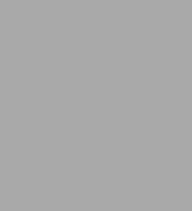
Data Analysis for Social Science: A Friendly and Practical Introduction
256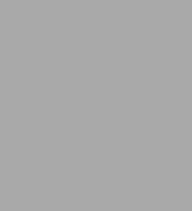
Data Analysis for Social Science: A Friendly and Practical Introduction
256Paperback
-
PICK UP IN STORECheck Availability at Nearby Stores
Available within 2 business hours
Related collections and offers
Overview
Data Analysis for Social Science provides a friendly introduction to the statistical concepts and programming skills needed to conduct and evaluate social scientific studies. Assuming no prior knowledge of statistics and coding and only minimal knowledge of math, the book teaches the fundamentals of survey research, predictive models, and causal inference while analyzing data from published studies with the statistical program R. It teaches not only how to perform the data analyses but also how to interpret the results and identify the analyses’ strengths and limitations.
- Progresses by teaching how to solve one kind of problem after another, bringing in methods as needed. It teaches, in this order, how to (1) estimate causal effects with randomized experiments, (2) visualize and summarize data, (3) infer population characteristics, (4) predict outcomes, (5) estimate causal effects with observational data, and (6) generalize from sample to population.
- Flips the script of traditional statistics textbooks. It starts by estimating causal effects with randomized experiments and postpones any discussion of probability and statistical inference until the final chapters. This unconventional order engages students by demonstrating from the very beginning how data analysis can be used to answer interesting questions, while reserving more abstract, complex concepts for later chapters.
- Provides a step-by-step guide to analyzing real-world data using the powerful, open-source statistical program R, which is free for everyone to use. The datasets are provided on the book’s website so that readers can learn how to analyze data by following along with the exercises in the book on their own computer.
- Assumes no prior knowledge of statistics or coding.
- Specifically designed to accommodate students with a variety of math backgrounds. It includes supplemental materials for students with minimal knowledge of math and clearly identifies sections with more advanced material so that readers can skip them if they so choose.
- Provides cheatsheets of statistical concepts and R code.
- Comes with instructor materials (upon request), including sample syllabi, lecture slides, and additional replication-style exercises with solutions and with the real-world datasets analyzed.
Looking for a more advanced introduction? Consider Quantitative Social Science by Kosuke Imai. In addition to covering the material in Data Analysis for Social Science, it teaches diffs-in-diffs models, heterogeneous effects, text analysis, and regression discontinuity designs, among other things.
Product Details
ISBN-13: | 9780691199436 |
---|---|
Publisher: | Princeton University Press |
Publication date: | 11/29/2022 |
Pages: | 256 |
Sales rank: | 199,417 |
Product dimensions: | 8.00(w) x 9.90(h) x 0.60(d) |
About the Author
Table of Contents
Preface xi
1 Introduction 1
1.1 Book Overview 3
1.2 Chapter Summaries 4
1.3 How to Use This Book 5
1.4 Why Learn to Analyze Data? 6
1.4.1 Learning to Code 6
1.5 Getting Ready 7
1.6 Introduction to R 8
1.6.1 Doing Calculations in R 9
1.6.2 Creating Objects in R 10
1.6.3 Using Functions in R 12
1.7 Loading and Making Sense of Data 14
1.7.1 Setting the Working Directory 15
1.7.2 Loading the Dataset 15
1.7.3 Understanding the Data 16
1.7.4 Identifying the Types of Variables Included 19
1.7.5 Identifying the Number of Observations 20
1.8 Computing and Interpreting Means 21
1.8.1 Accessing Variables inside Dataframes 21
1.8.2 Means 22
1.9 Summary 24
1.10 Cheatsheets 25
1.10.1 Concepts and Notation 25
1.10.2 R Symbols and Operators 26
1.10.3 R Functions 26
2 Estimating Causal Effects with Randomized Experiments 27
2.1 Project STAR 27
2.2 Treatment and Outcome Variables 28
2.2.1 Treatment Variables 29
2.2.2 Outcome Variables 29
2.3 Individual Causal Effects 29
2.4 Average Causal Effects 33
2.4.1 Randomized Experiments and the Difference-in-Means Estimator 35
2.5 Do Small Classes Improve Student Performance? 39
2.5.1 Relational Operators in R 39
2.5.2 Creating New Variables 40
2.5.3 Subsetting Variables 42
2.6 Summary 46
2.7 Cheatsheets 47
2.7.1 Concepts and Notation 47
2.7.2 R Symbols and Operators 50
2.7.3 R Functions 50
3 Inferring Population Characteristics via Survey Research 51
3.1 The EU Referendum In the UK 51
3.2 Survey Research 52
3.2.1 Random Sampling 53
3.2.2 Potential Challenges 54
3.3 Measuring Support for Brexit 55
3.3.1 Predicting the Referendum Outcome 56
3.3.2 Frequency Tables 57
3.3.3 Tables of Proportions 57
3.4 Who Supported Brexit? 58
3.4.1 Handling Missing Data 59
3.4.2 Two-Way Frequency Tables 62
3.4.3 Two-Way Tables of Proportions 64
3.4.4 Histograms 66
3.4.5 Density Histograms 68
3.4.6 Descriptive Statistics 71
3.5 Relationship between Education and the Leave Vote in the Entire UK 76
3.5.1 Scatter Plots 78
3.5.2 Correlation 82
3.6 Summary 88
3.7 Cheatsheets 90
3.7.1 Concepts and Notation 90
3.7.2 R Symbols and Operators 96
3.7.3 R Functions 96
4 Predicting Outcomes Using Linear Regression 98
4.1 GDP and Night-Time Light Emissions 98
4.2 Predictors, Observed vs. Predicted Outcomes, and Prediction Errors 99
4.3 Summarizing the Relationship between Two Variables with a Line 100
4.3.1 The Linear Regression Model 101
4.3.2 The Intercept Coefficient 103
4.3.3 The Slope Coefficient 104
4.3.4 The Least Squares Method 106
4.4 Predicting GDP Using Prior GDP 107
4.4.1 Relationship between GDP and Prior GDP 109
4.4.2 With Natural Logarithm Transformations 113
4.5 Predicting GDP Growth Using Night-Time Light Emissions 116
4.6 Measuring How Well the Model Fits the Data with the Coefficient of Determination, R2 120
4.6.1 How Well Do the Three Predictive Models in This Chapter Fit the Data? 122
4.7 Summary 123
4.8 Appendix: Interpretation of the Slope in the Log-Log Linear Model 124
4.9 Cheatsheets 126
4.9.1 Concepts and Notation 126
4.9.2 R Functions 128
5 Estimating Causal Effects with Observational Data 129
5.1 Russian State-Controlled TV Coverage of 2014 Ukrainian Affairs 129
5.2 Challenges of Estimating Causal Effects with Observational Data 130
5.2.1 Confounding Variables 130
5.2.2 Why Are Confounders a Problem? 131
5.2.3 Confounders in Randomized Experiments 133
5.3 The Effect of Russian TV on Ukrainians' Voting Behavior 135
5.3.1 Using the Simple Linear Model to Compute the Difference-in-Means Estimator 136
5.3.2 Controlling for Confounders Using a Multiple Linear Regression Model 142
5.4 The Effect of Russian TV on Ukrainian Electoral Outcomes 147
5.4.1 Using the Simple Linear Model to Compute the Difference-in-Means Estimator 149
5.4.2 Controlling for Confounders Using a Multiple Linear Regression Model 151
5.5 Internal and External Validity 153
5.5.1 Randomized Experiments vs. Observational Studies 153
5.5.2 The Role of Randomization 154
5.5.3 How Good Are the Two Causal Analyses in This Chapter? 155
5.5.4 How Good Was the Causal Analysis in Chapter 2? 156
5.5.5 The Coefficient of Determination, R2 157
5.6 Summary 157
5.7 Cheatsheets 159
5.7.1 Concepts and Notation 159
5.7.2 R Functions 161
6 Probability 162
6.1 What is Probability? 162
6.2 Axioms of Probability 163
6.3 Events, Random Variables, and Probability Distributions 165
6.4 Probability Distributions 166
6.4.1 The Bernoulli Distribution 166
6.4.2 The Normal Distribution 169
6.4.3 The Standard Normal Distribution 173
6.4.4 Recap 179
6.5 Population Parameters vs. Sample Statistics 179
6.5.1 The Law of Large Numbers 180
6.5.2 The Central Limit Theorem 183
6.5.3 Sampling Distribution of the Sample Mean 188
6.6 Summary 189
6.7 Appendix: For Loops 190
6.8 Cheatsheets 192
6.8.1 Concepts and Notation 192
6.8.2 R Symbols and Operators 194
6.8.3 R Functions 195
7 Quantifying Uncertainty 196
7.1 Estimators and Their Sampling Distributions 196
7.2 Confidence Intervals 202
7.2.1 For the Sample Mean 203
7.2.2 For the Difference-in-Means Estimator 206
7.2.3 For Predicted Outcomes 209
7.3 Hypothesis Testing 211
7.3.1 With the Difference-in-Means Estimator 218
7.3.2 With Estimated Regression Coefficients 220
7.4 Statistical vs. Scientific Significance 224
7.5 Summary 225
7.6 Cheatsheets 226
7.6.1 Concepts and Notation 226
7.6.2 R Symbols and Operators 229
7.6.3 R Functions 229
Index of Concepts 231
Index of Mathematical Notation 235
Index of R and RStudio 237
What People are Saying About This
“This is the book that I plan to teach from next time I teach introductory statistics. As it is, I recommend it as a reference for students in more advanced classes such as Applied Regression and Causal Inference, if they want a clean refresher from first principles.”—Andrew Gelman, coauthor of Regression and Other Stories“This is without doubt the best book to get started with data analysis in the social sciences. Readers learn best practices in research design, measurement, data analysis, and data visualization, all in an approachable and engaging way. My students—all of them complete novices—were easily able to conduct their own analyses after working through this book.”—Simon Weschle, Syracuse University“I love this book. More importantly, my students love this book. Data Analysis for Social Science is the perfect introduction to causal inference, probability and statistics, and the open-source programming language R, for students without prior experience. With multiple exercises using R Markdown and a variety of datasets drawn from the research literature, Data Analysis for Social Science gives students a hands-on path to build their skills and confidence.”—Anna Harvey, New York University“Data Analysis for Social Science is a game changer! I have been teaching quantitative methods for fourteen years, and I never had such good results and engagement from my students until I adopted this book. The logic behind the content structure is much more intuitive than usual, focusing on understanding the applications of quantitative methods (particularly linear regressions) before introducing the theory. The book and the instructor resources it comes with are incredibly practical and well designed, with relevant datasets and examples. After all these years, it is really refreshing to find a book that has students in mind and stresses intuition over abstraction, without sacrificing complexity and rigor.”—Javier Sajuria, Queen Mary University of London“Data Analysis for Social Science helped me teach introductory research methods at the right level for the types of students in my class. This book provides detailed explanations, step-by-step examples, and repetition to ensure complete beginners are not overwhelmed and slowly build confidence. I also use it as an optional text for higher-level courses because it clearly explains concepts even PhD students are often confused about. Furthermore, the instructor resources that come with it are the best I’ve seen provided with a textbook and made adopting the book much easier.”—Mark Richardson, Georgetown University“Data science from zero to sixty—gently, expertly, quickly.”—Gary King, Weatherhead University Professor, Harvard University“This book will transform the way we teach data science in the social sciences. Assuming zero background knowledge, it takes readers step-by-step through the most important concepts of data analysis and coding without sacrificing rigor. With clear explanations, beautiful visuals, and engaging examples, Data Analysis for Social Science is the obvious choice for any student looking to build their data science tool kit.”—Molly Roberts, University of California, San Diego“I highly recommend Data Analysis for Social Science! It is exceptionally well-written and cleverly organized. I particularly love its problem-solving approach and how it is intertwined with R code. While most textbooks teach statistics without offering students a clear motivation, this one teaches statistics as a way to solve real problems with real datasets. For example, if you want to estimate average causal effects with randomized experiments, then you must learn to compute the mean of a subset of the data. Or, if you want to understand the precision of your estimates, then you need to learn probability (but not beforehand!). I am using this book in my undergraduate courses with great satisfaction, and my students appreciate its easily understandable explanations.”—Guillermo Solovey, University of Buenos Aires“My favorite feature of Data Analysis for Social Science is that it puts causal inference first, before probability and statistical inference. I have found that this unconventional order is gentler and more engaging for complete beginners than the approach used in many other books. It also allows students with some prior knowledge of statistics to learn something new from the start.”—Max Goplerud, University of Pittsburgh“Data Analysis for Social Science is a great textbook for any undergraduate research methods course. I especially like that it teaches point estimates and uncertainty separately. In the past, when I taught these concepts together, I found students were overwhelmed. Breaking them up makes the statistics easier to understand. It’s a genius idea! I truly can’t recommend this book enough!”—Christopher Ojeda, University of California, Merced“I have been teaching statistics for twenty-five years and I have never seen a book this well done. Data Analysis for Social Science is such a perfect combination of what students need to know. The authors’ descriptions of the basic logic of causality, along with the many practical examples and visuals, are amazing features. Also, I have been resisting teaching intro students R because I am very watchful of overloading their bandwidth and I worry about killing their spirit with buggy code; I want them to love data analysis as much as I do! This book made me a convert. I am going to spend the time to learn R so that I can assign this book.”—Vanessa Baird, University of Colorado, Boulder“I have used Data Analysis for Social Science to teach required undergraduate courses with great success. Students liked the clear explanations and relevant real-world examples, and they even found coding in R fun! By the end, they walked away excited about how these skills opened up new career opportunities and helped them understand the research discussed in other classes.”—Alicia Cooperman, George Washington University“Looking to get started with data science, but scared it’d be too complicated? This book has you covered. Data Analysis for Social Science truly delivers what the title claims: friendly and practical. The focus is on experimental data and causal inference much more than on multiple regression analysis, reflecting recent developments in the social sciences. I don’t think I’ve seen a more accessible introduction to R and RStudio—cheat sheets included!”—Didier Ruedin, University of Neuchâtel“Following the step-by-step guidance provided in this book, I built my skills in R rather than another expensive proprietary software, allowing me to share my growing knowledge with my working-class, first-generation students. I am confident I can continue to independently develop these skills in ways that support both my teaching and research.”—Jamie D. Gravell, California State University, Stanislaus“At last, we have a truly modern introduction to social science statistics. The authors do not shy away from topics like causal inference, and they gently and seamlessly integrate instructions on how to use R. This textbook is a generous gift to both students and teachers.”—Valerio Baćak, School of Criminal Justice, Rutgers University, Newark“A very sensible and intuitive introduction to data science. Llaudet and Imai do an excellent job of explaining the why of data analysis along with the how. I would recommend this book to anyone looking for a nice primer on data science coupled with a good set of tools using the R software.”—Craig Depken, University of North Carolina, Charlotte