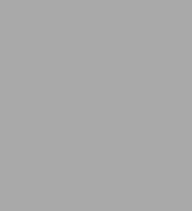
A Human's Guide to Machine Intelligence: How Algorithms Are Shaping Our Lives and How We Can Stay in Control
272Paperback(Reprint)
$15.99
$18.00
Save 11%
Current price is $15.99, Original price is $18. You Save 11%.
15.99
In Stock
Product Details
ISBN-13: | 9780525560906 |
---|---|
Publisher: | Penguin Publishing Group |
Publication date: | 03/10/2020 |
Edition description: | Reprint |
Pages: | 272 |
Sales rank: | 487,841 |
Product dimensions: | 5.40(w) x 8.30(h) x 0.80(d) |
About the Author
From the B&N Reads Blog