Introduction to Machine Learning: From Math to Code
Emphasizing how and why machine learning algorithms work, this introductory textbook bridges the gap between the theoretical foundations of machine learning and its practical algorithmic and code-level implementation. Over 85 thorough worked examples, in both Matlab and Python, demonstrate how algorithms are implemented and applied whilst illustrating the end result. Over 75 end-of-chapter problems empower students to develop their own code to implement these algorithms, equipping them with hands-on experience. Matlab coding examples demonstrate how a mathematical idea is converted from equations to code, and provide a jumping off point for students, supported by in-depth coverage of essential mathematics including multivariable calculus, linear algebra, probability and statistics, numerical methods, and optimization. Accompanied online by instructor lecture slides, downloadable Python code and additional appendices, this is an excellent introduction to machine learning for senior undergraduate and graduate students in Engineering and Computer Science.
1147151890
Introduction to Machine Learning: From Math to Code
Emphasizing how and why machine learning algorithms work, this introductory textbook bridges the gap between the theoretical foundations of machine learning and its practical algorithmic and code-level implementation. Over 85 thorough worked examples, in both Matlab and Python, demonstrate how algorithms are implemented and applied whilst illustrating the end result. Over 75 end-of-chapter problems empower students to develop their own code to implement these algorithms, equipping them with hands-on experience. Matlab coding examples demonstrate how a mathematical idea is converted from equations to code, and provide a jumping off point for students, supported by in-depth coverage of essential mathematics including multivariable calculus, linear algebra, probability and statistics, numerical methods, and optimization. Accompanied online by instructor lecture slides, downloadable Python code and additional appendices, this is an excellent introduction to machine learning for senior undergraduate and graduate students in Engineering and Computer Science.
84.99
Pre Order
5
1
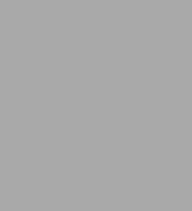
Introduction to Machine Learning: From Math to Code
668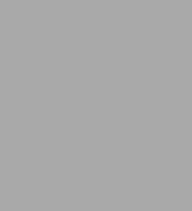
Introduction to Machine Learning: From Math to Code
668Hardcover
$84.99
84.99
Pre Order
Product Details
ISBN-13: | 9781316519509 |
---|---|
Publisher: | Cambridge University Press |
Publication date: | 09/30/2025 |
Pages: | 668 |
Product dimensions: | 0.00(w) x 10.00(h) x 0.00(d) |
About the Author
From the B&N Reads Blog