Optimization Algorithms for Distributed Machine Learning
This book discusses state-of-the-art shastic optimization algorithms for distributed machine learning and analyzes their convergence speed. The book first introduces shastic gradient descent (SGD) and its distributed version, synchronous SGD, where the task of computing gradients is divided across several worker nodes. The author discusses several algorithms that improve the scalability and communication efficiency of synchronous SGD, such as asynchronous SGD, local-update SGD, quantized and sparsified SGD, and decentralized SGD. For each of these algorithms, the book analyzes its error versus iterations convergence, and the runtime spent per iteration. The author shows that each of these strategies to reduce communication or synchronization delays encounters a fundamental trade-off between error and runtime.
1142508497
Optimization Algorithms for Distributed Machine Learning
This book discusses state-of-the-art shastic optimization algorithms for distributed machine learning and analyzes their convergence speed. The book first introduces shastic gradient descent (SGD) and its distributed version, synchronous SGD, where the task of computing gradients is divided across several worker nodes. The author discusses several algorithms that improve the scalability and communication efficiency of synchronous SGD, such as asynchronous SGD, local-update SGD, quantized and sparsified SGD, and decentralized SGD. For each of these algorithms, the book analyzes its error versus iterations convergence, and the runtime spent per iteration. The author shows that each of these strategies to reduce communication or synchronization delays encounters a fundamental trade-off between error and runtime.
49.99
In Stock
5
1
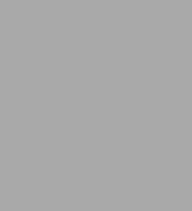
Optimization Algorithms for Distributed Machine Learning
127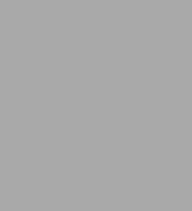
Optimization Algorithms for Distributed Machine Learning
127Paperback(1st ed. 2023)
$49.99
49.99
In Stock
Product Details
ISBN-13: | 9783031190698 |
---|---|
Publisher: | Springer International Publishing |
Publication date: | 11/27/2022 |
Series: | Synthesis Lectures on Learning, Networks, and Algorithms |
Edition description: | 1st ed. 2023 |
Pages: | 127 |
Product dimensions: | 6.61(w) x 9.45(h) x (d) |
About the Author
From the B&N Reads Blog