Hands-On Genetic Algorithms with Python - Second Edition: Apply genetic algorithms to solve real-world AI and machine learning problems
Explore the ever-growing world of genetic algorithms to build and enhance AI applications involving search, optimization, machine learning, deep learning, NLP, and XAI using Python libraries
1145910324
Key Features
- Learn how to implement genetic algorithms using Python libraries DEAP, scikit-learn, and NumPy
- Take advantage of cloud computing technology to increase the performance of your solutions
- Discover bio-inspired algorithms such as particle swarm optimization (PSO) and NEAT
- Purchase of the print or Kindle book includes a free PDF eBook
Book Description
Written by Eyal Wirsansky, a senior data scientist and AI researcher with over 25 years of experience and a research background in genetic algorithms and neural networks, Hands-On Genetic Algorithms with Python offers expert insights and practical knowledge to master genetic algorithms. After an introduction to genetic algorithms and their principles of operation, you’ll find out how they differ from traditional algorithms and the types of problems they can solve, followed by applying them to search and optimization tasks such as planning, scheduling, gaming, and analytics. As you progress, you’ll delve into explainable AI and apply genetic algorithms to AI to improve machine learning and deep learning models, as well as tackle reinforcement learning and NLP tasks. This updated second edition further expands on applying genetic algorithms to NLP and XAI and speeding up genetic algorithms with concurrency and cloud computing. You’ll also get to grips with the NEAT algorithm. The book concludes with an image reconstruction project and other related technologies for future applications. By the end of this book, you’ll have gained hands-on experience in applying genetic algorithms across a variety of fields, with emphasis on artificial intelligence with Python.What you will learn
- Use genetic algorithms to solve planning, scheduling, gaming, and analytics problems
- Create reinforcement learning, NLP, and explainable AI applications
- Enhance the performance of ML models and optimize deep learning architecture
- Deploy genetic algorithms using client-server architectures, enhancing scalability and computational efficiency
- Explore how images can be reconstructed using a set of semi-transparent shapes
- Delve into topics like elitism, niching, and multiplicity in genetic solutions to enhance optimization strategies and solution diversity
Who this book is for
If you’re a data scientist, software developer, AI enthusiast who wants to break into the world of genetic algorithms and apply them to real-world, intelligent applications as quickly as possible, this book is for you. Working knowledge of the Python programming language is required to get started with this book.
Hands-On Genetic Algorithms with Python - Second Edition: Apply genetic algorithms to solve real-world AI and machine learning problems
Explore the ever-growing world of genetic algorithms to build and enhance AI applications involving search, optimization, machine learning, deep learning, NLP, and XAI using Python libraries
Key Features
- Learn how to implement genetic algorithms using Python libraries DEAP, scikit-learn, and NumPy
- Take advantage of cloud computing technology to increase the performance of your solutions
- Discover bio-inspired algorithms such as particle swarm optimization (PSO) and NEAT
- Purchase of the print or Kindle book includes a free PDF eBook
Book Description
Written by Eyal Wirsansky, a senior data scientist and AI researcher with over 25 years of experience and a research background in genetic algorithms and neural networks, Hands-On Genetic Algorithms with Python offers expert insights and practical knowledge to master genetic algorithms. After an introduction to genetic algorithms and their principles of operation, you’ll find out how they differ from traditional algorithms and the types of problems they can solve, followed by applying them to search and optimization tasks such as planning, scheduling, gaming, and analytics. As you progress, you’ll delve into explainable AI and apply genetic algorithms to AI to improve machine learning and deep learning models, as well as tackle reinforcement learning and NLP tasks. This updated second edition further expands on applying genetic algorithms to NLP and XAI and speeding up genetic algorithms with concurrency and cloud computing. You’ll also get to grips with the NEAT algorithm. The book concludes with an image reconstruction project and other related technologies for future applications. By the end of this book, you’ll have gained hands-on experience in applying genetic algorithms across a variety of fields, with emphasis on artificial intelligence with Python.What you will learn
- Use genetic algorithms to solve planning, scheduling, gaming, and analytics problems
- Create reinforcement learning, NLP, and explainable AI applications
- Enhance the performance of ML models and optimize deep learning architecture
- Deploy genetic algorithms using client-server architectures, enhancing scalability and computational efficiency
- Explore how images can be reconstructed using a set of semi-transparent shapes
- Delve into topics like elitism, niching, and multiplicity in genetic solutions to enhance optimization strategies and solution diversity
Who this book is for
If you’re a data scientist, software developer, AI enthusiast who wants to break into the world of genetic algorithms and apply them to real-world, intelligent applications as quickly as possible, this book is for you. Working knowledge of the Python programming language is required to get started with this book.
39.99
In Stock
5
1
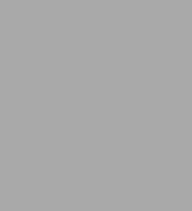
Hands-On Genetic Algorithms with Python - Second Edition: Apply genetic algorithms to solve real-world AI and machine learning problems
418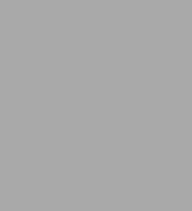
Hands-On Genetic Algorithms with Python - Second Edition: Apply genetic algorithms to solve real-world AI and machine learning problems
418Paperback(2nd ed.)
$39.99
39.99
In Stock
Product Details
ISBN-13: | 9781805123798 |
---|---|
Publisher: | Packt Publishing |
Publication date: | 07/12/2024 |
Edition description: | 2nd ed. |
Pages: | 418 |
Product dimensions: | 7.50(w) x 9.25(h) x 0.85(d) |
About the Author
From the B&N Reads Blog