Many data engineering teams today face the "good pipelines, bad data" problem. It doesn't matter how advanced your data infrastructure is if the data you're piping is bad. In this book, Barr Moses, Lior Gavish, and Molly Vorwerck, from the data observability company Monte Carlo, explain how to tackle data quality and trust at scale by leveraging best practices and technologies used by some of the world's most innovative companies.
- Build more trustworthy and reliable data pipelines
- Write scripts to make data checks and identify broken pipelines with data observability
- Learn how to set and maintain data SLAs, SLIs, and SLOs
- Develop and lead data quality initiatives at your company
- Learn how to treat data services and systems with the diligence of production software
- Automate data lineage graphs across your data ecosystem
- Build anomaly detectors for your critical data assets
Many data engineering teams today face the "good pipelines, bad data" problem. It doesn't matter how advanced your data infrastructure is if the data you're piping is bad. In this book, Barr Moses, Lior Gavish, and Molly Vorwerck, from the data observability company Monte Carlo, explain how to tackle data quality and trust at scale by leveraging best practices and technologies used by some of the world's most innovative companies.
- Build more trustworthy and reliable data pipelines
- Write scripts to make data checks and identify broken pipelines with data observability
- Learn how to set and maintain data SLAs, SLIs, and SLOs
- Develop and lead data quality initiatives at your company
- Learn how to treat data services and systems with the diligence of production software
- Automate data lineage graphs across your data ecosystem
- Build anomaly detectors for your critical data assets
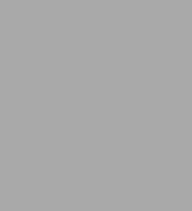
Data Quality Fundamentals: A Practitioner's Guide to Building Trustworthy Data Pipelines
308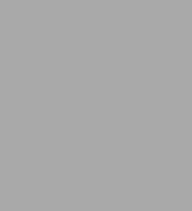
Data Quality Fundamentals: A Practitioner's Guide to Building Trustworthy Data Pipelines
308Product Details
ISBN-13: | 9781098112042 |
---|---|
Publisher: | O'Reilly Media, Incorporated |
Publication date: | 10/11/2022 |
Pages: | 308 |
Product dimensions: | 7.00(w) x 9.19(h) x (d) |