Combining Fuzzy Imprecision with Probabilistic Uncertainty in Decision Making
In the literature of decision analysis it is traditional to rely on the tools provided by probability theory to deal with problems in which uncertainty plays a substantive role. In recent years, however, it has become increasingly clear that uncertainty is a mul tifaceted concept in which some of the important facets do not lend themselves to analysis by probability-based methods. One such facet is that of fuzzy imprecision, which is associated with the use of fuzzy predicates exemplified by small, large, fast, near, likely, etc. To be more specific, consider a proposition such as "It is very unlikely that the price of oil will decline sharply in the near future," in which the italicized words play the role of fuzzy predicates. The question is: How can one express the mean ing of this proposition through the use of probability-based methods? If this cannot be done effectively in a probabilistic framework, then how can one employ the information provided by the proposition in question to bear on a decision relating to an investment in a company engaged in exploration and marketing of oil? As another example, consider a collection of rules of the form "If X is Ai then Y is B,," j = 1, . . . , n, in which X and Yare real-valued variables and Ai and Bi are fuzzy numbers exemplified by small, large, not very small, close to 5, etc.
1112056989
Combining Fuzzy Imprecision with Probabilistic Uncertainty in Decision Making
In the literature of decision analysis it is traditional to rely on the tools provided by probability theory to deal with problems in which uncertainty plays a substantive role. In recent years, however, it has become increasingly clear that uncertainty is a mul tifaceted concept in which some of the important facets do not lend themselves to analysis by probability-based methods. One such facet is that of fuzzy imprecision, which is associated with the use of fuzzy predicates exemplified by small, large, fast, near, likely, etc. To be more specific, consider a proposition such as "It is very unlikely that the price of oil will decline sharply in the near future," in which the italicized words play the role of fuzzy predicates. The question is: How can one express the mean ing of this proposition through the use of probability-based methods? If this cannot be done effectively in a probabilistic framework, then how can one employ the information provided by the proposition in question to bear on a decision relating to an investment in a company engaged in exploration and marketing of oil? As another example, consider a collection of rules of the form "If X is Ai then Y is B,," j = 1, . . . , n, in which X and Yare real-valued variables and Ai and Bi are fuzzy numbers exemplified by small, large, not very small, close to 5, etc.
109.99
In Stock
5
1
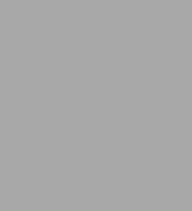
Combining Fuzzy Imprecision with Probabilistic Uncertainty in Decision Making
399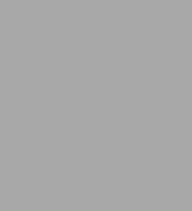
Combining Fuzzy Imprecision with Probabilistic Uncertainty in Decision Making
399Paperback(Softcover reprint of the original 1st ed. 1988)
$109.99
109.99
In Stock
Product Details
ISBN-13: | 9783540500056 |
---|---|
Publisher: | Springer Berlin Heidelberg |
Publication date: | 09/12/1988 |
Series: | Lecture Notes in Economics and Mathematical Systems , #310 |
Edition description: | Softcover reprint of the original 1st ed. 1988 |
Pages: | 399 |
Product dimensions: | 6.69(w) x 9.61(h) x 0.03(d) |
From the B&N Reads Blog