5
1
9781461471370
An Introduction to Statistical Learning: with Applications in R / Edition 1 available in Hardcover
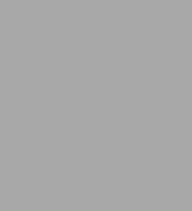
An Introduction to Statistical Learning: with Applications in R / Edition 1
- ISBN-10:
- 1461471370
- ISBN-13:
- 9781461471370
- Pub. Date:
- 09/01/2017
- Publisher:
- Springer New York
- ISBN-10:
- 1461471370
- ISBN-13:
- 9781461471370
- Pub. Date:
- 09/01/2017
- Publisher:
- Springer New York
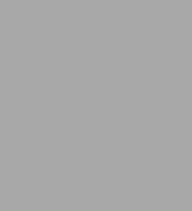
An Introduction to Statistical Learning: with Applications in R / Edition 1
$79.99
Current price is , Original price is $79.99. You
$79.99
This item is available online through Marketplace sellers.
$32.11
This item is available online through Marketplace sellers.
79.99
Out Of Stock
Product Details
ISBN-13: | 9781461471370 |
---|---|
Publisher: | Springer New York |
Publication date: | 09/01/2017 |
Series: | Springer Texts in Statistics , #103 |
Edition description: | 1st ed. 2013, Corr. 7th printing 2017 |
Pages: | 426 |
Product dimensions: | 6.20(w) x 9.30(h) x 1.00(d) |
About the Author
What People are Saying About This
From the B&N Reads Blog