This thesis demonstrates techniques that provide faster and more accurate solutions to a variety of problems in machine learning and signal processing. The author proposes a "greedy" algorithm, deriving sparse solutions with guarantees of optimality. The use of this algorithm removes many of the inaccuracies that occurred with the use of previous models.
1116466342
Algorithms for Sparsity-Constrained Optimization
This thesis demonstrates techniques that provide faster and more accurate solutions to a variety of problems in machine learning and signal processing. The author proposes a "greedy" algorithm, deriving sparse solutions with guarantees of optimality. The use of this algorithm removes many of the inaccuracies that occurred with the use of previous models.
169.99
In Stock
5
1
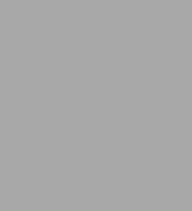
Algorithms for Sparsity-Constrained Optimization
107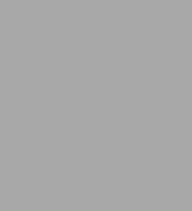
Algorithms for Sparsity-Constrained Optimization
107Paperback(Softcover reprint of the original 1st ed. 2014)
$169.99
169.99
In Stock
Product Details
ISBN-13: | 9783319377193 |
---|---|
Publisher: | Springer International Publishing |
Publication date: | 08/23/2016 |
Series: | Springer Theses , #261 |
Edition description: | Softcover reprint of the original 1st ed. 2014 |
Pages: | 107 |
Product dimensions: | 6.10(w) x 9.25(h) x (d) |
About the Author
From the B&N Reads Blog