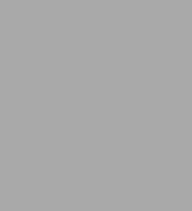
- ISBN-10:
- 0691010188
- ISBN-13:
- 9780691010182
- Pub. Date:
- 11/19/2000
- Publisher:
- Princeton University Press
- ISBN-10:
- 0691010188
- ISBN-13:
- 9780691010182
- Pub. Date:
- 11/19/2000
- Publisher:
- Princeton University Press
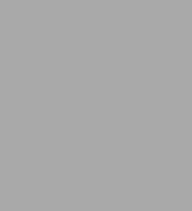
Buy New
$130.00Buy Used
$70.95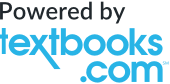
-
SHIP THIS ITEMIn stock. Ships in 1-2 days.PICK UP IN STORE
Your local store may have stock of this item.
Available within 2 business hours
$70.95-
SHIP THIS ITEM
Temporarily Out of Stock Online
Please check back later for updated availability.
Overview
Econometrics provides first-year graduate students with a thoroughly modern introduction to the subject, covering all the standard material necessary for understanding the principal techniques of econometrics, from ordinary least squares through cointegration. The book is distinctive in developing both time-series and cross-section analysis fully, giving readers a unified framework for understanding and integrating results.
Econometrics covers all the important topics in a succinct manner. All the estimation techniques that could possibly be taught in a first-year graduate course, except maximum likelihood, are treated as special cases of GMM (generalized methods of moments). Maximum likelihood estimators for a variety of models, such as probit and tobit, are collected in a separate chapter. This arrangement enables students to learn various estimation techniques in an efficient way. Virtually all the chapters include empirical applications drawn from labor economics, industrial organization, domestic and international finance, and macroeconomics. These empirical exercises provide students with hands-on experience applying the techniques covered. The exposition is rigorous yet accessible, requiring a working knowledge of very basic linear algebra and probability theory. All the results are stated as propositions so that students can see the points of the discussion and also the conditions under which those results hold. Most propositions are proved in the text.
For students who intend to write a thesis on applied topics, the empirical applications in Econometrics are an excellent way to learn how to conduct empirical research. For theoretically inclined students, the no-compromise treatment of basic techniques is an ideal preparation for more advanced theory courses.
Product Details
ISBN-13: | 9780691010182 |
---|---|
Publisher: | Princeton University Press |
Publication date: | 11/19/2000 |
Series: | Economics Series |
Edition description: | New Edition |
Pages: | 712 |
Product dimensions: | 7.00(w) x 10.00(h) x (d) |
About the Author
Read an Excerpt
COPYRIGHT NOTICE: Published by Princeton University Press and copyrighted, © 2000, by Princeton University Press. All rights reserved. No part of this book may be reproduced in any form by any electronic or mechanical means (including photocopying, recording, or information storage and retrieval) without permission in writing from the publisher, except for reading and browsing via the World Wide Web. Users are not permitted to mount this file on any network servers.
Preface
This book is designed to serve as the textbook for a first-year graduate course in econometrics. It has two distinguishing features. First, it covers a full range of techniques with the estimation method called the Generalized Method of Moments (GMM) as the organizing principle. I believe this unified approach is the most efficient way to cover the first-year materials in an accessible yet rigorous manner. Second, most chapters include a section examining in detail original applied articles from such diverse fields in economics as industrial organization, labor, finance, international, and macroeconomics. So the reader will know how to use the techniques covered in the chapter and under what conditions they are applicable.
Over the last several years, the lecture notes on which this book is based have been used at the University of Pennsylvania, Columbia University, Princeton University, the University of Tokyo, Boston College, Harvard University, and Ohio State University. Students seem to like the book a lot. My own experience from teaching out of the book is that students think the book is much better than the instructor.
Prerequisites
The reader of this book is assumed to have a working knowledge of the basics of calculus, probability theory, and linear algebra. An understanding of the following concepts is taken for granted: functions of several variables, partial derivatives, integrals, random variables, joint distributions, independence, unconditional and conditional expectations, variances and covariances of vector random variables, normal distributions, chi-square distributions, matrix multiplication, inverses of matrices, the rank of a matrix, determinants, and positive definite matrices. Any relevant concepts above this level will be introduced as the discussion progresses. Results on partitioned matrices and Kronecker products are collected in the appendix. Prior exposure to undergraduate econometrics is not required.
Organization of the Book
To understand how the book is organized, it is useful to distinguish between a model and an estimation procedure. The basic premise of econometrics is that economic data (such as postwar U.S. GDP) are random variables. A model is a family of probability distributions that could possibly have generated the economic data. An estimation procedure is a data-based protocol for choosing from the model a particular distribution that is likely to have generated the data. Most estimation procedures in econometrics are a specialization of the GMM estimation principle. For example, when GMMis applied to a model called the classical linear regression model, the resulting estimation procedure is Ordinary Least Squares (OLS), the most basic estimation procedure in econometrics. This viewpoint is the organizing principle in the first six chapters of the book, where most of the standard estimation procedures are presented.
The book could have presented GMM in the first chapter, but that would deprive the reader of the option to follow a series of topics specific to OLS without getting distracted by GMM. For this reason I chose to use the first two chapters to present the finite-sample and large-sample theory of OLS. GMM is presented in Chapter 3 as a generalization of OLS.
A major expositional innovation of the book is to treat multiple-equation estimation procedures -such as Seemingly Unrelated Regressions (SUR), Three-Stage Least Squares (3SLS), the Random-Effects method, covered in Chapter 4, and the Fixed-Effects method covered in Chapter 5as special cases of the single-equation GMM of Chapter 3. This makes it possible to derive the statistical properties of those advanced techniques merely by suitably specializing the results about single-equation GMM developed in Chapter 3. Chapter 6 completes the book's discussion of GMM by indicating how serial dependence in the error term can be incorporated in GMM.
For some models in econometrics, Maximum Likelihood (ML) is the more natural estimation principle than GMM. ML is covered in Chapters 7 and 8. To make clear the relationship between GMM and ML, the book's discussion of ML starts out in Chapter 7 with an estimation principle called Extremum Estimators, which includes both ML and GMMas special cases. Applications of ML to various models are covered in Chapter 8.
The book also includes an extensive treatment of time-series analysis. Basic time-series topics are covered in Section 2.2 and in the first half of Chapter 6. That is enough of a prerequisite for the important recent advances in nonstationary time-series analysis, which are covered in the last two chapters of the book.
Designing a Course Out of the Book
Several different courses can be designed based on the book.
- Assuming that the course meets twice for an hour and a half, eight weeks should be enough to cover core theory, which is Chapters 1- 4 and 6 (excluding those. sections on specific economic applications), Chapter 7 (with proofs and examples skipped), and Chapter 8.
- A twelve-week semester course can cover, in addition to the core theory, Chapter 5 and the economic applications included in Chapters 1-6.
- A short (say, six-week) course specializing in GMM estimation in cross-section and panel data would cover Chapters 1-5 (excluding Sections 2.10-2.12 but including Section 2.2). Chapters 7 and 8 (excluding Section 8.7) would add the ML component to the course.
- A short time-series course covering recent developments with economic applications would consist of Chapter 1 (excluding Section 1.7), Chapter 2, parts of Chapters 6 and 7 (Sections 6.1-6.5, 6.8, and 7.1), and Chapters 9 and 10. The applications sections in Chapters 2, 6, 9, and 10 can be dropped if the course focuses on just theory.
Review Questions and Analytical Exercises
The book includes a number of short questions for review at the end of each chapter, with lots of hints (and even answers). They can be used to check whether the reader actually understood the materials of the section. On the second reading, if not the first, readers should try to answer them before proceeding. Answers to selected review questions are available from the book's website.
There are several analytical exercises at the end of each chapter that ask the reader to prove results left unproved in the text or supplementary results that are useful for their own sake. Unless otherwise indicated, analytical exercises can be skipped without loss of continuity.
Empirical Exercises Each chapter usually has one big empirical exercise. It asks for a replication of the empirical results of the original article discussed in the applications section of the chapter and an estimation of the various extensions of the article's model using the estimation procedures presented in the chapter. The dataset for estimation, which usually is the same as the one used by the original article, can be downloaded from the book's website mentioned above.
To implement the estimation procedure on the dataset, readers should run a statistical package on a computer. There are many statistical packages that are widely used in econometrics. They include GAUSS, MATLAB, Eviews, LIMDEP, RATS, SAS, Stata, and TSP. GAUSS and MATLAB are different from the rest in that they are matrix-based languages rather than a collection of procedures. Consider, for example, carrying out OLS with GAUSS or MATLAB. After loading the dataset into the computer's workspace, it takes several lines reflecting the matrix operations of OLS to calculate the OLS estimate and associated statistics (such as R2). With the other packages, which are procedure-driven and sometimes called "canned packages," those several lines can be replaced by a one-line command invoking the OLS procedure. For example, TSP's OLS command is OLSQ.
There are advantages and disadvantages with canned packages. Obviously, it takes far fewer lines to accomplish the same thing, so one can spend less time on programming. On the other hand, procedures in a canned package, which accept data and spit out point estimates and associated statistics, are essentially a black box. Sometimes it is not clear from the documentation of the package how certain statistics are calculated. Although those canned packages mentioned above regularly incorporate new developments in econometrics, the estimation procedure desired may not be currently supported by the package, in which case it will be necessary to write one's own procedures in GAUSS or MATLAB. But it may be a blessing in disguise; actually writing down the underlying matrix operations provides you with an excellent chance to understand the estimation procedure.
With only a few exceptions, all the calculations needed for the empirical exercises of the book can be carried out with any of the canned packages mentioned above. My recommendation, therefore, is for economics Ph.D. students who are planning to write an applied thesis using state-of-the-art estimation procedures or a theoretical thesis proposing new ones, to use GAUSS or MATLAB. Otherwise, students should use any of the canned packages mentioned above.
Mathematical Notation
There is no single mathematical notation used by everyone in econometrics. The book's notation follows the most standard, if not universal, practice. Vectors are treated as column vectors and written in bold lowercase letters. Matrices are in bold uppercase letters. The transpose of the matrix A is denoted by A´. Scalar variables are (mostly lowercase) letters in italics.
Acknowledgments
I acknowledge with gratitude help from the following individuals and institutions. Mark Watson, Dale Jorgenson, Bo Honore, Serena Ng, Masao Ogaki, and Jushan Bai were kind and courageous enough to use early versions of the book as the textbook for their econometrics courses. Comments made by them and their students have been incorporated in this final version. Yuzo Honda read the manuscript and offered helpful suggestions. Naoto Kunitomo, Whitney Newey, Serena Ng, Pierre Perron, Jim Stock, Katsuto Tanaka, Mark Watson, Hal White, and Yoshihiro Yajima took time out to answer my questions and enquiries. Two graduate students at University of Tokyo, Mari Sakudo and Naoki Shimoi, read the entire manuscript to weed out typos. Their effort was underwritten by a grant-in-aid from the Zengin Foundation for Studies on Economics and Finance. Peter Dougherty, my editor at Princeton University Press, provided enthusiasm and just the right amount of pressure. Stephanie Hogue was a versatile enough LATEX expert to accommodate my formatting whims. Ellen Foos supervised production of the book. Last but not least, Jessica Helfand agreed, probably out of friendship, to do the cover design.
For more than five years all my free time went into writing this book. Now, having completed the book, I feel like someone who has just been released from prison. My research suffered, but hopefully the profession has not noticed.
Table of Contents
List of Figures xvii
Preface xix
1 Finite-Sample Properties of OLS 3
1.1 The Classical Linear Regression Model 3
The Linearity Assumption 4
Matrix Notation 6
The Strict Exogeneity Assumption 7
Implications of Strict Exogeneity 8
Strict Exogeneity in Time-Series Models 9
Other Assumptions of the Model 10
The Classical Regression Model for Random Samples 12
"Fixed" Regressors 13
1.2 The Algebra of Least Squares 15
OLS Minimizes the Sum of Squared Residuals 15
Normal Equations 16
Two Expressions for the OLS Estimator 18
More Concepts and Algebra 18
Influential Analysis (optional) 21
A Note on the Computation of OLS Estimates 23
1.3 Finite-Sample Properties of OLS 27
Finite-Sample Distribution of b 27
Finite-Sample Properties of s2 30
Estimate of Var(b | X) 31
1.4 Hypothesis Testing under Normality 33
Normally Distributed Error Terms 33
Testing Hypotheses about Individual Regression Coefficients 35
Decision Rule for the t-Test 37
Confidence Interval 38
p-Value 38
Linear Hypotheses 39
The F-Test 40
A More Convenient Expression for F 42
t versus F 43
An Example of a Test Statistic Whose Distribution Depends on X 45
1.5 Relation to Maximum Likelihood 47
The Maximum Likelihood Principle 47
Conditional versus Unconditional Likelihood 47
The Log Likelihood for the Regression Model 48
ML via Concentrated Likelihood 48
Cramer-Rao Bound for the Classical Regression Model 49
The F-Test as a Likelihood Ratio Test 52
Quasi-Maximum Likelihood 53
1.6 Generalized Least Squares (GLS) 54
Consequence of Relaxing Assumption 1.4 55
Efficient Estimation with Known V 55
A Special Case: Weighted Least Squares (WLS) 58
Limiting Nature of GLS 58
1.7 Application: Returns to Scale in Electricity Supply 60
The Electricity Supply Industry 60
The Data 60
Why Do We Need Econometrics? 61
The Cobb-Douglas Technology 62
How Do We Know Things Are Cobb-Douglas? 63
Are the OLS Assumptions Satisfied? 64
Restricted Least Squares 65
Testing the Homogeneity of the Cost Function 65
Detour: A Cautionary Note on R2 67
Testing Constant Returns to Scale 67
Importance of Plotting Residuals 68
Subsequent Developments 68
Problem Set 71
Answers to Selected Questions 84
2 Large-Sample Theory 88
2.1 Review of Limit Theorems for Sequences of Random Variables 88
Various Modes of Convergence 89
Three Useful Results 92
Viewing Estimators as Sequences of Random Variables 94
Laws of Large Numbers and Central Limit Theorems 95
2.2 Fundamental Concepts in Time-Series Analysis 97
Need for Ergodic Stationarity 97
Various Classes of Stochastic Processes 98
Different Formulation of Lack of Serial Dependence 106
The CLT for Ergodic Stationary Martingale Differences Sequences 106
2.3 Large-Sample Distribution of the OLS Estimator 109
The Model 109
Asymptotic Distribution of the OLS Estimator 113
s2 Is Consistent 115
2.4 Hypothesis Testing 117
Testing Linear Hypotheses 117
The Test Is Consistent 119
Asymptotic Power 120
Testing Nonlinear Hypotheses 121
2.5 Estimating E([not displayable]) Consistently 123
Using Residuals for the Errors 123
Data Matrix Representation of S 125
Finite-Sample Considerations 125
2.6 Implications of Conditional Homoskedasticity 126
Conditional versus Unconditional Homoskedasticity 126
Reduction to Finite-Sample Formulas 127
Large-Sample Distribution of t and F Statistics 128
Variations of Asymptotic Tests under Conditional Homoskedasticity 129
2.7 Testing Conditional Homoskedasticity 131
2.8 Estimation with Parameterized Conditional Heteroskedasticity (optional) 133
The Functional Form 133
WLS with Known [alpha] 134
Regression of e2i on zi Provides a Consistent Estimate of [alpha] 135
WLS with Estimated [alpha] 136
OLS versus WLS 137
2.9 Least Squares Projection 137
Optimally Predicting the Value of the Dependent Variable 138
Best Linear Predictor 139
OLS Consistently Estimates the Projection Coefficients 140
2.10 Testing for Serial Correlation 141
Box-Pierce and Ljung-Box 142
Sample Autocorrelations Calculated from Residuals 144
Testing with Predetermined, but Not Strictly Exogenous, Regressors 146
An Auxiliary Regression-Based Test 147
2.11 Application: Rational Expectations Econometrics 150
The Efficient Market Hypotheses 150
Testable Implications 152
Testing for Serial Correlation 153
Is the Nominal Interest Rate the Optimal Predictor? 156
Rt Is Not Strictly Exogenous 158
Subsequent Developments 159
2.12 Time Regressions 160
The Asymptotic Distribution of the OLS Estimates 161
Hypothesis Testing for Time Regressions 163
2.A Asymptotics with Fixed Regressors 164
2.B Proof of Proposition 2.10 165
Problem Set 168
Answers to Selected Questions 183
3 Single-Equation GMM 186
3.1 Endogeneity Bias: Working's Example 187
A Simultaneous Equations Model of Market Equilibrium 187
Endogeneity Bias 188
Observable Supply Shifters 189
3.2 More Examples 193
A Simple Macroeconometric Model 193
Errors-in-Variables 194
Production Function 196
3.3 The General Formulation 198
Regressors and Instruments 198
Identification 200
Order Condition for Identification 202
The Assumption for Asymptotic Normality 202
3.4 Generalized Method of Moments Defined 204
Method of Moments 205
Generalized Method of Moments 206
Sampling Error 207
3.5 Large-Sample Properties of GMM 208
Asymptotic Distribution of the GMM Estimator 209
Estimation of Error Variance 210
Hypothesis Testing 211
Estimation of S 212
Efficient GMM Estimator 212
Asymptotic Power 214
Small-Sample Properties 215
3.6 Testing Overidentifying Restrictions 217
Testing Subsets of Orthogonality Conditions 218
3.7 Hypothesis Testing by the Likelihood-Ratio Principle 222
The LR Statistic for the Regression Model 223
Variable Addition Test (optional) 224
3.8 Implications of Conditional Homoskedasticity 225
Efficient GMM Becomes 2SLS 226
J Becomes Sargan's Statistic 227
Small-Sample Properties of 2SLS 229
Alternative Derivations of 2SLS 229
When Regressors Are Predetermined 231
Testing a Subset of Orthogonality Conditions 232
Testing Conditional Homoskedasticity 234
Testing for Serial Correlation 234
3.9 Application: Returns from Schooling 236
The NLS-Y Data 236
The Semi-Log Wage Equation 237
Omitted Variable Bias 238
IQ as the Measure of Ability 239
Errors-in-Variables 239
2SLS to Correct for the Bias 242
Subsequent Developments 243
Problem Set 244
Answers to Selected Questions 254
4 Multiple-Equation GMM 258
4.1 The Multiple-Equation Model 259
Linearity 259
Stationarity and Ergodicity 260
Orthogonality Conditions 261
Identification 262
The Assumption for Asymptotic Normality 264
Connection to the "Complete" System of Simultaneous Equations 265
4.2 Multiple-Equation GMM Defined 265
4.3 Large-Sample Theory 268
4.4 Single-Equation versus Multiple-Equation Estimation 271
When Are They "Equivalent"? 272
Joint Estimation Can Be Hazardous 273
4.5 Special Cases of Multiple-Equation GMM: FIVE, 3SLS, and SUR 274
Conditional Homoskedasticity 274
Full-Information Instrumental Variables Efficient (FIVE) 275
Three-Stage Least Squares (3SLS) 276
Seemingly Unrelated Regressions (SUR) 279
SUR versus OLS 281
4.6 Common Coefficients 286
The Model with Common Coefficients 286
The GMM Estimator 287
Imposing Conditional Homoskedasticity 288
Pooled OLS 290
Beautifying the Formulas 292
The Restriction That Isn't 293
4.7 Application: Interrelated Factor Demands 296
The Translog Cost Function 296
Factor Shares 297
Substitution Elasticities 298
Properties of Cost Functions 299
Stochastic Specifications 300
The Nature of Restrictions 301
Multivariate Regression Subject to Cross-Equation Restrictions 302
Which Equation to Delete? 304
Results 305
Problem Set 308
Answers to Selected Questions 320
5 Panel Data 323
5.1 The Error-Components Model 324
Error Components 324
Group Means 327
A Reparameterization 327
5.2 The Fixed-Effects Estimator 330
The Formula 330
Large-Sample Properties 331
Digression: When [eta]i Is Spherical 333
Random Effects versus Fixed Effects 334
Relaxing Conditional Homoskedasticity 335
5.3 Unbalanced Panels (optional) 337
"Zeroing Out" Missing Observations 338
Zeroing Out versus Compression 339
No Selectivity Bias 340
5.4 Application: International Differences in Growth Rates 342
Derivation of the Estimation Equation 342
Appending the Error Term 343
Treatment of [alpha]i 344
Consistent Estimation of Speed of Convergence 345
Appendix 5.A: Distribution of Hausman Statistic 346
Problem Set 349
Answers to Selected Questions 363
6 Serial Correlation 365
6.1 Modeling Serial Correlation: Linear Processes 365
MA(q) 366
MA([infinity]) as a Mean Square Limit 366
Filters 369
Inverting Lag Polynomials 372
6.2 ARMA Processes 375
AR(1) and Its MA([infinity]) Representation 376
Autocovariances of AR(1) 378
AR(p) and Its MA([infinity]) Representation 378
ARMA(p,q) 380
ARMA(p) with Common Roots 382
Invertibility 383
Autocovariance-Generating Function and the Spectrum 383
6.3 Vector Processes 387
6.4 Estimating Autoregressions 392
Estimation of AR(1) 392
Estimation of AR(p) 393
Choice of Lag Length 394
Estimation of VARs 397
Estimation of ARMA(p,q) 398
6.5 Asymptotics for Sample Means of Serially Correlated Processes 400
LLN for Covariance-Stationary Processes 401
Two Central Limit Theorems 402
Multivariate Extension 404
6.6 Incorporating Serial Correlation in GMM 406
The Model and Asymptotic Results 406
Estimating S When Autocovariances Vanish after Finite Lags 407
Using Kernels to Estimate S 408
VARHAC 410
6.7 Estimation under Conditional Homoskedasticity (Optional) 413
Kernel-Based Estimation of S under Conditional Homoskedasticity 413
Data Matrix Representation of Estimated Long-Run Variance 414
Relation to GLS 415
6.8 Application: Forward Exchange Rates as Optimal Predictors 418
The Market Efficiency Hypothesis 419
Testing Whether the Unconditional Mean Is Zero 420
Regression Tests 423
Problem Set 428
Answers to Selected Questions 441
7 Extremum Estimators 445
7.1 Extremum Estimators 446
"Measurability" of [theta] 446
Two Classes of Extremum Estimators 447
Maximum Likelihood (ML) 448
Conditional Maximum Likelihood 450
Invariance of ML 452
Nonlinear Least Squares (NLS) 453
Linear and Nonlinear GMM 454
7.2 Consistency 456
Two Consistency Theorems for Extremum Estimators 456
Consistency of M-Estimators 458
Concavity after Reparameterization 461
Identification in NLS and ML 462
Consistency of GMM 467
7.3 Asymptotic Normality 469
Asymptotic Normality of M-Estimators 470
Consistent Asymptotic Variance Estimation 473
Asymptotic Normality of Conditional ML 474
Two Examples 476
Asymptotic Normality of GMM 478
GMM versus ML 481
Expressing the Sampling Error in a Common Format 483
7.4 Hypothesis Testing 487
The Null Hypothesis 487
The Working Assumptions 489
The Wald Statistic 489
The Lagrange Multiplier (LM) Statistic 491
The Likelihood Ratio (LR) Statistic 493
Summary of the Trinity 494
7.5 Numerical Optimization 497
Newton-Raphson 497
Gauss-Newton 498
Writing Newton-Raphson and Gauss-Newton in a Common Format 498
Equations Nonlinear in Parameters Only 499
Problem Set 501
Answers to Selected Questions 505
8 Examples of Maximum Likelihood 507
8.1 Qualitative Response (QR) Models 507
Score and Hessian for Observation t 508
Consistency 509
Asymptotic Normality 510
8.2 Truncated Regression Models 511
The Model 511
Truncated Distributions 512
The Likelihood Function 513
Reparameterizing the Likelihood Function 514
Verifying Consistency and Asymptotic Normality 515
Recovering Original Parameters 517
8.3 Censored Regression (Tobit) Models 518
Tobit Likelihood Function 518
Reparameterization 519
8.4 Multivariate Regressions 521
The Multivariate Regression Model Restated 522
The Likelihood Function 523
Maximizing the Likelihood Function 524
Consistency and Asymptotic Normality 525
8.5 FIML 526
The Multiple-Equation Model with Common Instruments Restated 526
The Complete System of Simultaneous Equations 529
Relationship between ([Gamma]0, [Beta]0) and [delta]0 530
The FIML Likelihood Function 531
The FIML Concentrated Likelihood Function 532
Testing Overidentifying Restrictions 533
Properties of the FIML Estimator 533
ML Estimation of the SUR Model 535
8.6 LIML 538
LIML Defined 538
Computation of LIML 540
LIML versus 2SLS 542
8.7 Serially Correlated Observations 543
Two Questions 543
Unconditional ML for Dependent Observations 545
ML Estimation of AR.1/ Processes 546
Conditional ML Estimation of AR(1) Processes 547
Conditional ML Estimation of AR(p) and VAR(p) Processes 549
Problem Set 551
9 Unit-Root Econometrics 557
9.1 Modeling Trends 557
Integrated Processes 558
Why Is It Important to Know if the Process Is I(1)? 560
Which Should Be Taken as the Null, I(0) or I(1)? 562
Other Approaches to Modeling Trends 563
9.2 Tools for Unit-Root Econometrics 563
Linear I(0) Processes 563
Approximating I(1) by a Random Walk 564
Relation to ARMA Models 566
The Wiener Process 567
A Useful Lemma 570
9.3 Dickey-Fuller Tests 573
The AR(1) Model 573
Deriving the Limiting Distribution under the I(1) Null 574
Incorporating the Intercept 577
Incorporating Time Trend 581
9.4 Augmented Dickey-Fuller Tests 585
The Augmented Autoregression 585
Limiting Distribution of the OLS Estimator 586
Deriving Test Statistics 590
Testing Hypotheses about [zeta] 591
What to Do When p Is Unknown? 592
A Suggestion for the Choice of pmax(T) 594
Including the Intercept in the Regression 595
Incorporating Time Trend 597
Summary of the DF and ADF Tests and Other Unit-Root Tests 599
9.5 Which Unit-Root Test to Use? 601
Local-to-Unity Asymptotics 602
Small-Sample Properties 602
9.6 Application: Purchasing Power Parity 603
The Embarrassing Resiliency of the Random Walk Model? 604
Problem Set 605
Answers to Selected Questions 619
10 Cointegration 623
10.1 Cointegrated Systems 624
Linear Vector I(0) and I(1) Processes 624
The Beveridge-Nelson Decomposition 627
Cointegration Defined 629
10.2 Alternative Representations of Cointegrated Systems 633
Phillips's Triangular Representation 633
VAR and Cointegration 636
The Vector Error-Correction Model (VECM) 638
Johansen's ML Procedure 640
10.3 Testing the Null of No Cointegration 643
Spurious Regressions 643
The Residual-Based Test for Cointegration 644
Testing the Null of Cointegration 649
10.4 Inference on Cointegrating Vectors 650
The SOLS Estimator 650
The Bivariate Example 652
Continuing with the Bivariate Example 653
Allowing for Serial Correlation 654
General Case 657
Other Estimators and Finite-Sample Properties 658
10.5 Application: the Demand for Money in the United States 659
The Data 660
(m - p, y, R) as a Cointegrated System 660
DOLS 662
Unstable Money Demand? 663
Problem Set 665
Appendix. Partitioned Matrices and Kronecker Products 670
Addition and Multiplication of Partitioned Matrices 671
Inverting Partitioned Matrices 672
What People are Saying About This
Econometrics covers both modern and classic topics without shifting gears. The coverage is quite advanced yet the presentation is simple. Hayashi brings students to the frontier of applied econometric practice through a careful and efficient discussion of modern economic theory. The empirical exercises are very useful. . . . The projects are carefully crafted and have been thoroughly debugged.
Students of econometrics and their teachers will find this book to be the best introduction to the subject at the graduate and advanced undergraduate level. Starting with least squares regression, Hayashi provides an elegant exposition of all the standard topics of econometrics, including a detailed discussion of stationary and non-stationary time series. The particular strength of the book is the excellent balance between econometric theory and its applications, using GMM as an organizing principle throughout. Each chapter includes a detailed empirical example taken from classic and current applications of econometrics.
Dale Jorgensen, Harvard University
Econometrics covers both modern and classic topics without shifting gears. The coverage is quite advanced yet the presentation is simple. Hayashi brings students to the frontier of applied econometric practice through a careful and efficient discussion of modern economic theory. The empirical exercises are very useful. . . . The projects are carefully crafted and have been thoroughly debugged.
Mark W. Watson, Princeton University
“Students of econometrics and their teachers will find this book to be the best introduction to the subject at the graduate and advanced undergraduate level. Starting with least squares regression, Hayashi provides an elegant exposition of all the standard topics of econometrics, including a detailed discussion of stationary and nonstationary time series. The particular strength of the book is the excellent balance between econometric theory and its applications, using GMM as an organizing principle throughout. Each chapter includes a detailed empirical example taken from classic and current applications of econometrics.”—Dale Jorgensen, Harvard University
“Econometrics will be a very useful book for intermediate and advanced graduate courses. It covers the topics with an easy-to-understand approach while at the same time offering a rigorous analysis. The computer programming tips and problems should also be useful to students. I highly recommend this book for an up-to-date coverage and thoughtful discussion of topics in the methodology and application of econometrics.”—Jerry A. Hausman, Massachusetts Institute of Technology
“Econometrics covers both modern and classic topics without shifting gears. The coverage is quite advanced yet the presentation is simple. Hayashi brings students to the frontier of applied econometric practice through a careful and efficient discussion of modern economic theory. The empirical exercises are very useful. The projects are carefully crafted and have been thoroughly debugged.”—Mark W. Watson, Princeton University
“Econometrics strikes a good balance between technical rigor and clear exposition. The use of empirical examples is well done throughout. I very much like the use of old ‘classic’ examples. It gives students a sense of history—and shows that great empirical econometrics is a matter of having important ideas and good data, not just fancy new methods. The style is just great, informal and engaging.”—James H. Stock, John F. Kennedy School of Government, Harvard University
Econometrics strikes a good balance between technical rigor and clear exposition. . . . The use of empirical examples is well done throughout. I very much like the use of old 'classic' examples. It gives students a sense of history--and shows that great empirical econometrics is a matter of having important ideas and good data,not just fancy new methods. . . . The style is just great,informal and engaging.
Econometrics strikes a good balance between technical rigor and clear exposition. . . . The use of empirical examples is well done throughout. I very much like the use of old 'classic' examples. It gives students a sense of historyand shows that great empirical econometrics is a matter of having important ideas and good data, not just fancy new methods. . . . The style is just great, informal and engaging.
James H. Stock, John F. Kennedy School of Government, Harvard University
Econometrics will be a very useful book for intermediate and advanced graduate courses. It covers the topics with an easy to understand approach while at the same time offering a rigorous analysis. The computer programming tips and problems should also be useful to students. I highly recommend this book for an up-to-date coverage and thoughtful discussion of topics in the methodology and application of econometrics.
Jerry A. Hausman, Massachusetts Institute of Technology
Recipe
"Students of econometrics and their teachers will find this book to be the best introduction to the subject at the graduate and advanced undergraduate level. Starting with least squares regression, Hayashi provides an elegant exposition of all the standard topics of econometrics, including a detailed discussion of stationary and non-stationary time series. The particular strength of the book is the excellent balance between econometric theory and its applications, using GMM as an organizing principle throughout. Each chapter includes a detailed empirical example taken from classic and current applications of econometrics."Dale Jorgensen, Harvard University
"Econometrics will be a very useful book for intermediate and advanced graduate courses. It covers the topics with an easy to understand approach while at the same time offering a rigorous analysis. The computer programming tips and problems should also be useful to students. I highly recommend this book for an up-to-date coverage and thoughtful discussion of topics in the methodology and application of econometrics."Jerry A. Hausman, Massachusetts Institute of Technology
"Econometrics covers both modern and classic topics without shifting gears. The coverage is quite advanced yet the presentation is simple. Hayashi brings students to the frontier of applied econometric practice through a careful and efficient discussion of modern economic theory. The empirical exercises are very useful. . . . The projects are carefully crafted and have been thoroughly debugged."Mark W. Watson, Princeton University
"Econometrics strikes a good balance between technical rigor and clearexposition. . . . The use of empirical examples is well done throughout. I very much like the use of old 'classic' examples. It gives students a sense of historyand shows that great empirical econometrics is a matter of having important ideas and good data, not just fancy new methods. . . . The style is just great, informal and engaging."James H. Stock, John F. Kennedy School of Government, Harvard University