Metaheuristic algorithms can be considered the epitome of unsupervised learning algorithms for the optimization of engineering and artificial intelligence problems, including simulated annealing (SA), tabu search (TS), genetic algorithm (GA), ant colony optimization (ACO), particle swarm optimization (PSO), differential evolution (DE), and others. Distinct from most supervised learning algorithms that need labeled data to learn and construct determination models, metaheuristic algorithms inherit characteristics of unsupervised learning algorithms used for solving complex engineering optimization problems without labeled data, just like self-learning, to find solutions to complex problems.
Metaheuristic algorithms can be considered the epitome of unsupervised learning algorithms for the optimization of engineering and artificial intelligence problems, including simulated annealing (SA), tabu search (TS), genetic algorithm (GA), ant colony optimization (ACO), particle swarm optimization (PSO), differential evolution (DE), and others. Distinct from most supervised learning algorithms that need labeled data to learn and construct determination models, metaheuristic algorithms inherit characteristics of unsupervised learning algorithms used for solving complex engineering optimization problems without labeled data, just like self-learning, to find solutions to complex problems.
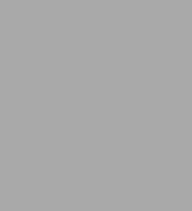
Handbook of Metaheuristic Algorithms: From Fundamental Theories to Advanced Applications
622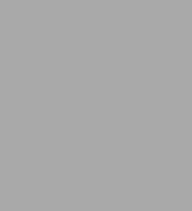
Handbook of Metaheuristic Algorithms: From Fundamental Theories to Advanced Applications
622Product Details
ISBN-13: | 9780443191084 |
---|---|
Publisher: | Elsevier Science |
Publication date: | 06/05/2023 |
Series: | Uncertainty, Computational Techniques, and Decision Intelligence |
Pages: | 622 |
Product dimensions: | 6.00(w) x 9.00(h) x 0.00(d) |