Advances in Decision Analysis: From Foundations to Applications available in Hardcover, Paperback
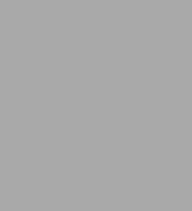
Advances in Decision Analysis: From Foundations to Applications
- ISBN-10:
- 0521682304
- ISBN-13:
- 9780521682305
- Pub. Date:
- 07/30/2007
- Publisher:
- Cambridge University Press
- ISBN-10:
- 0521682304
- ISBN-13:
- 9780521682305
- Pub. Date:
- 07/30/2007
- Publisher:
- Cambridge University Press
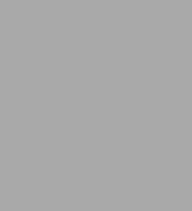
Advances in Decision Analysis: From Foundations to Applications
Buy New
$61.00Buy Used
$41.31-
SHIP THIS ITEMIn stock. Ships in 1-2 days.PICK UP IN STORE
Your local store may have stock of this item.
Available within 2 business hours
-
SHIP THIS ITEM
Temporarily Out of Stock Online
Please check back later for updated availability.
Overview
Product Details
ISBN-13: | 9780521682305 |
---|---|
Publisher: | Cambridge University Press |
Publication date: | 07/30/2007 |
Edition description: | New Edition |
Pages: | 638 |
Product dimensions: | 6.10(w) x 9.25(h) x 1.18(d) |
About the Author
Ralph F. Miles, Jr., received his Ph.D. in Physics from the California Institute of Technology and is a consultant in risk and decision analysis. He was the editor and coauthor of Systems Concepts and has written many articles. Until 1991, he worked as an engineer, supervisor, and manager in the Jet Propulsion Laboratory at the California Institute of Technology.
Detlof von Winterfeldt is Professor and Deputy Dean of the School of Policy, Planning, and Development at the University of Southern California. He is also Director of the Homeland Security Center for Risk and Economic Analysis of Terrorist Events at USC. He received his Ph.D. in Psychology from the University of Michigan. He cowrote Decision Analysis and Behavioral Research with Ward Edwards.
Read an Excerpt
Cambridge University Press
978-0-521-86368-1 - Advances in Decision Analysis - From Foundations to Applications - Edited by Ward Edwards, Ralph F. Miles and Detlof Von Winterfeldt
Excerpt
1 Introduction
Ward Edwards, Ralph F. Miles, Jr., and Detlof von Winterfeldt
This first chapter of Advances in Decision Analysis presents definitions for decision analysis that will be used consistently throughout this volume and provides a list of references on the subject of decision analysis. As this is an edited volume on “advances” in decision analysis, it is assumed that the reader is familiar with the subject to the level presented in one or more of the introductory decision analysis texts listed in the Preface.
This book attempts to maintain consistent distinctions among normative, prescriptive, and descriptive decision theories—distinctions that we find inconsistent in the literature. There is a rich and related literature on microeconomics, decision theory, behavioral psychology, and management science, which is only touched on in the following chapters.
Advances in Decision Analysis presents methodologies and applications of decision analysis as derived from prescriptive decision theory. Each of the first six parts of the book concentrates on different aspects of decision analysis. Part VII is devoted to applications ofdecision analysis.
The Rational Decision Maker
Many books in economics and decision analysis propose theories and methodologies that claim to be “rational.” Philosophers disagree on what is rational (Mele and Rawling 2004; Searle 2001). Many decision theories define the “rational decision maker” through mathematical principles or axioms, which, if combined, imply rational behavior (e.g., to maximize expected utility). But how compelling are these axioms? We would be remiss not to define what we mean by rationality.
For the purposes of this book, “rationality” will be interpreted as “Bayesian rationality,” with an emphasis on (1) decision making guided by maximizing subjective expected utility, and (2) the importance of information and the processing of that information through Bayes’ theorem. We take a pragmatic view of why this position is compelling. By pragmatic, we mean that systematic and repeated violations of these principles will result in inferior long-term consequences of actions and a diminished quality of life. See also Chapter 5: “Pragmatism” in McClennen (1990).
Pragmatism
The pragmatism of Peirce (1878) and James (1907) was preceded by that of Kant (1781), who, in his Critique of Pure Reason, makes two essential points: (1) The justification for reason is ends for happiness and (2) it cannot be obtained a priori from logic:
The sole business of reason is to bring about a union of all the ends, which are aimed at by our inclinations, into one ultimate end – that of happiness – and to show the agreement which should exist among the means of attaining that end. In this sphere, accordingly, reason cannot present to us any other than pragmatical laws of free action, for our guidance towards the aims set up by the senses, and is incompetent to give us laws which are pure and determined completely á priori.
In many ways, the behavioral movement of psychology and economics and the philosophical inclinations of the founding fathers of decision analysis aligned with this pragmatic philosophy. Many decision analysts today subscribe to the pragmatic idea that beliefs, values, and actions should serve the pursuit of happiness, whether this be wealth, health, quality of life, or an aggregation of those attributes into a general notion of utility.
Subjective Expected Utility (SEU)
There are three fundamental principles that are rational because they are pragmatic in the sense just discussed. These principles apply to choices with certain consequences as well as to choices with uncertain consequences (gambles). For generality, these principles are presented here for gambles, because sure things are simply degenerate gambles. The three principles are:
1. Transitivity.
2. The sure-thing principle.
3. Additivity of probability.
TRANSITIVITY. Transitivity states that if gamble f is preferred to gamble g, and gamble g is preferred to gamble h, then gamble f must be preferred to gamble h. The pragmatic argument for transitivity is that its intentional, systematic, and persistent violation subjects the decision maker to the possibility of becoming a “money pump” (Davidson, McKinsey, and Suppes 1955), and, in market situations, to be vulnerable to arbitrage opportunities.
SURE-THING PRINCIPLE. The sure-thing principle states that the preference between two gambles f and g, which have the same set of events and which have identical consequences in one of the events (but not necessarily in others), should not depend on what that identical consequence is. Much has been written about the rationality status of this principle since it was introduced by Savage (1954). To us, the convincing pragmatic argument is that violations of the sure-thing principle contradict a slightly generalized version of the monotonicity principle. The specific version of the monotonicity principle states that replacing a consequence in a gamble with a preferred consequence, creates a new gamble that is preferred to the original one. Luce (2003) refers to this condition as “consequence monotonicity,” to distinguish it form other types of monotonicity. The generalization applies this principle to the situation in which a consequence in a gamble can be a gamble itself (i.e., a second-stage gamble). It states that the replacement of a gamble with a preferred gamble creates a new gamble that is preferred to the original one. Violations of this principle can be shown to lead to money pump arguments similar to those of violating transitivity.
The power of the sure-thing principle is that it allows the separation of probabilities of events from the utilities of the consequences associated with these events. Technically, the principle allows the construction of an additive function over a series of events, where the consequences for each event are evaluated separately. Other technical principles ensure that the functions for evaluating events are related by positive linear transformations, thus leading to the interpretation of weights attached to events.
ADDITIVITY OF PROBABILITY. The weights attached to events can have very different interpretations. In decision analysis, these weights are interpreted as probabilities. The most important feature of these probabilities is that they are additive, that is, the probability of two mutually exclusive events is equal to the sum of the two probabilities. The principle underlying additivity of probability is what Luce (2003) calls “event monotonicity.” Event monotonicity requires that when we replace an event associated with a preferred consequence with an event that is more likely, and, at the same time, replace an event with a less-preferred consequence with a less likely one, we should prefer the new gamble over the original one.
Violation of event monotoncity or additivity subjects the decision maker to the sure long-term loss implied by the Dutch Book argument (de Finetti 1931, 1937; Kemeny 1955; Lehman 1955; Ramsey 1926; Shimony 1955). In other words, if you believe in nonadditive probabilities, someone can create a series of gambles that will lead to your eventual sure losses. Aside from avoiding the Dutch Book, the power of additive probabilities is that they open the door to the use of the whole apparatus of probability theory.
Acceptance of transitivity, the sure-thing principle, and the additivity of probability (plus some less important technical assumptions) inevitably leads to the acceptance of the subjective expected utility model of decision making (Savage 1954). Denying any of these principles leads to “nonexpected utility theories” that attempt to model descriptive decision making. Nau discusses these nonexpected utility theories in Chapter 14 in this volume.
Bayes’ Theorem
Bayes’ theorem is a trivial consequence of the definition of conditional probability, although calling it a “definition” is inadequate because there are intuitive notions as to what “conditionalization” means (Hájek 2003). Define p(A!E) = p(A∩E)/p(E) to be the probability of Event A conditional on Event E, where A and E are events and p(A) and p(E ) are unconditional probabilities of the events and where p(E ) ≠ 0.
Let Ai (i = 1,…,m) and Ej (j = 1,…,n) be partitions of the same event space. Then it can be shown that
Display matter not available in HTML version |
Credit is given to Thomas Bayes (1763) for the theorem, although the story is more complicated than that (Bernardo and Smith 1994; Dale 1999). The richness for conditionalization becomes apparent when p(Ai) is interpreted as the unconditional probability of Event Ai prior to observing the Event Ej, p(Ej ! Ai), which is interpreted as the probability of Event Ej conditional on observing Event Ai and called the “likelihood function.” The terms in the denominator are a normalizing function that equates to p(Ej).
The pragmatic argument for a bettor to use Bayes’ theorem as a rule for updating probabilities is that otherwise a bettor updating by Bayes’ theorem can construct a Dutch Book against a bookie who does not likewise update. Roughly, the bettor observes the bookie’s fair odds before and after the evidence obtains and places a series of fair bets such that the bettor is guaranteed to win no matter the outcome of the bets. For the details on how the Dutch Book is constructed, see Freedman and Purves (1969), Skyrms (1987, 1990, 1993), and Teller (1973, 1976).
Decision Theory
Decision theory, the foundation of decision analysis, has a long history, some of which is discussed in the next chapter. Briefly, decision theory started with two streams of thought: modification of the expected value model to account for nonlinear utilities and the introduction of subjective probabilities and use of Bayes’ theorem for updating probabilities. Both streams of thought date back to the eighteenth century. Daniel Bernoulli (1738), responding to several criticisms of the expected value model, proposed the use of a logarithmic utility function to replace the direct monetary consequences in an expected utility calculation. Although the notion of subjective probability was known since the 1660s (Hacking 1975), its implementation lay fallow until Thomas Bayes (1763) used subjective probability when he proposed an algorithm to update probabilities based on new data. It took two centuries for these ideas to culminate first in the expected utility model (von Neumann and Morgenstern 1947), and soon thereafter in the subjective expected utility model (Savage 1954). Many books on decision theory followed (see Chapter 2, in this volume). Subjective expected utility theory is the foundation of decision analysis to this date. The first conference on “decision processes” was held at the RAND Corporation in 1952 (Thrall, Coombs, and Davis 1954).
Another precedent of decision analysis was created in game theory by von Neumann and Morgenstern (1947), which was popularized by the very influential book by Luce and Raiffa (1957). Game theory is very much alive today in experimental economics and terrorism research, although it does not appear to have much direct impact on decision analysis.
Social choice theory is concerned with the rules that govern aggregate choices by individuals, including voting and selection of aggregate decision rules (Arrow 1963). Some of social choice theory has been used in multiple stakeholder applications of decision analysis (e.g., Dyer and Miles 1976).
Normative, Descriptive, and Prescriptive Decision Theories
Following Bell, Raiffa, and Tversky (1988), we can distinguish among three different perspectives in the study of decision making. In the normative perspective, the focus is on rational choice and normative models are built on basic assumptions (or axioms) that people should consider to provide logical guidance for their decisions. In the domain of decision making under risk or uncertainty, the traditional expected value model, the expected utility model of von Neumann and Morgenstern (1947), and the subjective expected utility model of Savage (1954) are the dominant normative models of rational choice. In the domain of beliefs, probability theory and Bayesian statistics in the presence of evidence for updating beliefs provide the normative foundation.
The descriptive perspective focuses on how real people make judgments and decisions, and some descriptive researchers develop mathematical models of actual behavior. Such models are judged by the extent to which their predictions correspond to the actual judgments and choices people make (Allais and Hagen 1979; Gilovich, Griffin, and Kahneman 2002; Kahneman, Slovic, and Tversky 1982; Kahneman and Tversky 2000). One of the most prominent descriptive models of decision making under uncertainty is the prospect theory model of Kahneman and Tversky (1979), later refined in Tversky and Kahneman (1992). The model captures many of the ways in which people deviate from the normative ideal of the expected utility model in a reasonably parsimonious form.
The prescriptive perspective focuses on helping people make better decisions by using normative models, but with awareness of the limitations of human judgment and of the practical problems of implementing a rational model in a complex world. Regarding the limitations of human judgment, the prescriptive perspective attempts to ensure that the tasks presented to experts and decision makers are sufficiently simple and are not affected by biases and errors known from descriptive studies. Training, tools, and debiasing techniques are used to avoid such biases and errors. To implement rational models in a complex world, it is important to simplify a complex decision environment to a manageable size for analysis. Savage (1954) recognized this issue when discussing the issues related to “small” and “large” worlds.
Decision Analysis
Decision analysis is unabashedly normative in theory and thoroughly prescriptive in practice. Its purpose is to assist decision makers to make better decisions. Decision analysis draws on the disciplines of mathematics, economics, behavioral psychology, and computer science.
The fundamentals of decision analysis were developed in the late 1950s and the ideas first appeared in book form with Schlaifer’s Probability and Statistics for Business Decisions (1959). Decision analysis came to early maturity in the 1960s when Howard (1965, 1966) merged decision theory and systems modeling. Howard named the new discipline “decision analysis.” Quoting Howard (1983): “Specifically, decision analysis results from combining the fields of systems analysis and statistical decision theory.” Howard edited the Special Issue on Decision Analysis (Howard 1968), in which he wrote “The Foundations of Decision Analysis” (Howard 1968a). Raiffa (1968) published the first book titled Decision Analysis. It is amazing that, with the help of Ward Edwards, in the same year Lusted, an M.D., published a book on medical decision making (Lusted 1968).
For the state of statistical decision theory at that time, see Raiffa and Schlaifer (1961) and much later, Pratt, Raiffa, and Schlaifer (1995). For an overview of systems analysis during that period, see Miles (1973). There are many books that are truly dedicated to decision analysis (Baird 1989; Bell, Keeney, and Raiffa 1977; Bell and Schleifer 1995; Brown 2005; Brown, Kahr, and Peterson 1974; Bunn 1984; Clemen 1996; French 1989; Golub 1997; Goodwin and Wright 1991; Hammond, Keeney, and Raiffa 1999; Howard and Matheson 1983a; Jeffrey 1983; Keeney 1978, 1992; Keeney and Raiffa 1976; Kirkwood 1997; Lindley 1985; Marshall and Oliver 1995; Morgan and Henrion 1990; von Winterfeldt and Edwards 1986; Watson and Buede 1987; Zeckhauser, Keeney, and Sebenius 1996). The Decision Analysis Society of the Institute for Operations Research and the Management Sciences (INFORMS) also has a quarterly journal, Decision Analysis.
What does decision analysis have to offer that other related disciplines do not? Research on the mathematical foundations of decision theory that are largely normative, in the sense that we have defined “normative,” can contribute to decision analysis, but is not decision analysis per se (Fishburn 1964, 1968, 1970, 1982; Jeffrey 1983, 1992; Kreps 1988; Luce 1959; Wakker 1989). Research that is axiomatic in nature, but violates the three fundamental principles of rationality (transitivity, sure-thing, and additivity of probabilities), cannot serve as a foundation of decision analysis (Bacharach and Hurley 1991; Fishburn 1988; Hooker, Leach, and McClennen 1978; Quiggin 1993; Stigum and Wenstøp, 1983).
Descriptive decision theory or behavioral psychology are important for their insight into the cognitive limitations of decision makers, but their conclusions are not always guidelines for rational decision making we have discussed. Some publications are a mixture of normative, prescriptive, and descriptive decision theory (Dawes 1988; Edwards 1992; Edwards and Tversky 1967; Gärdenfors and Sahlin 1988; Hogarth 1990; Kleindorfer, Kunreuther, and Schoemaker 1993; Shanteau, Mellers, and Schum 1999; Zeckhauser, Keeney, and Sebenius 1996). Management science combines economic and social science theories with analyses of real-world results, but these analyses are not always studies framed within the paradigm of decision analysis (Bazerman 1998; HBS 2001; Hoch and Kunreuther 2001; March 1994; Russo and Schoemaker 1989).
Philosophical studies cannot be construed as decision analysis (Eells 1982; Eells and Skyrms 1994; Gibbard 1990; Joyce 1999; Kaplan 1996; Kyburg and Teng 2001; Kyburg and Thalos 2003; Levi 1986; Maher 1993; Resnik 1987; Skyrms 1990; Sobel 1994; Weirich 2001). Microeconomics concentrates on theory and not on how decision makers would actually implement the theory (Kreps 1990; Mas-Colell, Whinston, and Green 1995). The same can be said for excellent studies on Bayesian statistics (Berger 1985; Bernardo and Smith 1994; Berry 1996; Gill 2002; Leonard and Hsu 1999; Robert 2001; Zellner 1971) or operations research (Hillier and Lieberman 2001).
Decision analysis is normative at its roots, but becomes prescriptive in recognizing the limitations of human decision makers and the complexity of the environment in which it has to be implemented. See Gigerenzer and Selten (2001), Simon (1982), and Rubenstein (1998) for a discussion of bounded rationality.
REFERENCES
Allais, M., and Hagen, O. (Eds.). (1979). Expected utility hypothesis and the Allais paradox. Dordrecht, The Netherlands: D. Reidel Publishing.
Arrow, K. J. (1963). Social choice and individual values. (2nd ed.). New Haven, CT: Yale University Press.
Bacharach, M., and Hurley. S. (Eds.). (1991). Foundations of decision theory: Issues and advances. Cambridge, MA: Blackwell.
Baird, B. F. (1989). Managerial decisions under uncertainty. New York: Wiley.
Bayes, T. (1763). An essay towards solving a problem in the doctrine of chances. Published posthumously in 1763 in Philosophical Transactions of the Royal Society, London, 53, 370–418 and 54, 296–325. Reprinted in 1958 in Biometrica, 45, 293–315.
Bazerman, M. (1998). Judgment in managerial decision making. (4th ed.). New York: John Wiley.
Bell, D. E., Keeney, R. L., and Raiffa, H. (Eds.). (1977). Conflicting objectives in decisions. New York: John Wiley.
Bell, D. E., Raiffa, H., and Tversky, A. (Eds.). (1988). Decision making: Descriptive, normative, and prescriptive interactions. Cambridge, UK: Cambridge University Press.
Bell, D. E., and Schleifer, Jr., A. (1995). Decision making under uncertainty. Cambridge, MA: Course Technology.
Berger, J. O. (1985). Statistical decision theory and bayesian analysis. (2nd ed.). New York: Springer-Verlag.
Bernardo, J. M., and Smith, A. F. M. (1994). Bayesian theory. New York: John Wiley.
Bernoulli, D. (1738). Specimen theoriae novae de mensura sortis. Comentarii academiae scientiarum imperiales petropolitanae, 5, 175–192. Translated into English by L. Sommer in 1954. Exposition of a new theory on the measurement of risk. Econometrica, XXII, 23–36.
Berry, D. A. (1996). Statistics: A Bayesian perspective. Belmont, CA: Wadsworth Publishing.
Brown, R. V. (2005). Tools of rational choice. New York: John Wiley.
Brown, R. V., Kahr, A. S., and Peterson, C. (1974). Decision analysis for the manager. New York: Holt, Rinehart, & Winston.
Bunn, D. V. (1984). Applied decision analysis. New York: McGraw-Hill.
Clemen, R. T. (1996). Making hard decisions: An introduction to decision analysis. (2nd ed.). Belmont, CA: Duxbury Press.
Dale, A. I. (1999). A history of inverse probability. (2nd ed.). New York: Springer.
Davidson, D., McKinsey, J. C. C., and Suppes, P. (1955). Outlines of a formal theory of value. Philosophy of Science, 22, 140–160.
Dawes, R. M. (1988). Rational Choice in an Uncertain World. Orlando, FL: Harcourt Brace Jovanovich.
de Finetti, B. (1931). Sul significato soggettivo della probabilità. Fundamenta mathematicae, 17, 298–329. Translated into English in 1993 in P. Monari and D. Cocchi (Eds.). On the subjective meaning of probability. Probabilitá e induzione. Bologna: Clueb, pp. 291–321.
de Finetti, B. (1937). La prévision: Ses lois logiques, ses sources subjectives. Annales del’Institut Henri Poincaré, 7, 1–68. Translated with corrections by H. E. Kyburg, Jr. Foresight. Its logical laws, its subjective sources. In H. E. Kyburg, Jr. and H. E. Smokler (Eds.). (1980). Studies in subjective probability. (2nd ed.). Huntington, NY: Krieger, pp. 53–111.
Dyer, J. S., and Miles, Jr., R. F. (1976). An actual application of collective choice theory to the selection of trajectories for the Mariner Jupiter/Saturn 1977 Project. Operations Research, 24, 220–244.
Edwards. W. (Ed.). (1992). Utility theory: Measurements and applications. Dordrecht, The Netherlands: Kluwer Academic Publishers.
Edwards, W., and Tversky, A. (Eds.). (1967). Decision Making: Selected Readings. Middlesex, UK: Penguin Books.
Eells, E. (1982). Rational decision and causality. Cambridge, UK: Cambridge University Press.
Eells, E., and Skyrms, B. (Eds.). (1994). Probability and conditionals: Belief revision and rational decision. Cambridge, UK: Cambridge University Press.
Fishburn, P. C. (1964). Decision and value theory. New York: John Wiley.
Fishburn, P. C. (1968). Utility theory. Management Science, 14, 335–378.
Fishburn, P. C. (1970). Utility theory for decision making. New York: John Wiley.
Fishburn, P. C. (1982). The foundations of expected utility. Dordrecht, The Netherlands: D. Reidel Publishing.
Fishburn, P. C. (1988). Nonlinear preference and utility theory. Baltimore, MD: Johns Hopkins University Press.
Freedman, D. A., and Purves, R. A. (1969). Bayes’ method for bookies. Annals of Mathematical Statistics, 40, 1177–1186.
French, S. (Ed.). (1989). Readings in decision analysis. Boca Raton, FL: Chapman & Hall/CRC.
Gärdenfors, P., and Sahlin, N.-E. (Eds.). (1988). Decision, probability, and utility: Selected readings. Cambridge, UK: Cambridge University Press.
Gibbard, A. (1990). Wise choices, apt feelings. Cambridge, MA: Harvard University Press.
Gigerenzer, G., and Selten, R. (Eds.). (2001). Bounded rationality: The adaptive toolkit. Cambridge, MA: MIT Press.
Gill, J. (2002). Bayesian methods: A social and behavioral sciences approach. Boca Raton, FL: Chapman & Hall/CRC.
Gilovich, T., Griffin, D., and Kahneman, D. (Eds.). (2002). Heuristics and biases: The psychology of intuitive judgment. Cambridge, UK: Cambridge University Press.
Golub, A. L. (1997). Decision analysis: An integrated approach. New York: John Wiley.
Goodwin, P., and Wright, G. (1991). Decision analysis for management judgment. New York: John Wiley.
Hacking, I. (1975). The Emergence of probability. Cambridge, UK: Cambridge University Press.
Hájek, A. 2003. What conditional probability could not be. Synthese, 137, 273–323.
Hammond, J. S., Keeney, R. L., and Raiffa, H. (1999). Smart choices: A practical guide to making better decisions. Boston, MA: Harvard Business School Press.
HBS. Harvard Business School Publishing. (2001). Harvard Business Review on decision making. Boston, MA: Harvard Business School Publishing.
Hillier, F. S., and Lieberman, G. J. (2001). Introduction to operations research. (7th ed.). Boston: McGraw-Hill.
Hoch, S. J., and Kunreuther, H. C. (Eds.). (2001). Wharton on making decisions. New York: John Wiley.
Hogarth, R. M. (1990). Insights in decision making: A tribute to Hillel J. Einhorn. Chicago, IL: University of Chicago Press.
Hooker, C. A., Leach, J. J., and McClennen, E. F. (Eds.). (1978). Foundations and applications of decision theory. Vol. Ⅰ: Theoretical foundations. Vol. Ⅱ: Epistemic and social applications. Dordrecht, The Netherlands: D. Reidel Publishing.
Howard, R. A. (1965). Bayesian decision models for system engineering. IEEE Transactions on Systems Science and Cybernectics, SSC-1, pp. 36–40.
Howard, R. A. (1966). Decision analysis: Applied decision theory. In Proceedings of the Fourth International Conference on Operational Research. New York: John Wiley, pp. 55–71.
Howard, R. A. (Ed.). (1968). Special issue on decision analysis. IEEE Transactions on Systems Science and Cybernetics, SSC-4. New York: IEEE.
Howard, R. A. (1968a). The foundations of decision analysis. In R. A. Howard, (1968). Special issue on decision analysis. IEEE Transactions on Systems Science and Cybernetics, SSC-4. New York: IEEE, pp. 211–219.
Howard, R. A. (1983). The evolution of decision analysis. In Howard, R. A., and Matheson, J. E. (Eds.). (1983). The principles and applications of decision analysis. Vol. Ⅰ: General collection, Vol. Ⅱ: Professional collection. Menlo Park, CA: Strategic Decisions Group, pp. 5–15.
Howard, R. A., and Matheson, J. E. (Eds.). (1983a). The principles and applications of decision analysis. Vol. Ⅰ: General collection, Vol. Ⅱ: Professional collection. Menlo Park, CA: Strategic Decisions Group.
INFORMS. Institute for Operations Research and the Management Sciences, Hanover, MD.
James, W. (1907). Pragmatism: A new name for some old ways of thinking. New York: Longmans, Green, & Co. Reprinted in 1995 with new footnotes as: Pragmatism. Mineola, NY: Dover Publications.
Jeffrey, R. C. (1983). The logic of decision. (2nd ed.). Chicago, IL: University of Chicago Press.
Jeffrey, R. C. (1992). Probability and the art of judgment. Cambridge, UK: Cambridge University Press.
Joyce, J. M. (1999). The foundations of causal decision theory. Cambridge, UK: Cambridge University Press.
Kahneman, D., Slovic, P., and Tversky, A. (Eds.). (1982). Judgment under uncertainty: Heuristics and biases. Cambridge, UK: Cambridge University Press.
Kahneman, D., and Tversky, A. (1979). Prospect theory: An analysis of decision under risk. Econometrica, 47, 263–291.
Kahneman, D., and Tversky, A. (Eds.). (2000). Choices, values, and frames. Cambridge, UK: Cambridge University Press.
Kant, I. (1781). Critique of pure reason. Translated in 1900 by J. M. D. Meiklejohn. London: Colonial Press. Reprinted in 2003 by Dover Publications, Mineola, NY, p. 449.
Kaplan, M. (1996). Decision theory as philosophy. Cambridge, UK: Cambridge University Press.
Keeney, R. L. (1978). Decision analysis. In J. J. Moder and S. E. Elmaghraby (Eds.), Handbook of operations research. New York: Van Nostrand Reinhold, pp. 423–450.
Keeney, R. L. (1992). Value-Focused thinking: A path to creative decision making. Cambridge, MA: Harvard University Press.
Keeney, R. L., and Raiffa, H. (1976). Decisions with multiple objectives: Preferences and value tradeoffs. New York: John Wiley.
Kemeny, J. G. (1955). Fair bets and inductive probabilities. Journal of Symbolic Logic, 20, 263–273.
Kirkwood, C. W. (1997). Strategic decision making: Multiobjective decision analysis with spreadsheets. Belmont, CA: Wadsworth/Thompson International.
Kleindorfer, P. R., Kunreuther, H. C., and Schoemaker, P. J. H. (1993). Decision sciences: An integrative perspective. Cambridge, UK: Cambridge University Press.
Kreps, D. M. (1988). Notes on the theory of choice. Boulder, CO: Westview Press.
Kreps, D. M. (1990). A course in microeconomic theory. Princeton, NJ: Princeton University Press.
Kyburg, H. E., Jr., and Teng, C. M. (2001). Uncertain inference. Cambridge, UK: Cambridge University Press.
Kyburg, H. E., Jr., and Thalos, M. (Eds.). (2003). Probability is the very guide of life. Chicago, IL: Open Court.
Lehman, R. S. (1955). On confirmation and rational betting. Journal of Symbolic Logic, 20, 251–262.
Leonard, T., and Hsu, J. S. J. (1999). Bayesian Methods: An analysis for statisticians and interdisciplinary researchers. Cambridge, UK: Cambridge University Press.
Levi, I. (1986). Hard choices: Decision making under unresolved conflict. Cambridge, UK: Cambridge University Press.
Lindley, D. V. (1985). Making decisions. (2nd ed.). New York: John Wiley.
Luce, R. D. (1959). Individual Choice Behavior: A Theoretical Analysis. New York: John Wiley. Reprinted in 2005 by John Wiley, NY.
Luce, R. D. (2003). Rationality in choice under certainty and uncertainty. In S. L. Schneider and J. Shanteau (Eds.), Emerging perspectives on judgment and decision research. Cambridge, UK: Cambridge University Press, pp. 64–83.
Luce, R. D., and Raiffa, H. (1957). Games and decisions: Introduction and critical survey. New York: John Wiley.
Lusted, L. B. (1968). Introduction to medical decision making. Springfield, IL: Charles Thomas.
Maher, P. (1993). Betting on theories. Cambridge, UK: Cambridge University Press.
March, J. G. (1994). A primer on decision making: How decisions happen. New York: Free Press.
Marshall, K. T., and Oliver, R. M. (1995). Decision making and forecasting: With emphasis on model building and policy analysis. New York: McGraw-Hill.
Mas-Colell, A., Whinston, M. D., and Green, J. R. (1995). Microeconomic theory. New York: Oxford University Press.
McClennen, E. F. (1990). Rationality and dynamic choice. Cambridge, UK: Cambridge University Press.
Mele, A. R., and Rawling, P. (Eds.). (2004). The Oxford handbook of rationality. New York: Oxford University Press.
Miles, Jr., R. F. (1973). Systems concepts: Lectures on contemporary approaches to systems. New York: John Wiley.
Morgan, M. G., and Henrion, M. (1990). Uncertainty: A guide to dealing with uncertainty in quantitative risk and policy analysis. Cambridge, UK: Cambridge University Press.
Peirce, C. S. (1878). How to make our ideas clear. Popular Science Monthly, 12, 286–302. Reprinted in 1955 in J. Buchler (Ed.), Philosophical writings of Peirce. New York: Dover Publications, pp. 23–41. Reprinted in 1992 in N. Houser and C. Klosel. The essential Peirce: Selected philosophical writings (Vol. 1: 1867–1893). Bloomington, IN: Indiana University Press, pp. 124–141.
© Cambridge University Press