Learning in Graphical Models
In the past decade, a number of different research communities within the computational sciences have studied learning in networks, starting from a number of different points of view. There has been substantial progress in these different communities and surprising convergence has developed between the formalisms. The awareness of this convergence and the growing interest of researchers in understanding the essential unity of the subject underlies the current volume. Two research communities which have used graphical or network formalisms to particular advantage are the belief network community and the neural network community. Belief networks arose within computer science and statistics and were developed with an emphasis on prior knowledge and exact probabilistic calculations. Neural networks arose within electrical engineering, physics and neuroscience and have emphasised pattern recognition and systems modelling problems. This volume draws together researchers from these two communities and presents both kinds of networks as instances of a general unified graphical formalism. The book focuses on probabilistic methods for learning and inference in graphical models, algorithm analysis and design, theory and applications. Exact methods, sampling methods and variational methods are discussed in detail. Audience: A wide cross-section of computationally oriented researchers, including computer scientists, statisticians, electrical engineers, physicists and neuroscientists.
1100657883
Learning in Graphical Models
In the past decade, a number of different research communities within the computational sciences have studied learning in networks, starting from a number of different points of view. There has been substantial progress in these different communities and surprising convergence has developed between the formalisms. The awareness of this convergence and the growing interest of researchers in understanding the essential unity of the subject underlies the current volume. Two research communities which have used graphical or network formalisms to particular advantage are the belief network community and the neural network community. Belief networks arose within computer science and statistics and were developed with an emphasis on prior knowledge and exact probabilistic calculations. Neural networks arose within electrical engineering, physics and neuroscience and have emphasised pattern recognition and systems modelling problems. This volume draws together researchers from these two communities and presents both kinds of networks as instances of a general unified graphical formalism. The book focuses on probabilistic methods for learning and inference in graphical models, algorithm analysis and design, theory and applications. Exact methods, sampling methods and variational methods are discussed in detail. Audience: A wide cross-section of computationally oriented researchers, including computer scientists, statisticians, electrical engineers, physicists and neuroscientists.
329.99
In Stock
5
1
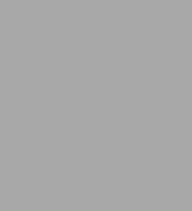
Learning in Graphical Models
630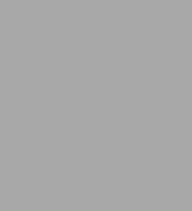
Learning in Graphical Models
630Paperback(Softcover reprint of the original 1st ed. 1998)
$329.99
329.99
In Stock
Product Details
ISBN-13: | 9789401061049 |
---|---|
Publisher: | Springer Netherlands |
Publication date: | 10/29/2012 |
Series: | NATO Science Series D: , #89 |
Edition description: | Softcover reprint of the original 1st ed. 1998 |
Pages: | 630 |
Product dimensions: | 6.30(w) x 9.45(h) x 0.05(d) |
About the Author
From the B&N Reads Blog