Model Predictive Control / Edition 2 available in Paperback
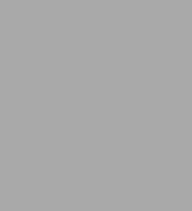
Model Predictive Control / Edition 2
- ISBN-10:
- 1852336943
- ISBN-13:
- 9781852336943
- Pub. Date:
- 05/13/2004
- Publisher:
- Springer London
- ISBN-10:
- 1852336943
- ISBN-13:
- 9781852336943
- Pub. Date:
- 05/13/2004
- Publisher:
- Springer London
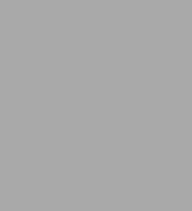
Model Predictive Control / Edition 2
Paperback
Buy New
$89.99-
SHIP THIS ITEMIn stock. Ships in 1-2 days.PICK UP IN STORE
Your local store may have stock of this item.
Available within 2 business hours
Overview
Product Details
ISBN-13: | 9781852336943 |
---|---|
Publisher: | Springer London |
Publication date: | 05/13/2004 |
Series: | Advanced Textbooks in Control and Signal Processing |
Edition description: | 2nd ed. 2004 |
Pages: | 405 |
Product dimensions: | 6.10(w) x 9.25(h) x 0.03(d) |
About the Author
Carlos Bordons received the Ph.D. degree in Electrical Engineering in 1994. He is currently full professor of Systems Engineering and Automatic Control at the University of Seville. His current research interests include advanced process control, especially model predictive control and its application to microgrids. He is co-author of the books Model Predictive Control in the Process Industry, Model Predictive Control (1st and 2nd edition) and Model Predictive Control of Microgrids, published by Springer. He is an Associate Editor of the journal Control Engineering Practice and was European Union Control Association Council Member from 2007 to 2015. From 2008 to 2012, he was the Managing Director of AICIA, which is the main Research and Technology Organization in Andalusia (Southern Spain). He is currently a member of the governing board of Corporación Tecnológica de Andalucía and head of the Laboratory of Engineering for Energy and Environmental Sustainability. He is a visiting professor at the University of Technology Sydney and Universidade Federal de Santa Catarina in Brazil.
José M. Maestre holds a PhD from the University of Seville, where he currently serves as a full professor. He has held positions at universities such as TU Delft, University of Pavia, Kyoto University and Tokyo Institute of Technology. He is author of Service Robotics within the Digital Home (Springer, 2011), A Programar se Aprende Jugando (Paraninfo, 2017), and Sistemas de Medida y Regulación (Paraninfo, 2018), and also editor of Distributed Model Predictive Control Made Easy (Springer, 2014) and Control Systems Benchmarks (Springer, 2025). His research focuses on the control of distributed cyber-physical systems, with a special emphasis on integrating heterogeneous agents into the control loop. He has published more than 200 journal and conference papers and has led multiple research projects. Finally, his achievements have been recognized with several awards and honors, including the Spanish Royal Academy of Engineering’s medal for his contributions to predictive control in large-scale systems.