Linear Prediction Theory: A Mathematical Basis for Adaptive Systems
Lnear prediction theory and the related algorithms have matured to the point where they now form an integral part of many real-world adaptive systems. When it is necessary to extract information from a random process, we are frequently faced with the problem of analyzing and solving special systems of linear equations. In the general case these systems are overdetermined and may be characterized by additional properties, such as update and shift-invariance properties. Usually, one employs exact or approximate least-squares methods to solve the resulting class of linear equations. Mainly during the last decade, researchers in various fields have contributed techniques and nomenclature for this type of least-squares problem. This body of methods now constitutes what we call the theory of linear prediction. The immense interest that it has aroused clearly emerges from recent advances in processor technology, which provide the means to implement linear prediction algorithms, and to operate them in real time. The practical effect is the occurrence of a new class of high-performance adaptive systems for control, communications and system identification applications. This monograph presumes a background in discrete-time digital signal processing, including Z-transforms, and a basic knowledge of discrete-time random processes. One of the difficulties I have en- countered while writing this book is that many engineers and computer scientists lack knowledge of fundamental mathematics and geometry.
1112060105
Linear Prediction Theory: A Mathematical Basis for Adaptive Systems
Lnear prediction theory and the related algorithms have matured to the point where they now form an integral part of many real-world adaptive systems. When it is necessary to extract information from a random process, we are frequently faced with the problem of analyzing and solving special systems of linear equations. In the general case these systems are overdetermined and may be characterized by additional properties, such as update and shift-invariance properties. Usually, one employs exact or approximate least-squares methods to solve the resulting class of linear equations. Mainly during the last decade, researchers in various fields have contributed techniques and nomenclature for this type of least-squares problem. This body of methods now constitutes what we call the theory of linear prediction. The immense interest that it has aroused clearly emerges from recent advances in processor technology, which provide the means to implement linear prediction algorithms, and to operate them in real time. The practical effect is the occurrence of a new class of high-performance adaptive systems for control, communications and system identification applications. This monograph presumes a background in discrete-time digital signal processing, including Z-transforms, and a basic knowledge of discrete-time random processes. One of the difficulties I have en- countered while writing this book is that many engineers and computer scientists lack knowledge of fundamental mathematics and geometry.
54.99
In Stock
5
1
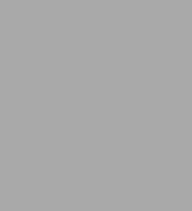
Linear Prediction Theory: A Mathematical Basis for Adaptive Systems
422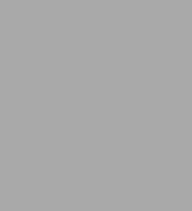
Linear Prediction Theory: A Mathematical Basis for Adaptive Systems
422Paperback(Softcover reprint of the original 1st ed. 1990)
$54.99
54.99
In Stock
Product Details
ISBN-13: | 9783642752087 |
---|---|
Publisher: | Springer Berlin Heidelberg |
Publication date: | 12/27/2011 |
Series: | Springer Series in Information Sciences , #21 |
Edition description: | Softcover reprint of the original 1st ed. 1990 |
Pages: | 422 |
Product dimensions: | 6.10(w) x 9.25(h) x 0.04(d) |
From the B&N Reads Blog