Deep Learning on Embedded Systems is a comprehensive guide to the practical implementation of deep learning for engineering tasks through computers and embedded hardware such as Raspberry Pi and Nvidia Jetson Nano. After an introduction to the field, the book provides fundamental knowledge on deep learning, convolutional and recurrent neural networks, computer vision, and basics of Linux terminal and docker engines. This book shows detailed setup steps of Jetson Nano and Raspberry Pi for utilizing essential frameworks such as PyTorch and OpenCV. GPU configuration and dependency installation procedure for using PyTorch is also discussed allowing newcomers to seamlessly navigate the learning curve.
A key challenge of utilizing deep learning on embedded systems is managing limited GPU and memory resources. This book outlines a strategy of training complex models on a desktop computer and transferring them to embedded systems for inference. Also, students and researchers often face difficulties with the varying probabilistic theories and notations found in data science literature. To simplify this, the book mainly focuses on the practical implementation part of deep learning using Python programming, low-cost hardware, and freely available software such as Anaconda and Visual Studio Code. To aid in reader learning, questions and answers are included at the end of most chapters.
Written by a highly qualified author, Deep Learning on Embedded Systems includes discussion on:
- Fundamentals of deep learning, including neurons and layers, activation functions, network architectures, hyperparameter tuning, and convolutional and recurrent neural networks (CNNs & RNNs)
- PyTorch, OpenCV, and other essential framework setups for deep transfer learning, along with Linux terminal operations, docker engine, docker images, and virtual environments in embedded devices
- Training models for image classification and object detection with classification, then converting trained PyTorch models to ONNX format for efficient deployment on Jetson Nano and Raspberry Pi
Deep Learning on Embedded Systems serves as an excellent introduction to the field for undergraduate engineering students seeking to learn deep learning implementations for their senior capstone or class projects and graduate researchers and educators who wish to implement deep learning in their research.
Deep Learning on Embedded Systems is a comprehensive guide to the practical implementation of deep learning for engineering tasks through computers and embedded hardware such as Raspberry Pi and Nvidia Jetson Nano. After an introduction to the field, the book provides fundamental knowledge on deep learning, convolutional and recurrent neural networks, computer vision, and basics of Linux terminal and docker engines. This book shows detailed setup steps of Jetson Nano and Raspberry Pi for utilizing essential frameworks such as PyTorch and OpenCV. GPU configuration and dependency installation procedure for using PyTorch is also discussed allowing newcomers to seamlessly navigate the learning curve.
A key challenge of utilizing deep learning on embedded systems is managing limited GPU and memory resources. This book outlines a strategy of training complex models on a desktop computer and transferring them to embedded systems for inference. Also, students and researchers often face difficulties with the varying probabilistic theories and notations found in data science literature. To simplify this, the book mainly focuses on the practical implementation part of deep learning using Python programming, low-cost hardware, and freely available software such as Anaconda and Visual Studio Code. To aid in reader learning, questions and answers are included at the end of most chapters.
Written by a highly qualified author, Deep Learning on Embedded Systems includes discussion on:
- Fundamentals of deep learning, including neurons and layers, activation functions, network architectures, hyperparameter tuning, and convolutional and recurrent neural networks (CNNs & RNNs)
- PyTorch, OpenCV, and other essential framework setups for deep transfer learning, along with Linux terminal operations, docker engine, docker images, and virtual environments in embedded devices
- Training models for image classification and object detection with classification, then converting trained PyTorch models to ONNX format for efficient deployment on Jetson Nano and Raspberry Pi
Deep Learning on Embedded Systems serves as an excellent introduction to the field for undergraduate engineering students seeking to learn deep learning implementations for their senior capstone or class projects and graduate researchers and educators who wish to implement deep learning in their research.
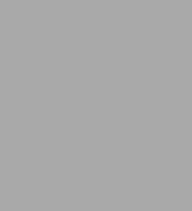
Deep Learning on Embedded Systems: A Hands-On Approach Using Jetson Nano and Raspberry Pi
256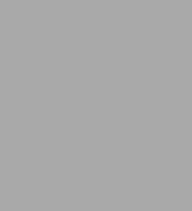