Further, this book covers about techniques for quantiative assessment of soft classified fraction outputs from soft classification and supported by in-house developed tool called sub-pixel multi-spectral image classifier (SMIC). It is aimed at graduate, postgraduate, research scholars and working professionals of different branches such as Geoinformation sciences, Geography, Electrical, Electronics and Computer Sciences etc., working in the fields of earth observation and satellite image processing. Learning algorithms discussed in this book may also be useful in other related fields, for example, in medical imaging. Overall, this book aims to:
- exclusive focus on using large range of fuzzy classification algorithms for remote sensing images;
- discuss ANN, CNN, RNN, and hybrid learning classifiers application on remote sensing images;
- describe sub-pixel multi-spectral image classifier tool (SMIC) to support discussed fuzzy and learning algorithms;
- explain how to assess soft classified outputs as fraction images using fuzzy error matrix (FERM) and its advance versions with FERM tool, Entropy, Correlation Coefficient, Root Mean Square Error and Receiver Operating Characteristic (ROC) methods and;
- combines explanation of the algorithms with case studies and practical applications.
Further, this book covers about techniques for quantiative assessment of soft classified fraction outputs from soft classification and supported by in-house developed tool called sub-pixel multi-spectral image classifier (SMIC). It is aimed at graduate, postgraduate, research scholars and working professionals of different branches such as Geoinformation sciences, Geography, Electrical, Electronics and Computer Sciences etc., working in the fields of earth observation and satellite image processing. Learning algorithms discussed in this book may also be useful in other related fields, for example, in medical imaging. Overall, this book aims to:
- exclusive focus on using large range of fuzzy classification algorithms for remote sensing images;
- discuss ANN, CNN, RNN, and hybrid learning classifiers application on remote sensing images;
- describe sub-pixel multi-spectral image classifier tool (SMIC) to support discussed fuzzy and learning algorithms;
- explain how to assess soft classified outputs as fraction images using fuzzy error matrix (FERM) and its advance versions with FERM tool, Entropy, Correlation Coefficient, Root Mean Square Error and Receiver Operating Characteristic (ROC) methods and;
- combines explanation of the algorithms with case studies and practical applications.
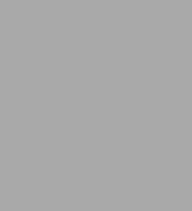
Fuzzy Machine Learning Algorithms for Remote Sensing Image Classification
220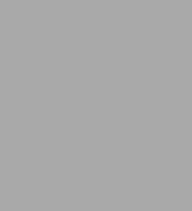