Neuro-Fuzzy and Fuzzy-Neural Applications in Telecommunications
Neurofuzzy and fuzzyneural techniques as tools of studying and analyzing complex problems are relatively new even though neural networks and fuzzy logic systems have been applied as computational intelligence structural e- ments for the last 40 years. Computational intelligence as an independent sci- tific field has grown over the years because of the development of these str- tural elements. Neural networks have been revived since 1982 after the seminal work of J. J. Hopfield and fuzzy sets have found a variety of applications since the pub- cation of the work of Lotfi Zadeh back in 1965. Artificial neural networks (ANN) have a large number of highly interconnected processing elements that usually operate in parallel and are configured in regular architectures. The c- lective behavior of an ANN, like a human brain, demonstrates the ability to learn,recall,and generalize from training patterns or data. The performance of neural networks depends on the computational function of the neurons in the network,the structure and topology of the network,and the learning rule or the update rule of the connecting weights. This concept of trainable neural n- works further strengthens the idea of utilizing the learning ability of neural networks to learn the fuzzy control rules,the membership functions and other parameters of a fuzzy logic control or decision systems,as we will explain later on,and this becomes the advantage of using a neural based fuzzy logic system in our analysis. On the other hand,fuzzy systems are structured numerical estimators.
1101519476
Neuro-Fuzzy and Fuzzy-Neural Applications in Telecommunications
Neurofuzzy and fuzzyneural techniques as tools of studying and analyzing complex problems are relatively new even though neural networks and fuzzy logic systems have been applied as computational intelligence structural e- ments for the last 40 years. Computational intelligence as an independent sci- tific field has grown over the years because of the development of these str- tural elements. Neural networks have been revived since 1982 after the seminal work of J. J. Hopfield and fuzzy sets have found a variety of applications since the pub- cation of the work of Lotfi Zadeh back in 1965. Artificial neural networks (ANN) have a large number of highly interconnected processing elements that usually operate in parallel and are configured in regular architectures. The c- lective behavior of an ANN, like a human brain, demonstrates the ability to learn,recall,and generalize from training patterns or data. The performance of neural networks depends on the computational function of the neurons in the network,the structure and topology of the network,and the learning rule or the update rule of the connecting weights. This concept of trainable neural n- works further strengthens the idea of utilizing the learning ability of neural networks to learn the fuzzy control rules,the membership functions and other parameters of a fuzzy logic control or decision systems,as we will explain later on,and this becomes the advantage of using a neural based fuzzy logic system in our analysis. On the other hand,fuzzy systems are structured numerical estimators.
169.99
In Stock
5
1
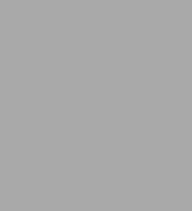
Neuro-Fuzzy and Fuzzy-Neural Applications in Telecommunications
339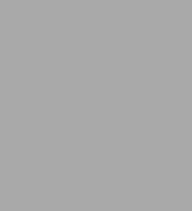
Neuro-Fuzzy and Fuzzy-Neural Applications in Telecommunications
339Hardcover(2004)
$169.99
169.99
In Stock
Product Details
ISBN-13: | 9783540407591 |
---|---|
Publisher: | Springer Berlin Heidelberg |
Publication date: | 05/14/2004 |
Series: | Signals and Communication Technology |
Edition description: | 2004 |
Pages: | 339 |
Product dimensions: | 6.10(w) x 9.25(h) x 0.03(d) |
From the B&N Reads Blog