Ways to combat retraining depend on the algorithm and consist of the correct values of the trainer's met parameters. In practice, model estimation is not performed on the same input data that was used to train the model. Divide 10-20% of all available data into a separate set and call it a set for evaluation. We will bring the other 10-20% into the set for ratification, and 60-80% of the remaining ones will be given to the trainer. The principle of data sharing depends on the data and the task. Random sampling is often a good method if inputs are independent of each other, and there is no strong imbalance between the number of positive and negative entries.
The intuitive analogy here is the same as with university studies: the teacher solves some problems with students in pairs and gives other's similar tasks in the exam. What is important here (both in teaching students and models) is that these tasks are varied, and students cannot simply memorize the answers, and those who have mastered the material (similar tasks) will be able to repeat the thought process and answer correctly.
In machine learning, we split into data two sets: we will use the evaluation set to evaluate each model we train, using different approaches, algorithms, and model types to select the best one. That is, for each model, we will have two precision values - precision on the training dataset and precision on the evaluation dataset. It is normal for the former to be higher than the second, but not significantly. A big difference indicates retraining.
Ways to combat retraining depend on the algorithm and consist of the correct values of the trainer's met parameters. In practice, model estimation is not performed on the same input data that was used to train the model. Divide 10-20% of all available data into a separate set and call it a set for evaluation. We will bring the other 10-20% into the set for ratification, and 60-80% of the remaining ones will be given to the trainer. The principle of data sharing depends on the data and the task. Random sampling is often a good method if inputs are independent of each other, and there is no strong imbalance between the number of positive and negative entries.
The intuitive analogy here is the same as with university studies: the teacher solves some problems with students in pairs and gives other's similar tasks in the exam. What is important here (both in teaching students and models) is that these tasks are varied, and students cannot simply memorize the answers, and those who have mastered the material (similar tasks) will be able to repeat the thought process and answer correctly.
In machine learning, we split into data two sets: we will use the evaluation set to evaluate each model we train, using different approaches, algorithms, and model types to select the best one. That is, for each model, we will have two precision values - precision on the training dataset and precision on the evaluation dataset. It is normal for the former to be higher than the second, but not significantly. A big difference indicates retraining.
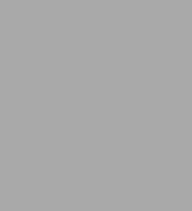
Machine Learning with Python: Advanced Methods and Strategies to Learn Machine Learning with Python
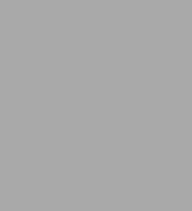
Machine Learning with Python: Advanced Methods and Strategies to Learn Machine Learning with Python
FREE
with a B&N Audiobooks Subscription
Product Details
BN ID: | 2940191939339 |
---|---|
Publisher: | MS Publishing LLC |
Publication date: | 07/04/2024 |
Edition description: | Unabridged |
Videos
