Applications Of Data Mining In Computer Security concentrates heavily on the use of data mining in the area of intrusion detection. The reason for this is twofold. First, the volume of data dealing with both network and host activity is so large that it makes it an ideal candidate for using data mining techniques. Second, intrusion detection is an extremely critical activity. This book also addresses the application of data mining to computer forensics. This is a crucial area that seeks to address the needs of law enforcement in analyzing the digital evidence.
Applications Of Data Mining In Computer Security concentrates heavily on the use of data mining in the area of intrusion detection. The reason for this is twofold. First, the volume of data dealing with both network and host activity is so large that it makes it an ideal candidate for using data mining techniques. Second, intrusion detection is an extremely critical activity. This book also addresses the application of data mining to computer forensics. This is a crucial area that seeks to address the needs of law enforcement in analyzing the digital evidence.
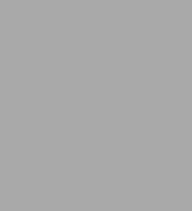
Applications of Data Mining in Computer Security
252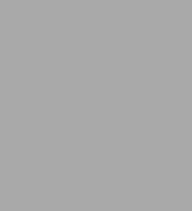
Applications of Data Mining in Computer Security
252Paperback(Softcover reprint of the original 1st ed. 2002)
Product Details
ISBN-13: | 9781461353218 |
---|---|
Publisher: | Springer US |
Publication date: | 10/23/2012 |
Series: | Advances in Information Security , #6 |
Edition description: | Softcover reprint of the original 1st ed. 2002 |
Pages: | 252 |
Product dimensions: | 6.10(w) x 9.25(h) x 0.02(d) |