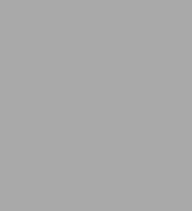
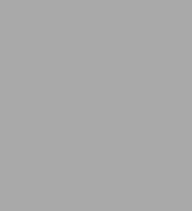
Hardcover(1st ed. 2017)
-
SHIP THIS ITEMNot Eligible for Free ShippingPICK UP IN STORECheck Availability at Nearby Stores
Available within 2 business hours
Related collections and offers
Overview
Product Details
ISBN-13: | 9783319542737 |
---|---|
Publisher: | Springer International Publishing |
Publication date: | 08/31/2017 |
Edition description: | 1st ed. 2017 |
Pages: | 275 |
Product dimensions: | 6.10(w) x 9.25(h) x (d) |
About the Author
Professor of Animal Breeding and Genetics
Visiting scientist at ABRO (Edinburgh), INRA (Jouy en Josas) and FAO (Rome). He was President of the World Rabbit Science Association and editor in chief of the journal World Rabbit Science. His career has focused on the genetics of litter size components and genetics of meat quality in rabbits and pigs. He has published more than one hundred papers in international journals. Invited speaker several times at the European Association for Animal Production and at the World Congress on Genetics Applied to Livesk Production among others. Chapman Lecturer at the University of Wisconsin. He has taught courses on Bayesian Inference at the universities of Valencia (Spain), Edinburgh (UK), Wisconsin (USA), Padua (Italy), Sao Paulo, Lavras (Brazil), Nacional (Uruguay), Lomas (Argentina) and at INRA in Toulouse (France).
Table of Contents
Foreword
Notation
1. Do we understand classical statistics?
1.1. Historical introduction
1.2. Test of hypothesis
1.2.1. The procedure
1.2.2. Common misinterpretations
1.3. Standard errors and Confidence intervals
1.3.1. Definition of standard error and confidence interval
1.3.2. Common misinterpretations
1.4. Bias and Risk of an estimator
1.4.1. Unbiased estimators
1.4.2. Common misinterpretations
1.5. Fixed and random effects
1.5.1. Definition of “fixed” and “random” effects
1.5.2. Shrinkage of random effects estimates
1.5.3. Bias, variance and Risk of an estimator when the effect is fixed or random
1.5.4. Common misinterpretations
1.6. Likelihood
1.6.1. Definition of likelihood
1.6.2. The method of maximum likelihood
1.6.3. Common misinterpretations
Appendix 1.1
Appendix 1.2
Appendix 1.3
Appendix 1.4
2. The Bayesian choice
2.1. Bayesian inference
2.1.1. The foundations of Bayesian inference
2.1.2. Bayes theorem
2.1.3. Prior information
2.2. Features of Bayesian inference
2.2.1. Point estimates: Mean, median, mode
2.2.2. Credibility intervals
2.2.3. Marginalisation
2.3. Test of hypotheses
2.3.1. Model choice
2.3.2. Bayes factors
2.3.3. Model averaging
2.4. Common misinterpretations
2.5. Bayesian Inference in practice
2.6. Advantages of Bayesian inference
Appendix 2.1
Appendix 2.2
Appendix 2.3
3. Posterior distributions
3.1. Notation
3.2. Probability density function
3.2.1. Definition
3.2.2. Transformed densities
3.3. Features of a distribution
3.3.1. Mean
3.3.2. Median
3.3.3. Mode
3.3.4. Credibility intervals
3.4. Conditional distribution
3.4.1. Bayes Theorem
3.4.2. Conditional distribution of the sample of a Normal distribution
3.4.3. Conditional distribution of the variance of a Normal distribution
3.4.4. Conditional distribution of the mean of a Normal distribution
3.5. Marginal distribution
3.5.1. Definition
3.5.2. Marginal distribution of the variance of a normal distribution
3.5.3. Marginal distribution of the mean of a normal distribution
Appendix 3.1
Appendix 3.2
Appendix 3.3
Appendix 3.4
4. MCMC
4.1. Samples of Marginal Posterior distributions
4.1.1. Taking samples of Marginal Posterior distributions
4.1.2. Making inferences from samples of Marginal Posterior distributions
4.2. Gibbs sampling
4.2.1. How it works
4.2.2. Why it works
4.2.3. When it works
4.2.4. Gibbs sampling features
4.2.5. Example
4.3. Other MCMC methods
4.3.1. Acceptance-Rejection
4.3.2. Metropolis
Appendix 4.1
5. The “baby” model
5.1. The model
5.2. Analytical solutions
5.2.1. Marginal posterior distribution of the mean and variance
5.2.2. Joint posterior distribution of the mean and variance
5.2.3. Inferences
5.3. Working with MCMC
5.3.1. The process
5.3.2. Using Flat priors
5.3.3. Using vague informative priors
5.3.4. Common misinterpretations
Appendix 5.1
Appendix 5.2
Appendix 5.3
6. The linear model. I. The “fixed” effects model
6.1. The model
6.1.1. The model
6.1.2. Example
6.1.3. Common misinterpretations
6.2. Marginal posterior distributions via MCMC using Flat priors
6.2.1. Joint posterior distribution
6.2.2. Conditional distributions
6.2.3. Gibbs sampling
6.3. Marginal posterior distributions via MCMC using vague informative priors
6.3.1. Vague in formative priors
6.3.2. Conditional distributions.
6.4. Least Squares as a Bayesian Estimator
Appendix 6.1
Appendix 6.2
7. The linear model. II. The “mixed” model
7.1. The mixed model with repeated records
7.1.1. The model
7.1.2. Common misinterpretations
7.1.3. Marginal posterior distributions via MCMC
7.1.4. Gibbs sampling
7.2. The genetic animal model
7.2.1. The model
7.2.2. Marginal posterior distributions via MCMC
7.3. Bayesian interpretation of BLUP and REML
7.3.1. BLUP in a frequentist context
7.3.2. BLUP as a Bayesian estimator
7.3.3. REML as a Bayesian estimator
7.4. The multitrait model
7.4.1. The model
7.4.2. Data augmentation
7.4.3. More complex models
Appendix 7.1
8. A scope of the possibilities of Bayesian inference + MCMC
8.1. Nested models: Examples in growth curves
8.1.1. The model
8.1.2. Marginal posterior distributions
8.1.3. More complex models
8.2. Modelling residuals. Examples in canalization.
8.2.1. The model
8.2.2. Marginal posterior distributions
8.2.3. More complex models
8.3. Modelling priors: Examples in genomic selection
8.3.1. The model
8.3.2. RR-BLUP
8.3.3. Bayes A
8.3.4. Bayes B
8.3.5. Bayes C and Bayes Cp
8.3.6. Bayes L (Bayesian Lasso)
8.3.7. Bayesian alphabet in practice
Appendix 8.1
9. Prior information
Abstract
9.1. Exact prior information
9.1.1. Prior information
9.1.2. Posterior probabilities with exact prior information
9.1.3. Influence of prior information in posterior probabilities
9.2. Vague prior information
9.2.1. A vague definition of vague prior information
9.2.2. Examples of the use of vague prior information
9.3. No prior information
9.3.1. Flat priors
9.3.2. Jeffrey’s priors
9.3.3. Bernardo’s “Reference” priors
9.4. Improper priors
9.5. The Achilles heel of Bayesian inference
Appendix 9.1
Appendix 9.2
10. Model choice
10.1 Model selection
10.1.1. The purpose of model selection
10.1.2. Fitting data vs predicting new records
10.1.3. Common misinterpretations
10.2. Hypothesis tests
10.2.1. Likelihood ratio test and other frequentist tests
10.2.2. Bayesian model choice
10.3. The concept of Information
10.3.1. Fisher information
10.3.2. Shannon information and entropy
10.3.3. Kullback Information and Divergence
10.4. Model selection criteria
10.4.1. Akaike Information Criterion (AIC)
10.4.2. Deviance Information Criterion (DIC)
10.4.3. Bayesian Information Criterion (BIC)
10.4.4. Model choice in practice
Appendix 10.1
Appendix 10.2
Appendix 10.3
Appendix 10.4
Appendix: Three new dialogues between Hylas and Filonus on scientific inference
References